One-Dimensional Climate Models: The Basics of Brown Dwarfs¶
In this tutorial you will learn the very basics of running 1D climate runs. For a more in depth look at the climate code check out Mukherjee et al. 2022 (note this should also be cited if using this code/tutorial).
What you should already be familiar with:
What you will need to download to use this tutorial:
Download 1460 PT, 196 wno Correlated-K Tables from Roxana Lupu to be used by the climate code for opacity
Download the sonora bobcat cloud free
structures_
file so that you can validate your model run
Note: the two files above are dependent on metallicity and C/O. For this tutorial we will stick to solar M/H and solar C/O, but note that you can change that by picking the right C-K file in the opannection
step
[1]:
import warnings
warnings.filterwarnings('ignore')
import picaso.justdoit as jdi
import picaso.justplotit as jpi
jpi.output_notebook()
import astropy.units as u
import numpy as np
import matplotlib.pyplot as plt
%matplotlib inline
[2]:
#1 ck tables from roxana
mh = '+000' #log metallicity
CtoO = '100'# CtoO ratio relative to solar
ck_db = f'/data/kcoeff_2020_v3/sonora_2020_feh{mh}_co_{CtoO}.data.196'
#sonora bobcat cloud free structures file
sonora_profile_db = '/data/sonora_bobcat/structure/structures_m+0.0'
What does a climate model solve for?¶
1D Radiative-Convective Equilibrium Models solve for atmospheric structures of brown dwarfs and exoplanets, which includes:
The Temperature Structure (T(P) profile)
The Chemical Structure
Energy Transport in the atmosphere
But these physical components are not independent of each other. For example, the chemistry is dependent on the T(P) profile, the radiative transfer is dependent on clouds and the chemistry and so on.
PICASO
tries to find the atmospheric state of your object by taking care of all of these processes and their interconnections self-consistently and iteratively. Therefore, you will find that the climate portion of PICASO
is slower than running a single forward model evaluation.
Starting up the Run¶
You will notice that starting a run is nearly identical as running a spectrum. However, how we will add climate=True
to our inputs flag. We will also specify browndwarf
in this case, which will turn off the irradiation the object is receiving.
New Parameter: Effective Temperature. This excerpt from Modeling Exoplanetary Atmospheres (Fortney et al) provides a thorough description and more reading, if you are interested.
If the effective temperature, \(T_{eff}\), is defined as the temperature of a blackbody of the same radius that would emit the equivalent flux as the real planet, \(T_{eff}\) and \(T_{eq}\) can be simply related. This relation requires the inclusion of a third temperature, \(T_{int}\), the intrinsic effective temperature, that describes the flux from the planet’s interior. These temperatures are related by:”
\(T_{eff}^4 = T_{int}^4 + T_{eq}^4\)
We then recover our limiting cases: if a planet is self-luminous (like a young giant planet) and far from its parent star, \(T_{eff} \approx T_{int}\); for most rocky planets, or any planets under extreme stellar irradiation, \(T_{eff} \approx T_{eq}\).
[3]:
cl_run = jdi.inputs(calculation="browndwarf", climate = True) # start a calculation
#note you need to put the climate keyword to be True in order to do so
# now you need to add these parameters to your calculation
teff= 1000 # Effective Temperature of your Brown Dwarf in K
grav = 1000 # Gravity of your brown dwarf in m/s/s
cl_run.gravity(gravity=grav, gravity_unit=u.Unit('m/(s**2)')) # input gravity
cl_run.effective_temp(teff) # input effective temperature
Let’s now grab our gaseous opacities, whose path we have already defined above. Again, this code uses a correlated-k approach for accurately capturing opacities (see section 2.1.4; Mukerjee et al 2022).
[4]:
# Notice The keyword ck is set to True because you want to use the correlated-k opacities for your calculation
# and not the line by line opacities
opacity_ck = jdi.opannection(ck_db=ck_db) # grab your opacities
Initial T(P) Guess¶
Every calculation requires an initial guess of the pressure temperature profile. The code will iterate from there to find the correct solution. A few tips:
We recommend using typically 51-91 atmospheric pressure levels. Too many pressure layers increases the computational time required for convergence. Too little layers makes the atmospheric grid too coarse for an accurate calculation.
Start with a guess that is close to your expected solution. We will show an example using an isothermal P(T) profile below so you can see the iterative process. Later though, we recommend leveraging pre-computed grids (e.g. Sonora) as a starting guess for Brown Dwarfs.
[5]:
nlevel = 91 # number of plane-parallel levels in your code
#Lets set the max and min at 1e-4 bars and 500 bars
Pmin = 1e-4 #bars
Pmax = 500 #bars
pressure=np.logspace(np.log10(Pmin),np.log10(Pmax),nlevel) # set your pressure grid
temp_guess = np.zeros(shape=(nlevel)) + 500 # K , isothermal atmosphere guess
Initial Convective Zone Guess¶
You also need to have a crude guess of the convective zone of your atmosphere. Generally the deeper atmosphere is always convective. Again a good guess is always the published SONORA grid of models for this. But lets assume that the bottom 7 levels of the atmosphere is convective.
New Parameters:
nofczns
: Number of convective zones. Though the code has functionality to solve for more than one. In this basic tutorial, let’s stick to 1 for now.rfacv
: (See Mukherjee et al Eqn. 20r_st
) https://arxiv.org/pdf/2208.07836.pdfnstr_upper
: this defines the top most level of your guessed convective zone. If you don’t have a clue where your convective zone might end be choose a number that is \(\sim\)nlevel-5 (a few pressure levels away from the very bottom of your grid)
Non-zero values of rst (aka “rfacv” legacy terminology) is only relevant when the external irradiation on the atmosphere is non-zero. In the scenario when a user is computing a planet-wide average T(P) profile, the stellar irradiation is contributing to 50% (one hemisphere) of the planet and as a result rst = 0.5. If instead the goal is to compute a night-side average atmospheric state, rst is set to be 0. On the other extreme, to compute the day-side atmospheric state of a tidally locked planet rst should be set at 1.
[6]:
nofczns = 1 # number of convective zones initially. Let's not play with this for now.
nstr_upper = 83 # top most level of guessed convective zone
nstr_deep = nlevel -2 # this is always the case. Dont change this
nstr = np.array([0,nstr_upper,nstr_deep,0,0,0]) # initial guess of convective zones
# Here are some other parameters needed for the code.
rfacv = 0.0 #we are focused on a brown dwarf so let's keep this as is
Now we would use the inputs_climate function to input everything together to our cl_run we started.
[7]:
cl_run.inputs_climate(temp_guess= temp_guess, pressure= pressure,
nstr = nstr, nofczns = nofczns , rfacv = rfacv)
Run the Climate Code¶
The actual climate code can be run with the cl_run.run command. The save_all_profiles is set to True to save the T(P) profile at all steps. The code will now iterate from your guess to reach the correct atmospheric solution for your brown dwarf of interest.
[8]:
out = cl_run.climate(opacity_ck, save_all_profiles=True,with_spec=True)
Iteration number 0 , min , max temp 499.99441223740007 755.7256184267335 , flux balance -0.9375713000957998
Iteration number 1 , min , max temp 499.684629541375 2408.2450611089544 , flux balance 0.12082246704840309
Iteration number 2 , min , max temp 489.0489855596098 2400.4827908767315 , flux balance 0.12943466540276088
Iteration number 3 , min , max temp 478.7144203837924 2393.3279356287444 , flux balance 0.12147972759228186
Iteration number 4 , min , max temp 457.22135487015066 2379.115692181715 , flux balance 0.10781988494806535
Iteration number 5 , min , max temp 364.0917364355565 2323.581628774084 , flux balance 0.09583341293536846
Iteration number 6 , min , max temp 305.6636424896158 2314.511883907575 , flux balance 0.008816341975061157
Iteration number 7 , min , max temp 275.65114238837873 2313.8549187701637 , flux balance 0.0002846276663427909
Iteration number 8 , min , max temp 266.6542309263717 2313.814702059855 , flux balance 1.060434512602519e-05
We are already at a root, tolf , test = 5e-05 , 1.060434512602519e-05
Big iteration is 266.6542309263717 0
Iteration number 0 , min , max temp 267.4805886558728 2353.263324195818 , flux balance -0.8107827692691664
Iteration number 1 , min , max temp 277.0781216370256 2765.7119542549335 , flux balance -0.7092698041663692
Iteration number 2 , min , max temp 290.63182267809293 3035.0476392696132 , flux balance -0.5580046958917871
Iteration number 3 , min , max temp 344.5601059833999 3757.5067605696545 , flux balance 0.4130787669453553
Iteration number 4 , min , max temp 334.7488160906482 3573.3074886701215 , flux balance 0.05055089962015744
Iteration number 5 , min , max temp 333.5329910027303 3549.312440333875 , flux balance 0.0012940773383887767
Iteration number 6 , min , max temp 333.5015555175979 3548.91368724969 , flux balance 7.594977461031216e-06
In t_start: Converged Solution in iterations 6
Big iteration is 333.5015555175979 1
Iteration number 0 , min , max temp 333.6847625425446 3618.9398849662466 , flux balance -0.181285220349651
Iteration number 1 , min , max temp 355.05342993876326 5199.9 , flux balance 0.01922278426944214
Iteration number 2 , min , max temp 353.3192578152149 5199.9 , flux balance 0.0003152873071917125
Iteration number 3 , min , max temp 353.2795104584925 5199.9 , flux balance 1.6756955098714269e-06
Iteration number 4 , min , max temp 353.278951817682 5199.9 , flux balance 8.69952105305755e-09
In t_start: Converged Solution in iterations 4
Big iteration is 353.278951817682 2
Iteration number 0 , min , max temp 353.38425552615655 5199.9 , flux balance -0.02446863680275883
Iteration number 1 , min , max temp 357.8187283187612 5199.9 , flux balance 0.0006892507979793695
Iteration number 2 , min , max temp 357.7852138622747 5199.9 , flux balance 4.518773896925885e-06
Iteration number 3 , min , max temp 357.7852121869602 5199.9 , flux balance 4.504008245557094e-06
In t_start: Converged Solution in iterations 3
Big iteration is 357.7852121869602 3
Iteration number 0 , min , max temp 357.8003729029258 5199.9 , flux balance -0.006183689922693025
In t_start: Converged Solution in iterations 0
Profile converged before itmx
Iteration number 0 , min , max temp 357.8152677932942 5199.9 , flux balance -0.006100070665392575
In t_start: Converged Solution in iterations 0
Big iteration is 357.8152677932942 0
Iteration number 0 , min , max temp 357.8299029188794 5199.9 , flux balance -0.006017698709388589
In t_start: Converged Solution in iterations 0
Profile converged before itmx
convection zone status
0 83 89 0 0 0
1
[ 0 80 80 80 83 89]
Iteration number 0 , min , max temp 357.8454650213587 5199.9 , flux balance -0.0059264054272434846
Iteration number 1 , min , max temp 358.5340630073482 5199.9 , flux balance 6.701353965856e-05
Iteration number 2 , min , max temp 358.54142846061393 5199.9 , flux balance 2.3440364618448767e-07
In t_start: Converged Solution in iterations 2
Big iteration is 358.54142846061393 0
Iteration number 0 , min , max temp 358.54189377982397 5199.9 , flux balance -0.002219833404027642
In t_start: Converged Solution in iterations 0
Profile converged before itmx
Grow Phase : Upper Zone
[ 0 79 80 80 83 89]
Iteration number 0 , min , max temp 358.54472115685417 5199.9 , flux balance -0.002167452681643466
Iteration number 1 , min , max temp 358.65417213253176 5199.9 , flux balance 1.0016017088360219e-05
Iteration number 2 , min , max temp 358.65567702694693 5199.9 , flux balance 1.711958756863136e-08
Iteration number 3 , min , max temp 358.6556989456957 5199.9 , flux balance -9.33034498200691e-12
In t_start: Converged Solution in iterations 3
Big iteration is 358.6556989456957 0
Iteration number 0 , min , max temp 358.6558217280679 5199.9 , flux balance -0.0008635275885169664
In t_start: Converged Solution in iterations 0
Profile converged before itmx
[ 0 79 81 81 83 89]
Iteration number 0 , min , max temp 358.6567925129316 5199.9 , flux balance -0.0008336097111106223
In t_start: Converged Solution in iterations 0
Big iteration is 358.6567925129316 0
Iteration number 0 , min , max temp 358.6577397084271 5199.9 , flux balance -0.000816399861440016
In t_start: Converged Solution in iterations 0
Profile converged before itmx
[ 0 78 81 81 83 89]
Iteration number 0 , min , max temp 358.65874749919675 5199.9 , flux balance -0.0007966712951396764
In t_start: Converged Solution in iterations 0
Big iteration is 358.65874749919675 0
Iteration number 0 , min , max temp 358.6597471554962 5199.9 , flux balance -0.0007781113604892664
In t_start: Converged Solution in iterations 0
Profile converged before itmx
[ 0 77 81 81 83 89]
Iteration number 0 , min , max temp 358.6606365800866 5199.9 , flux balance -0.0007630185956735683
In t_start: Converged Solution in iterations 0
Big iteration is 358.6606365800866 0
Iteration number 0 , min , max temp 358.66153416892786 5199.9 , flux balance -0.000746050441041222
In t_start: Converged Solution in iterations 0
Profile converged before itmx
[ 0 76 81 81 83 89]
Iteration number 0 , min , max temp 358.6622462228763 5199.9 , flux balance -0.0007646395022767075
In t_start: Converged Solution in iterations 0
Big iteration is 358.6622462228763 0
Iteration number 0 , min , max temp 358.6629819663984 5199.9 , flux balance -0.0007490583166722165
In t_start: Converged Solution in iterations 0
Profile converged before itmx
[ 0 75 81 81 83 89]
Iteration number 0 , min , max temp 358.6654350916063 5199.9 , flux balance -0.0031392259853150004
In t_start: Converged Solution in iterations 0
Big iteration is 358.6654350916063 0
Iteration number 0 , min , max temp 358.66799822007897 5199.9 , flux balance -0.0030741484323680945
In t_start: Converged Solution in iterations 0
Profile converged before itmx
[ 0 75 82 82 83 89]
Iteration number 0 , min , max temp 358.6702438719396 3589.7748418560386 , flux balance -0.0030212220593243072
In t_start: Converged Solution in iterations 0
Big iteration is 358.6702438719396 0
Iteration number 0 , min , max temp 358.6726661030299 3666.566542187319 , flux balance -0.0029594970132165825
In t_start: Converged Solution in iterations 0
Big iteration is 358.6726661030299 1
Iteration number 0 , min , max temp 358.67526977593724 3743.048137651186 , flux balance -0.0028934017188332766
In t_start: Converged Solution in iterations 0
Big iteration is 358.67526977593724 2
Iteration number 0 , min , max temp 358.67806386346064 3819.171285991439 , flux balance -0.002822845996494602
In t_start: Converged Solution in iterations 0
Big iteration is 358.67806386346064 3
Iteration number 0 , min , max temp 358.6810515575669 3894.933976888795 , flux balance -0.002747734469609438
In t_start: Converged Solution in iterations 0
Big iteration is 358.6810515575669 4
Not converged
[ 0 75 89 0 83 89]
Iteration number 0 , min , max temp 358.7632496070537 3523.0553326263807 , flux balance 2.7827723948529942e-06
In t_start: Converged Solution in iterations 0
Big iteration is 358.7632496070537 0
Iteration number 0 , min , max temp 358.7940582859162 3526.04056402486 , flux balance -1.5944736298697232e-06
In t_start: Converged Solution in iterations 0
Profile converged before itmx
[ 0 74 89 0 83 89]
Iteration number 0 , min , max temp 358.9742024473379 3470.551489340211 , flux balance 3.0034714995645495e-05
Iteration number 1 , min , max temp 358.9765409379798 3470.44515761467 , flux balance 1.330522469655611e-07
In t_start: Converged Solution in iterations 1
Big iteration is 358.9765409379798 0
Iteration number 0 , min , max temp 359.0742956244379 3473.994066916569 , flux balance -5.638476076794328e-06
In t_start: Converged Solution in iterations 0
Profile converged before itmx
[ 0 73 89 0 83 89]
Iteration number 0 , min , max temp 359.20493416089477 3461.539958705847 , flux balance -1.3093034739490076e-05
In t_start: Converged Solution in iterations 0
Big iteration is 359.20493416089477 0
Iteration number 0 , min , max temp 359.28676471798815 3462.991568317384 , flux balance -8.670345461491372e-06
In t_start: Converged Solution in iterations 0
Profile converged before itmx
Grow Phase : Upper Zone
final [ 0 73 89 0 83 89]
Iteration number 0 , min , max temp 359.317877248601 3463.350020680684 , flux balance -2.5401864230600185e-06
In t_start: Converged Solution in iterations 0
Big iteration is 359.317877248601 0
Iteration number 0 , min , max temp 359.3272974208263 3463.4387908028148 , flux balance -6.679273597784512e-07
In t_start: Converged Solution in iterations 0
Profile converged before itmx
YAY ! ENDING WITH CONVERGENCE
xarray
model storage¶
[9]:
preserve_clima = jdi.output_xarray(out, cl_run)
[10]:
preserve_clima
[10]:
<xarray.Dataset> Size: 89kB Dimensions: (pressure: 91, pressure_layer: 90, wavelength: 196) Coordinates: * pressure (pressure) float64 728B 0.0001 0.0001187 ... 421.2 500.0 * wavelength (wavelength) float64 2kB 227.5 138.8 97.75 ... 0.287 0.2679 * pressure_layer (pressure_layer) float64 720B 0.0001089 0.0001293 ... 458.9 Data variables: (12/117) temperature (pressure) float64 728B 359.3 361.5 ... 3.35e+03 3.463e+03 dtdp (pressure_layer) float64 720B 0.03531 0.01945 ... 0.1947 guess 1 (pressure) float64 728B 500.0 500.0 500.0 ... 500.0 500.0 guess 2 (pressure) float64 728B 500.0 500.0 500.0 ... 679.1 714.5 guess 3 (pressure) float64 728B 500.0 500.0 500.0 ... 679.1 714.5 guess 4 (pressure) float64 728B 500.0 500.0 500.0 ... 718.3 755.7 ... ... OCS (pressure) float64 728B 1.013e-22 1.074e-22 ... 4.346e-10 Li (pressure) float64 728B 4.5e-38 4.5e-38 ... 2.194e-09 LiOH (pressure) float64 728B 4.5e-38 4.5e-38 ... 5.348e-11 LiH (pressure) float64 728B 4.5e-38 4.5e-38 ... 1.204e-09 LiCl (pressure) float64 728B 4.5e-38 4.5e-38 ... 4.816e-13 LiF (pressure) float64 728B 4.5e-38 4.5e-38 ... 2.427e-14 Attributes: climate_params: {'cvs_locs': array([ 0, 73, 89, 0, 83, 89]), 'converged... planet_params: {"effective_temp": 999.9689549563479, "gravity": {"value...
- pressure: 91
- pressure_layer: 90
- wavelength: 196
- pressure(pressure)float640.0001 0.0001187 ... 421.2 500.0
- units :
- bar
array([1.000000e-04, 1.186952e-04, 1.408854e-04, 1.672242e-04, 1.984870e-04, 2.355944e-04, 2.796392e-04, 3.319181e-04, 3.939708e-04, 4.676242e-04, 5.550473e-04, 6.588143e-04, 7.819806e-04, 9.281731e-04, 1.101697e-03, 1.307660e-03, 1.552130e-03, 1.842303e-03, 2.186724e-03, 2.595536e-03, 3.080775e-03, 3.656731e-03, 4.340362e-03, 5.151800e-03, 6.114937e-03, 7.258134e-03, 8.615054e-03, 1.022565e-02, 1.213735e-02, 1.440645e-02, 1.709976e-02, 2.029659e-02, 2.409107e-02, 2.859493e-02, 3.394079e-02, 4.028608e-02, 4.781762e-02, 5.675721e-02, 6.736805e-02, 7.996262e-02, 9.491175e-02, 1.126557e-01, 1.337168e-01, 1.587154e-01, 1.883875e-01, 2.236068e-01, 2.654104e-01, 3.150293e-01, 3.739246e-01, 4.438304e-01, 5.268051e-01, 6.252922e-01, 7.421915e-01, 8.809454e-01, 1.045640e+00, 1.241124e+00, 1.473154e+00, 1.748562e+00, 2.075458e+00, 2.463468e+00, 2.924018e+00, 3.470667e+00, 4.119514e+00, 4.889664e+00, 5.803794e+00, 6.888823e+00, 8.176699e+00, 9.705346e+00, 1.151978e+01, 1.367342e+01, 1.622968e+01, 1.926385e+01, 2.286525e+01, 2.713995e+01, 3.221380e+01, 3.823622e+01, 4.538455e+01, 5.386926e+01, 6.394020e+01, 7.589392e+01, 9.008241e+01, 1.069235e+02, 1.269130e+02, 1.506395e+02, 1.788018e+02, 2.122291e+02, 2.519057e+02, 2.989999e+02, 3.548984e+02, 4.212472e+02, 5.000000e+02])
- wavelength(wavelength)float64227.5 138.8 97.75 ... 0.287 0.2679
- units :
- micron
array([227.531286, 138.792505, 97.751711, 73.691968, 53.87931 , 42.211904, 35.752592, 29.70003 , 24.838549, 21.394951, 19.381723, 18.180165, 16.977929, 15.776603, 14.575135, 13.58788 , 12.786906, 11.986096, 11.29178 , 10.691757, 10.091327, 9.647853, 9.347542, 8.995233, 8.673027, 8.345156, 8.074283, 7.903889, 7.713966, 7.498219, 7.283852, 7.093708, 6.872616, 6.663779, 6.515295, 6.297428, 6.063914, 5.899009, 5.759373, 5.572117, 5.379091, 5.264682, 5.149065, 4.99975 , 4.881621, 4.82742 , 4.796393, 4.749804, 4.699911, 4.662331, 4.605006, 4.504099, 4.395218, 4.300705, 4.201857, 4.073652, 3.949369, 3.849337, 3.74932 , 3.662132, 3.587122, 3.513148, 3.43808 , 3.36338 , 3.307835, 3.269069, 3.230939, 3.193434, 3.155918, 3.118422, 3.084777, 3.054764, 3.014477, 2.964742, 2.908583, 2.846813, 2.787456, 2.730375, 2.675585, 2.622951, 2.573572, 2.527199, 2.481605, 2.436796, 2.394177, 2.353606, 2.314333, 2.276478, 2.240018, 2.208932, 2.182822, 2.162525, 2.147732, 2.136535, 2.128826, 2.113562, 2.091066, 2.069847, 2.049842, 2.029839, 2.009848, 1.982033, 1.94705 , 1.916269, 1.88935 , 1.863065, 1.837391, 1.812317, 1.788365, 1.765568, 1.740462, 1.713268, 1.691532, 1.674874, 1.659751, 1.646091, 1.627432, 1.604029, 1.583694, 1.567152, 1.552289, 1.537586, 1.522557, 1.506024, 1.488095, 1.470588, 1.452433, 1.433692, 1.415929, 1.40007 , 1.386482, 1.374098, 1.35829 , 1.339298, 1.318457, 1.295962, 1.276068, 1.25857 , 1.24106 , 1.22356 , 1.206051, 1.188555, 1.169211, 1.148221, 1.125613, 1.101619, 1.080246, 1.06125 , 1.039574, 1.015582, 0.996011, 0.980416, 0.962798, 0.943913, 0.931485, 0.918911, 0.903959, 0.891452, 0.877435, 0.865475, 0.852159, 0.834147, 0.822341, 0.80973 , 0.792428, 0.777427, 0.764968, 0.752426, 0.738951, 0.728775, 0.714255, 0.696874, 0.677124, 0.657415, 0.642413, 0.627973, 0.616515, 0.604407, 0.59094 , 0.578428, 0.565425, 0.551911, 0.536933, 0.523492, 0.506396, 0.484938, 0.464786, 0.444776, 0.42581 , 0.408323, 0.387097, 0.362069, 0.337037, 0.312 , 0.286957, 0.267868])
- pressure_layer(pressure_layer)float640.0001089 0.0001293 ... 386.7 458.9
- units :
- bars
array([1.089473e-04, 1.293152e-04, 1.534909e-04, 1.821862e-04, 2.162462e-04, 2.566738e-04, 3.046593e-04, 3.616159e-04, 4.292205e-04, 5.094640e-04, 6.047091e-04, 7.177604e-04, 8.519468e-04, 1.011220e-03, 1.200269e-03, 1.424661e-03, 1.691003e-03, 2.007139e-03, 2.382377e-03, 2.827766e-03, 3.356422e-03, 3.983910e-03, 4.728708e-03, 5.612747e-03, 6.662059e-03, 7.907542e-03, 9.385869e-03, 1.114057e-02, 1.322332e-02, 1.569544e-02, 1.862973e-02, 2.211258e-02, 2.624657e-02, 3.115340e-02, 3.697758e-02, 4.389060e-02, 5.209601e-02, 6.183545e-02, 7.339568e-02, 8.711712e-02, 1.034038e-01, 1.227353e-01, 1.456809e-01, 1.729161e-01, 2.052431e-01, 2.436136e-01, 2.891575e-01, 3.432160e-01, 4.073807e-01, 4.835412e-01, 5.739400e-01, 6.812390e-01, 8.085977e-01, 9.597663e-01, 1.139196e+00, 1.352171e+00, 1.604961e+00, 1.905011e+00, 2.261156e+00, 2.683883e+00, 3.185639e+00, 3.781199e+00, 4.488100e+00, 5.327157e+00, 6.323077e+00, 7.505187e+00, 8.908293e+00, 1.057371e+01, 1.255048e+01, 1.489682e+01, 1.768180e+01, 2.098744e+01, 2.491108e+01, 2.956824e+01, 3.509607e+01, 4.165734e+01, 4.944524e+01, 5.868911e+01, 6.966113e+01, 8.268439e+01, 9.814236e+01, 1.164902e+02, 1.382683e+02, 1.641177e+02, 1.947998e+02, 2.312179e+02, 2.744445e+02, 3.257523e+02, 3.866522e+02, 4.589375e+02])
- temperature(pressure)float64359.3 361.5 ... 3.35e+03 3.463e+03
- units :
- Kelvin
array([ 359.32729742, 361.50865944, 362.71573621, 364.09303267, 365.65224469, 367.40910842, 369.37720472, 371.5951964 , 374.07262666, 376.77719633, 379.68819624, 382.83510893, 386.22933858, 389.87552451, 393.79945575, 397.97374192, 402.48191934, 407.22974814, 412.09503458, 417.07357575, 422.16416497, 427.36196004, 432.65447075, 438.02283304, 443.44101065, 448.8890927 , 454.36179311, 459.881516 , 465.49485721, 471.25534552, 477.23203262, 483.48237618, 490.06059965, 497.08699825, 504.87907863, 512.97642531, 521.26909686, 529.88837245, 539.22450329, 549.36292366, 560.50965636, 572.96152418, 586.41383945, 600.57288659, 615.8096688 , 632.36128141, 650.26546916, 669.64708619, 690.38567238, 712.50550271, 736.2965899 , 761.76014839, 789.14837855, 818.44183221, 849.73959421, 883.14091323, 918.85384265, 956.94882907, 997.81322633, 1041.6156305 , 1088.94765687, 1139.34577851, 1190.70753389, 1241.64068997, 1290.80964022, 1339.45780069, 1387.5794589 , 1436.1100487 , 1484.11132074, 1532.22485903, 1581.76785198, 1634.57169836, 1693.43928577, 1765.56804124, 1846.96024826, 1931.10803537, 2018.06864575, 2107.80958048, 2200.12155184, 2295.10973601, 2392.42528092, 2491.91981097, 2593.70666321, 2697.13497462, 2802.21227124, 2909.07600151, 3017.19221998, 3126.5916161 , 3237.44938513, 3349.7541662 , 3463.4387908 ])
- dtdp(pressure_layer)float640.03531 0.01945 ... 0.199 0.1947
- units :
- K/bar
array([0.03531355, 0.01944961, 0.02211345, 0.0249335 , 0.0279671 , 0.03117128, 0.03493075, 0.03877095, 0.04203352, 0.04490597, 0.04815959, 0.05150271, 0.05482396, 0.05843026, 0.06152254, 0.06572296, 0.06842557, 0.06929569, 0.07006696, 0.07078434, 0.07139974, 0.07181402, 0.07195134, 0.07173036, 0.07124793, 0.07070455, 0.07045463, 0.07078759, 0.07176121, 0.0735332 , 0.07592145, 0.07885131, 0.08306285, 0.09075236, 0.0928356 , 0.09356834, 0.09568889, 0.10190681, 0.10868468, 0.11720276, 0.12820066, 0.13540704, 0.13920587, 0.14618223, 0.1547535 , 0.16290384, 0.17136607, 0.17795583, 0.18401049, 0.19164304, 0.19837249, 0.20609702, 0.21266307, 0.2189625 , 0.224956 , 0.23130105, 0.23702228, 0.24398507, 0.2506715 , 0.2592871 , 0.26397623, 0.25727237, 0.24439207, 0.22659659, 0.21585635, 0.20594127, 0.20058118, 0.19183369, 0.18615434, 0.18567357, 0.19159822, 0.20643606, 0.24337093, 0.26296252, 0.25995221, 0.25700111, 0.25385836, 0.25009473, 0.24662153, 0.24229747, 0.23773971, 0.23358987, 0.22814911, 0.22299719, 0.21837138, 0.21291513, 0.20781347, 0.20329462, 0.1989702 , 0.19473326])
- guess 1(pressure)float64500.0 500.0 500.0 ... 500.0 500.0
- units :
- Kelvin
array([500., 500., 500., 500., 500., 500., 500., 500., 500., 500., 500., 500., 500., 500., 500., 500., 500., 500., 500., 500., 500., 500., 500., 500., 500., 500., 500., 500., 500., 500., 500., 500., 500., 500., 500., 500., 500., 500., 500., 500., 500., 500., 500., 500., 500., 500., 500., 500., 500., 500., 500., 500., 500., 500., 500., 500., 500., 500., 500., 500., 500., 500., 500., 500., 500., 500., 500., 500., 500., 500., 500., 500., 500., 500., 500., 500., 500., 500., 500., 500., 500., 500., 500., 500., 500., 500., 500., 500., 500., 500., 500.])
- guess 2(pressure)float64500.0 500.0 500.0 ... 679.1 714.5
- units :
- Kelvin
array([500. , 500. , 500. , 500. , 500. , 500. , 500. , 500. , 500. , 500. , 500. , 500. , 500. , 500. , 500. , 500. , 500. , 500. , 500. , 500. , 500. , 500. , 500. , 500. , 500. , 500. , 500. , 500. , 500. , 500. , 500. , 500. , 500. , 500. , 500. , 500. , 500. , 500. , 500. , 500. , 500. , 500. , 500. , 500. , 500. , 500. , 500. , 500. , 500. , 500. , 500. , 500. , 500. , 500. , 500. , 500. , 500. , 500. , 500. , 500. , 500. , 500. , 500. , 500. , 500. , 500. , 500. , 500. , 500. , 500. , 500. , 500. , 500. , 500. , 500. , 500. , 500. , 500. , 500. , 500. , 500. , 500. , 500. , 500. , 526.21618047, 553.79142688, 582.79529984, 613.29508474, 645.36407929, 679.07339522, 714.49416807])
- guess 3(pressure)float64500.0 500.0 500.0 ... 679.1 714.5
- units :
- Kelvin
array([500. , 500. , 500. , 500. , 500. , 500. , 500. , 500. , 500. , 500. , 500. , 500. , 500. , 500. , 500. , 500. , 500. , 500. , 500. , 500. , 500. , 500. , 500. , 500. , 500. , 500. , 500. , 500. , 500. , 500. , 500. , 500. , 500. , 500. , 500. , 500. , 500. , 500. , 500. , 500. , 500. , 500. , 500. , 500. , 500. , 500. , 500. , 500. , 500. , 500. , 500. , 500. , 500. , 500. , 500. , 500. , 500. , 500. , 500. , 500. , 500. , 500. , 500. , 500. , 500. , 500. , 500. , 500. , 500. , 500. , 500. , 500. , 500. , 500. , 500. , 500. , 500. , 500. , 500. , 500. , 500. , 500. , 500. , 500. , 526.21618047, 553.79142688, 582.79529984, 613.29508474, 645.36407929, 679.07339522, 714.49416807])
- guess 4(pressure)float64500.0 500.0 500.0 ... 718.3 755.7
- units :
- Kelvin
array([499.99441224, 499.9944854 , 499.99452587, 499.99457343, 499.99462802, 499.99469027, 499.99476061, 499.99483898, 499.99492568, 499.99502096, 499.9951245 , 499.99523578, 499.99535405, 499.99547845, 499.99560744, 499.99574103, 499.99587948, 499.99602276, 499.99617133, 499.99632623, 499.99648947, 499.99666488, 499.99685695, 499.99706953, 499.99730562, 499.9975672 , 499.99785494, 499.99817133, 499.99851901, 499.99889787, 499.99930962, 499.99975994, 500.00025749, 500.00081591, 500.00145829, 500.00219113, 500.00302081, 500.00396478, 500.00504394, 500.00628461, 500.00772276, 500.00940506, 500.01133099, 500.01354212, 500.01610298, 500.01909273, 500.02260858, 500.02677615, 500.0316478 , 500.03736037, 500.04412082, 500.05218576, 500.0618687 , 500.07354968, 500.08769328, 500.1046211 , 500.12484475, 500.14902688, 500.17791653, 500.21235435, 500.25329168, 500.30160107, 500.35809249, 500.42434537, 500.50243365, 500.59515474, 500.70626753, 500.84079306, 501.00484029, 501.20559118, 501.45360617, 501.76247753, 502.14980306, 502.63834782, 503.25848132, 504.05743961, 505.09182541, 506.43203401, 508.16869339, 510.41959335, 513.33856563, 517.12767043, 522.21086459, 529.05230039, 556.77451924, 585.93289272, 616.59416855, 648.83282105, 682.71836212, 718.32404886, 755.72561843])
- guess 5(pressure)float64499.7 499.7 ... 2.309e+03 2.408e+03
- units :
- Kelvin
array([ 499.68462954, 499.68875767, 499.69104208, 499.69372589, 499.69680679, 499.70031984, 499.70428965, 499.70871245, 499.71360584, 499.718983 , 499.72482603, 499.73110621, 499.73778085, 499.74480144, 499.75208031, 499.75961957, 499.76743291, 499.77551855, 499.78390247, 499.79264418, 499.80185599, 499.81175433, 499.82259295, 499.83458879, 499.84791138, 499.86267176, 499.87890882, 499.89676204, 499.91638087, 499.93775823, 499.96099199, 499.98640134, 500.01447513, 500.04598354, 500.0822287 , 500.12357793, 500.17039056, 500.22365051, 500.2845373 , 500.35453552, 500.43567376, 500.53058483, 500.6392392 , 500.7639793 , 500.90844393, 501.07709704, 501.27541865, 501.51048861, 501.78525531, 502.10742527, 502.4886586 , 502.94340409, 503.48931011, 504.14775533, 504.9448592 , 505.89864407, 507.03779356, 508.39942153, 510.0253941 , 511.96257453, 514.2638331 , 516.97733188, 520.14740097, 523.86108767, 528.23243035, 533.41485611, 539.61376912, 547.10216441, 556.20922593, 567.31720878, 580.9847181 , 597.92000847, 619.02281435, 645.42875381, 678.60912247, 720.80736434, 774.53214372, 842.64549114, 928.4585609 , 1035.70170844, 1168.36829299, 1330.38765663, 1531.10099971, 1774.05349937, 1855.87606732, 1940.57365388, 2028.22008048, 2118.82188904, 2212.3439425 , 2308.8786336 , 2408.24506111])
- guess 6(pressure)float64489.0 489.1 ... 2.301e+03 2.4e+03
- units :
- Kelvin
array([ 489.04898556, 489.061507 , 489.06595941, 489.07266957, 489.0809023 , 489.0909479 , 489.10298626, 489.11577414, 489.12983772, 489.14625762, 489.1648591 , 489.18549358, 489.20778554, 489.23133836, 489.25399161, 489.27640961, 489.30008509, 489.3251848 , 489.35213112, 489.38159148, 489.41448977, 489.45329282, 489.50116511, 489.56029415, 489.63182003, 489.71590791, 489.81171982, 489.92016903, 490.04157198, 490.17416421, 490.31851002, 490.47830545, 490.65906584, 490.86952924, 491.12583691, 491.4271712 , 491.77042446, 492.16207508, 492.61097105, 493.12968818, 493.73721546, 494.46247141, 495.30133859, 496.26577464, 497.3828643 , 498.68540322, 500.21341148, 502.02126986, 504.12751599, 506.58587827, 509.48172393, 512.91946436, 517.02274088, 521.93554696, 527.82620988, 534.78778103, 542.97238957, 552.56566278, 563.74837679, 576.68523238, 591.5170906 , 608.28353235, 626.93486281, 647.58721287, 670.38059638, 695.48661137, 723.0822457 , 753.3044512 , 786.10004235, 821.16367128, 858.19939524, 896.71667585, 936.10084256, 975.73878361, 1015.18286025, 1054.48146864, 1093.48689432, 1132.03424117, 1171.05432109, 1216.314516 , 1282.60647529, 1388.93461905, 1550.56573766, 1767.84843814, 1849.44604347, 1933.92001599, 2021.33747088, 2111.71987039, 2205.01753076, 2301.32261353, 2400.48279088])
- guess 7(pressure)float64478.7 478.7 ... 2.294e+03 2.393e+03
- units :
- Kelvin
array([ 478.71442038, 478.73420853, 478.74024585, 478.75031437, 478.76296807, 478.77874697, 478.79799864, 478.81825883, 478.84058966, 478.8671532 , 478.8976446 , 478.93181856, 478.96899991, 479.00846799, 479.04609417, 479.08321076, 479.12273318, 479.16498259, 479.21074416, 479.26124083, 479.31825162, 479.386426 , 479.4715678 , 479.57761257, 479.70653131, 479.85845849, 480.03165363, 480.22783773, 480.44747926, 480.68695221, 480.94710645, 481.23470839, 481.55986745, 481.93864782, 482.40092543, 482.94420471, 483.56153845, 484.26395648, 485.06674476, 485.99185708, 487.07274298, 488.36116611, 489.84689556, 491.54737148, 493.5068584 , 495.77799453, 498.42364658, 501.52930909, 505.1137749 , 509.25103479, 514.06184443, 519.6873321 , 526.28360328, 534.01665422, 543.06038392, 553.44142212, 565.24387473, 578.5626797 , 593.4506072 , 609.91920278, 627.95721798, 647.48194367, 668.39627767, 690.85826428, 715.09593795, 741.38606846, 770.00580762, 801.18244108, 834.9522781 , 871.09919569, 909.41002204, 949.47477938, 990.73967509, 1032.60948697, 1074.59107742, 1116.5890799 , 1158.32784257, 1199.50456849, 1240.49809868, 1284.70700938, 1341.96671174, 1428.95496464, 1566.49761912, 1762.13045243, 1843.52056513, 1927.78825686, 2014.99448343, 2105.17441375, 2198.26500347, 2294.358201 , 2393.32793563])
- guess 8(pressure)float64457.2 457.3 ... 2.281e+03 2.379e+03
- units :
- Kelvin
array([ 457.22135487, 457.25673748, 457.2663207 , 457.28376784, 457.30616267, 457.33460129, 457.3698132 , 457.40679484, 457.44781039, 457.49735636, 457.55489227, 457.62000854, 457.69143114, 457.76778496, 457.84093586, 457.91366236, 457.99178849, 458.07594039, 458.16762982, 458.26919287, 458.38441218, 458.52258291, 458.69470666, 458.90811022, 459.16637169, 459.46949511, 459.81384716, 460.2030991 , 460.63819244, 461.11139776, 461.62392351, 462.18869538, 462.82507486, 463.56393048, 464.46288969, 465.51535023, 466.70603882, 468.054691 , 469.58894618, 471.34870834, 473.39507119, 475.8235525 , 478.60808415, 481.77333347, 485.3927977 , 489.55157216, 494.34797705, 499.9156984 , 506.25837296, 513.46861217, 521.70816833, 531.15342371, 541.97863765, 554.34035324, 568.36835135, 583.93488564, 600.98410717, 619.46314805, 639.26278773, 660.24704537, 682.30391241, 705.31154817, 729.20854849, 754.24859814, 780.75980131, 809.10187096, 839.61251028, 872.56745654, 908.05641811, 945.92699524, 986.03737599, 1028.05512545, 1071.48860229, 1115.76253133, 1160.34247639, 1204.99237369, 1249.37780953, 1293.18837762, 1336.43806842, 1380.36072059, 1429.24590146, 1493.57686523, 1594.96734965, 1750.77670261, 1831.7542746 , 1915.61174364, 2002.3978937 , 2092.17498874, 2184.85358156, 2280.52519733, 2379.11569218])
- guess 9(pressure)float64364.1 364.2 ... 2.227e+03 2.324e+03
- units :
- Kelvin
array([ 364.09173644, 364.2095572 , 364.24251589, 364.30234031, 364.37975844, 364.4786967 , 364.60193099, 364.73341463, 364.88150852, 365.06161681, 365.27244848, 365.5130877 , 365.77977924, 366.06830856, 366.3537868 , 366.64578166, 366.96221478, 367.30481044, 367.67793953, 368.0884087 , 368.55089405, 369.09605554, 369.75497439, 370.54767431, 371.48384125, 372.5625533 , 373.77204275, 375.12720464, 376.63276281, 378.26114358, 380.01295286, 381.92581516, 384.05660476, 386.49668414, 389.41673068, 392.78699585, 396.55393631, 400.768939 , 405.5046982 , 410.86599303, 417.01333691, 424.19914532, 432.30345431, 441.35309807, 451.50148749, 462.91324964, 475.7603499 , 490.27957631, 506.32375359, 523.94490233, 543.32377052, 564.6118802 , 587.88294245, 613.10127091, 640.12147129, 668.32345662, 697.30855941, 726.78235198, 756.48562627, 786.26222186, 816.11328083, 846.10869386, 876.40034424, 907.43931501, 939.66606756, 973.46344608, 1009.12290091, 1046.84251917, 1086.64663186, 1128.36503152, 1171.91060509, 1217.07106313, 1263.51172694, 1310.80737532, 1358.53130685, 1406.41924013, 1454.34434044, 1502.33042494, 1550.29584989, 1597.02748371, 1638.62970066, 1668.28117213, 1684.82372133, 1706.58104349, 1785.94584811, 1868.19844036, 1953.34097153, 2041.45068909, 2132.51200364, 2226.52864173, 2323.58162877])
- guess 10(pressure)float64305.7 306.1 ... 2.218e+03 2.315e+03
- units :
- Kelvin
array([ 305.66364249, 306.09740352, 306.2882101 , 306.54687822, 306.85917047, 307.23366834, 307.67650711, 308.16333425, 308.71033148, 309.34237272, 310.0563247 , 310.84870854, 311.71078231, 312.63262587, 313.57504139, 314.55368033, 315.59146156, 316.68802833, 317.84757127, 319.0783111 , 320.41015083, 321.89384844, 323.56817437, 325.45692625, 327.5721518 , 329.9116914 , 332.45849397, 335.24261547, 338.27516701, 341.50579849, 344.92254302, 348.56601676, 352.50156816, 356.83814053, 361.77910703, 367.25283943, 373.17318788, 379.58286953, 386.54403567, 394.1475827 , 402.53744192, 411.94329567, 422.13061544, 433.08036228, 444.9055634 , 457.71867664, 471.62692342, 486.79873586, 503.001588 , 520.23916932, 538.65972073, 558.39716512, 579.53489312, 602.08847409, 626.0138677 , 650.88698937, 676.49849997, 702.72429095, 729.44256967, 756.57729948, 784.14295428, 812.17022983, 840.74023092, 870.22663658, 901.01287784, 933.44788861, 967.80607003, 1004.27506418, 1042.86619138, 1083.39244999, 1125.74788074, 1169.70123326, 1214.90802809, 1260.95060504, 1307.42731787, 1354.131065 , 1400.90452483, 1447.68243853, 1494.44623011, 1540.91970505, 1586.24139667, 1629.19255653, 1669.88742156, 1699.39319048, 1778.49464711, 1860.4850019 , 1945.35893574, 2033.19363905, 2123.99021731, 2217.73593633, 2314.51188391])
- guess 11(pressure)float64275.7 276.7 ... 2.217e+03 2.314e+03
- units :
- Kelvin
array([ 275.65114239, 276.70945095, 277.23181866, 277.88279288, 278.64272243, 279.52341647, 280.5318079 , 281.63578557, 282.8547005 , 284.21384236, 285.70191198, 287.30579552, 289.0052621 , 290.77910549, 292.57254901, 294.4051525 , 296.29840267, 298.24703507, 300.2526841 , 302.32264279, 304.49526272, 306.83131554, 309.37128349, 312.13810758, 315.14135769, 318.3740578 , 321.81221256, 325.4874543 , 329.40715233, 333.50462436, 337.75648787, 342.19816077, 346.89193486, 351.94606307, 357.56455407, 363.65985458, 370.14020831, 377.04676672, 384.44035026, 392.41117271, 401.10384876, 410.74992552, 421.11272445, 432.18347037, 444.08518311, 456.93927603, 470.86073358, 486.02452409, 502.2019085 , 519.39734685, 537.75555485, 557.40224283, 578.40856139, 600.77617493, 624.44996284, 649.00781967, 674.25329349, 700.08568268, 726.41254304, 753.18422495, 780.43092936, 808.18607834, 836.52261606, 865.80120755, 896.39306761, 928.63737729, 962.80130065, 999.06738685, 1037.44379301, 1077.74310798, 1119.86181018, 1163.57317105, 1208.54030224, 1254.35669217, 1300.63434664, 1347.18329926, 1393.84707715, 1440.55166068, 1487.307547 , 1534.04644744, 1580.45184815, 1625.79550008, 1668.89753412, 1698.87293543, 1777.95531945, 1859.92634841, 1944.7808156 , 2032.59558684, 2123.37297429, 2217.09905418, 2313.85491877])
- guess 12(pressure)float64266.7 268.2 ... 2.217e+03 2.314e+03
- units :
- Kelvin
array([ 266.65423093, 268.23544002, 269.0334897 , 269.99510739, 271.09510388, 272.34319421, 273.74241142, 275.25431786, 276.89467559, 278.68238419, 280.59834025, 282.62181061, 284.7256225 , 286.88324365, 289.03463441, 291.20307486, 293.41001021, 295.64962924, 297.92404779, 300.24156168, 302.64336786, 305.19298698, 307.93207517, 310.88406458, 314.05847478, 317.4479642 , 321.02808975, 324.83125248, 328.86494345, 333.06164156, 337.3982084 , 341.91113652, 346.66407477, 351.76680803, 357.42481921, 363.55122324, 370.05564488, 376.98049301, 384.38765711, 392.36828388, 401.06780775, 410.71846469, 421.08404564, 432.15633158, 444.05871863, 456.9128733 , 470.83395092, 485.99703783, 502.17346324, 519.3677019 , 537.7244277 , 557.36924667, 578.37313779, 600.73753348, 624.40705648, 648.95947281, 674.19835146, 700.02326747, 726.34232215, 753.10651991, 780.3465783 , 808.09612267, 836.42795836, 865.70242152, 896.29036713, 928.53065481, 962.69019033, 998.95134011, 1037.32216566, 1077.61527892, 1119.72730066, 1163.43174191, 1208.39208285, 1254.20231966, 1300.47506193, 1347.0210178 , 1393.68394499, 1440.38977566, 1487.15004227, 1533.90197643, 1580.33972774, 1625.73191616, 1668.86320608, 1698.84110048, 1777.92231737, 1859.89215023, 1944.7454257 , 2032.55897674, 2123.33518934, 2217.06006693, 2313.81470206])
- guess 13(pressure)float64267.5 269.1 ... 2.255e+03 2.353e+03
- units :
- Kelvin
array([ 267.48058866, 269.06374045, 269.86438448, 270.82805277, 271.9292456 , 273.17859463, 274.58025672, 276.1001256 , 277.75449556, 279.55939802, 281.4952312 , 283.54140928, 285.6707667 , 287.85663353, 290.03923534, 292.24081756, 294.48241415, 296.75941126, 299.07420345, 301.43531197, 303.88232119, 306.47695723, 309.26007386, 312.25442483, 315.46898498, 318.89607992, 322.51151148, 326.34760205, 330.41310857, 334.64319566, 339.01586849, 343.5671241 , 348.35954498, 353.50170579, 359.19745624, 365.362185 , 371.90767788, 378.87716656, 386.33286142, 394.36541412, 403.11975709, 412.82649103, 423.25281351, 434.39233721, 446.36907683, 459.306342 , 473.3201065 , 488.58739636, 504.88178901, 522.20463918, 540.69908758, 560.49031072, 581.6492666 , 604.17346221, 628.00539097, 652.71735748, 678.11714389, 704.112436 , 730.62496588, 757.61667977, 785.13018988, 813.20552973, 841.92060108, 871.64053647, 902.76167702, 935.60290493, 970.41079605, 1007.34825163, 1046.38748684, 1087.29782663, 1129.92266225, 1174.01967975, 1219.27719298, 1265.35036641, 1311.93781278, 1358.92189175, 1406.2196319 , 1453.81785585, 1501.78583817, 1550.14274512, 1598.69332726, 1646.90387861, 1693.93441913, 1730.16817385, 1810.39508558, 1893.50579957, 1979.52720599, 2068.53673571, 2160.46357631, 2255.3659312 , 2353.2633242 ])
- guess 14(pressure)float64277.1 278.7 ... 2.658e+03 2.766e+03
- units :
- Kelvin
array([ 277.07812164, 278.68495747, 279.51591185, 280.50383599, 281.61968081, 282.8844592 , 284.31511206, 285.9268673 , 287.7421501 , 289.74430293, 291.90800312, 294.21431965, 296.63611819, 299.14511655, 301.68439779, 304.26439574, 306.9015268 , 309.60472669, 312.37948849, 315.23678199, 318.19767406, 321.3034245 , 324.58586877, 328.06019307, 331.72932879, 335.58197667, 339.59681981, 343.80534195, 348.2307701 , 352.83879776, 357.62038023, 362.60588562, 367.84586742, 373.43527214, 379.55968829, 386.16031656, 393.17329775, 400.65113554, 408.65961181, 417.2837161 , 426.66264402, 437.00853792, 448.12684473, 460.03161341, 472.85371333, 486.73479771, 501.79979005, 518.24728016, 535.87589521, 554.65127789, 574.69884436, 596.13870769, 619.04168978, 643.36141643, 669.01720156, 695.52143706, 722.72988557, 750.64091099, 779.32763846, 808.87233409, 839.44759345, 871.16134038, 904.14379138, 938.80725763, 975.82197362, 1015.27633142, 1057.1841313 , 1101.48671022, 1147.76262655, 1195.32104878, 1243.45721704, 1291.78987399, 1340.30489542, 1389.32432938, 1439.46423992, 1491.36541551, 1545.7151698 , 1603.1332787 , 1664.28065916, 1730.00619221, 1801.21037708, 1879.28358715, 1966.98615166, 2066.51691084, 2158.17152036, 2252.69446258, 2350.11570795, 2450.13167064, 2552.85545777, 2658.15018109, 2765.71195425])
- guess 15(pressure)float64290.6 292.3 ... 2.924e+03 3.035e+03
- units :
- Kelvin
array([ 290.63182268, 292.29135551, 293.16827369, 294.19834817, 295.34771846, 296.64809603, 298.13022979, 299.86405103, 301.87937686, 304.12346155, 306.56408701, 309.18368042, 311.95453891, 314.84587028, 317.80160143, 320.81864587, 323.90937847, 327.09672888, 330.38855203, 333.79704954, 337.32051746, 340.97702944, 344.79022674, 348.76914244, 352.91223338, 357.20638211, 361.63465301, 366.22816372, 371.02703276, 376.03047265, 381.2430256 , 386.688909 , 392.4066677 , 398.47785496, 405.07187401, 412.15778074, 419.6956182 , 427.74694103, 436.38031481, 445.67380031, 455.75955153, 466.83073532, 478.72877811, 491.48493413, 505.23513219, 520.13675276, 536.32001148, 554.00125602, 573.00127208, 593.24425295, 614.83387234, 637.88453468, 662.47075718, 688.50887767, 715.90596052, 744.13304348, 773.09677827, 802.87399177, 833.66014978, 865.62121284, 899.0129852 , 933.96442543, 970.60600295, 1009.33047392, 1050.95432027, 1095.30588092, 1142.16210314, 1191.26085251, 1241.93419969, 1293.33982564, 1344.72608949, 1395.88575944, 1447.20280748, 1499.41665342, 1553.57681884, 1610.65476454, 1671.64680647, 1737.40009879, 1808.76916761, 1886.84021982, 1972.73116395, 2068.07436792, 2176.09373751, 2298.64488594, 2396.88261064, 2497.60071788, 2600.94389713, 2706.34214082, 2813.83373476, 2923.6214013 , 3035.04763927])
- guess 16(pressure)float64344.6 346.5 ... 3.644e+03 3.758e+03
- units :
- Kelvin
array([ 344.56010598, 346.52549525, 347.61397907, 348.8597475 , 350.21336753, 351.73314401, 353.48814789, 355.70388528, 358.43326844, 361.52141068, 364.91481659, 368.59809225, 372.53963827, 376.69887604, 381.01294462, 385.43820671, 389.98047369, 394.70083283, 399.61060434, 404.72070075, 409.953997 , 415.25830668, 420.64053924, 426.10012985, 431.63013466, 437.21901891, 442.86585595, 448.60075379, 454.51723347, 460.71571058, 467.23965284, 474.09470814, 481.28688133, 488.86144755, 496.95059001, 505.6065548 , 514.85248434, 524.77346227, 535.44187841, 546.91062561, 559.28583269, 572.70436129, 587.0991377 , 602.53659287, 619.16023444, 637.1623389 , 656.67792715, 677.96453819, 700.90556333, 725.30331372, 751.20325787, 778.71918625, 807.9650608 , 838.78098944, 871.07053584, 904.2205586 , 938.27045957, 973.47297061, 1010.29436911, 1049.05394348, 1090.15413399, 1133.71084811, 1179.79547088, 1228.68627246, 1281.49905853, 1337.40021321, 1395.6220145 , 1455.48449011, 1515.94029258, 1576.19094966, 1635.91800214, 1695.43559348, 1756.15326097, 1819.65298694, 1887.79287321, 1962.23432161, 2044.61767926, 2136.31321764, 2238.70986854, 2353.58340995, 2482.76717218, 2628.76415703, 2796.79495359, 2989.76670932, 3095.88077597, 3203.01808009, 3311.34244525, 3420.79229205, 3531.46820223, 3643.56956846, 3757.50676057])
- guess 17(pressure)float64334.7 336.7 ... 3.46e+03 3.573e+03
- units :
- Kelvin
array([ 334.74881609, 336.70736352, 337.77582216, 339.00972874, 340.36485705, 341.88850536, 343.63541135, 345.77374344, 348.34789085, 351.24031108, 354.4045516 , 357.8234719 , 361.46562413, 365.29268264, 369.23960607, 373.27638715, 377.41407016, 381.69998765, 386.14287339, 390.75207206, 395.47220958, 400.2739557 , 405.17363516, 410.17786457, 415.28481338, 420.48494263, 425.7726265 , 431.17738884, 436.77638685, 442.63815319, 448.79302429, 455.25246275, 462.03412642, 469.1959491 , 476.88611466, 485.13069245, 493.92932495, 503.35797139, 513.4854846 , 524.37022074, 536.12364117, 548.899607 , 562.59670833, 577.26421199, 593.04119884, 610.10559689, 628.58991227, 648.73856621, 670.42040008, 693.48333825, 717.99963762, 744.09371707, 771.87983516, 801.23114039, 832.05750865, 863.77260294, 896.36326069, 930.00927715, 965.0705908 , 1001.79836592, 1040.53304664, 1081.38654863, 1124.44795646, 1170.03438788, 1219.16636636, 1271.24336982, 1325.69636778, 1382.00163336, 1439.27628422, 1496.78163675, 1554.15510399, 1611.50473488, 1669.91703278, 1730.68594461, 1795.39250902, 1865.49510083, 1942.44059045, 2027.45597978, 2121.79858709, 2227.07312045, 2344.93755458, 2477.67415971, 2630.02481483, 2804.93811033, 2911.06238527, 3018.23307278, 3126.57473209, 3236.26277591, 3347.23124372, 3459.55841036, 3573.30748867])
- guess 18(pressure)float64333.5 335.5 ... 3.436e+03 3.549e+03
- units :
- Kelvin
array([ 333.532991 , 335.49191985, 336.5567531 , 337.78848017, 339.14290195, 340.66578503, 342.40996952, 344.53603224, 347.08775637, 349.95258435, 353.08476283, 356.46680067, 360.06711285, 363.84745838, 367.7417105 , 371.72142759, 375.79864761, 380.01891139, 384.39067143, 388.92312899, 393.56336741, 398.28479053, 403.10531086, 408.03282283, 413.06645164, 418.19721974, 423.41905626, 428.76129923, 434.29887931, 440.09624653, 446.18204862, 452.56840984, 459.27440297, 466.35937151, 473.97319352, 482.13815065, 490.85038252, 500.1843502 , 510.20826389, 520.98107299, 532.61460015, 545.26474931, 558.82615674, 573.34521269, 588.96010034, 605.84657726, 624.13699999, 644.07462468, 665.52770057, 688.35136027, 712.62263932, 738.46852079, 766.00425727, 795.10761497, 825.68792665, 857.15940651, 889.49755677, 922.86771099, 957.61100783, 993.96576626, 1032.26141877, 1072.60877951, 1115.10012995, 1160.059058 , 1208.49266896, 1259.84414162, 1313.58561163, 1369.23599849, 1425.97728206, 1483.12885154, 1540.36533228, 1597.69984921, 1656.14538673, 1716.92247551, 1781.55070036, 1851.44833552, 1928.03223842, 2012.51288896, 2106.13064453, 2210.45505744, 2327.08553103, 2458.21612905, 2608.53223499, 2781.26511358, 2887.16953559, 2994.31538851, 3102.61906995, 3212.25451231, 3323.23536983, 3435.56786628, 3549.31244033])
- guess 19(pressure)float64333.5 335.5 ... 3.435e+03 3.549e+03
- units :
- Kelvin
array([ 333.50155552, 335.46075949, 336.52558522, 337.75737983, 339.11192024, 340.63492934, 342.37918431, 344.5050363 , 347.05625957, 349.9204495 , 353.051868 , 356.43301131, 360.03228714, 363.81145205, 367.70432428, 371.6825029 , 375.75806748, 379.97650983, 384.34627408, 388.87655669, 393.51448936, 398.23353894, 403.05167412, 407.9768364 , 413.0081872 , 418.13677433, 423.35652029, 428.69675404, 434.23235118, 440.02766346, 446.11129599, 452.49538671, 459.19904342, 466.28164092, 473.89310038, 482.05560219, 490.7651805 , 500.09624846, 510.11699087, 520.88636411, 532.51618684, 545.16241182, 558.71957902, 573.23397946, 588.84376331, 605.72462067, 624.00890926, 643.93987282, 665.3857925 , 688.20203376, 712.46580707, 738.30421631, 765.83259263, 794.92883768, 825.50223212, 856.96690608, 889.29793461, 922.66013101, 957.39400864, 993.73743211, 1032.01945603, 1072.35082645, 1114.82391013, 1159.7624825 , 1208.17331845, 1259.50109594, 1313.21952204, 1368.84946166, 1425.57628793, 1482.72310931, 1539.96737816, 1597.3173571 , 1655.78239485, 1716.57935817, 1781.22510846, 1851.13644513, 1927.72954265, 2012.21486037, 2105.83277357, 2210.15223328, 2326.77114257, 2457.88113138, 2608.1673088 , 2780.87206021, 2886.77278462, 2993.91816743, 3102.22116457, 3211.85568343, 3322.83669575, 3435.16923288, 3548.91368725])
- guess 20(pressure)float64333.7 335.6 ... 3.505e+03 3.619e+03
- units :
- Kelvin
array([ 333.68476254, 335.64548325, 336.71120919, 337.94408223, 339.30048192, 340.82607951, 342.57337729, 344.70160745, 347.25392803, 350.11894775, 353.25153955, 356.63412572, 360.23517884, 364.01664271, 367.91306281, 371.89559985, 375.97578266, 380.19817316, 384.57131978, 389.10469057, 393.74629704, 398.46965586, 403.29257351, 408.22168442, 413.25592614, 418.38672374, 423.60835111, 428.95036784, 434.4861894 , 440.28008229, 446.36139154, 452.74330764, 459.4451847 , 466.52664062, 474.13779288, 482.29974622, 491.00811548, 500.33832039, 510.35718094, 521.12202712, 532.74570663, 545.38654807, 558.93861182, 573.44964483, 589.0561974 , 605.93132275, 624.20985671, 644.13406524, 665.57197921, 688.38026698, 712.63630265, 738.46873893, 765.99170256, 795.0863175 , 825.66273508, 857.13958222, 889.49509445, 922.89374202, 957.6739067 , 994.07498548, 1032.42500371, 1072.83698214, 1115.39669747, 1160.41005579, 1208.86927 , 1260.20538095, 1313.90333606, 1369.48357119, 1426.14430035, 1483.20304881, 1540.36436222, 1597.65109044, 1656.08454524, 1716.89348551, 1781.6292255 , 1851.79868044, 1928.96261598, 2014.62247685, 2110.64162077, 2219.95198833, 2347.17470577, 2493.41862952, 2660.11574231, 2850.37877312, 2956.62850911, 3063.82775737, 3172.22437595, 3281.9670087 , 3392.89531411, 3505.19679363, 3618.93988497])
- guess 21(pressure)float64355.1 357.2 ... 5.2e+03 5.2e+03
- units :
- Kelvin
array([ 355.05342994, 357.19124674, 358.36204807, 359.72079999, 361.29396596, 363.12121259, 365.22309087, 367.62861363, 370.30925187, 373.27148351, 376.54128499, 380.0924663 , 383.90104929, 387.9507824 , 392.26084233, 396.75138107, 401.36981619, 406.0524502 , 410.81995662, 415.71341175, 420.78325048, 426.00879855, 431.38899307, 436.77830748, 442.14962184, 447.53829214, 452.9795183 , 458.52967279, 464.09211598, 469.72120031, 475.53242033, 481.66151864, 488.156541 , 495.10544333, 502.68120514, 510.77961783, 519.34745935, 528.57745062, 538.37732993, 548.61480944, 559.52258045, 571.53591735, 584.4929996 , 598.61139892, 613.84113905, 630.04772887, 647.6550015 , 666.79116421, 687.29501024, 709.17518089, 732.52811712, 757.66320476, 784.55401188, 813.45772608, 844.38612118, 877.28259651, 912.49396452, 950.14410221, 990.32193057, 1033.44469898, 1079.71930127, 1129.52280249, 1182.17243911, 1235.89394418, 1289.98737881, 1342.29880276, 1393.62159326, 1443.42339469, 1492.39469897, 1539.19824073, 1586.69464111, 1636.60891276, 1691.36062684, 1753.56822586, 1828.80157704, 1929.06043044, 2072.67436509, 2294.71244513, 2668.30892697, 3349.99807102, 4675.38221271, 5199.9 , 5199.9 , 5199.9 , 5199.9 , 5199.9 , 5199.9 , 5199.9 , 5199.9 , 5199.9 , 5199.9 ])
- guess 22(pressure)float64353.3 355.4 ... 5.2e+03 5.2e+03
- units :
- Kelvin
array([ 353.31925782, 355.44703807, 356.61154612, 357.96205254, 359.51216 , 361.30281765, 363.36044127, 365.73766283, 368.41873798, 371.38809855, 374.65864727, 378.20584275, 382.00439349, 386.0344039 , 390.30059699, 394.73193907, 399.28638447, 403.91749439, 408.64327114, 413.49954129, 418.52000655, 423.68363519, 428.9928665 , 434.32687271, 439.66297829, 445.02999008, 450.45705705, 455.99490123, 461.57164335, 467.24369972, 473.11308886, 479.29639796, 485.83746208, 492.82114379, 500.41692465, 508.53738429, 517.13655729, 526.3927391 , 536.2318886 , 546.54145076, 557.53629848, 569.62062499, 582.63975254, 596.7969287 , 612.06162785, 628.3262987 , 645.98798829, 665.18396473, 685.75339704, 707.69185771, 731.09333047, 756.25537821, 783.15994154, 812.04251887, 842.90957788, 875.67295732, 910.64551409, 947.92725133, 987.59078454, 1030.0008773 , 1075.33158872, 1123.89095751, 1175.07567978, 1227.45836482, 1280.72777853, 1333.07788364, 1385.09278994, 1436.15369535, 1486.61945103, 1535.06728509, 1583.89825885, 1634.69745864, 1689.87635454, 1752.04307026, 1826.41219486, 1923.28640309, 2056.01140478, 2246.31571308, 2534.7816152 , 3001.6679909 , 3807.89901249, 4501.02197123, 5199.9 , 5199.9 , 5199.9 , 5199.9 , 5199.9 , 5199.9 , 5199.9 , 5199.9 , 5199.9 ])
- guess 23(pressure)float64353.3 355.4 ... 5.2e+03 5.2e+03
- units :
- Kelvin
array([ 353.27951046, 355.40752724, 356.57216045, 357.92276791, 359.47251509, 361.26240586, 363.31905718, 365.69588456, 368.37755125, 371.34772074, 374.61886412, 378.16650184, 381.96530985, 385.99529366, 390.26076068, 394.69095524, 399.24413024, 403.87431783, 408.59942267, 413.45512298, 418.47466892, 423.63704045, 428.9447818 , 434.27772389, 439.61331134, 444.98018538, 450.40734268, 455.94532539, 461.52282992, 467.19632183, 473.06746751, 479.25237459, 485.79478301, 492.77951337, 500.37597465, 508.49710101, 517.09709309, 526.35397532, 536.19402345, 546.50498705, 557.50136531, 569.58683982, 582.60687895, 596.76456212, 612.02967318, 628.29502449, 645.95729195, 665.15386173, 685.72389331, 707.66279103, 731.06450013, 756.22645 , 783.13065817, 812.01238798, 842.87803557, 875.63908576, 910.60795184, 947.88418343, 987.5398293 , 1029.93836353, 1075.25232252, 1123.78724803, 1174.94001004, 1227.29120051, 1280.54256068, 1332.89984318, 1384.94090209, 1436.03977337, 1486.5434577 , 1535.02371918, 1583.87572686, 1634.68626714, 1689.86999672, 1752.03689961, 1826.39904792, 1923.23307388, 2055.71103674, 2244.49582983, 2524.871543 , 2957.03327206, 3639.53750946, 4422.7605889 , 5199.9 , 5199.9 , 5199.9 , 5199.9 , 5199.9 , 5199.9 , 5199.9 , 5199.9 , 5199.9 ])
- guess 24(pressure)float64353.3 355.4 ... 5.2e+03 5.2e+03
- units :
- Kelvin
array([ 353.27895182, 355.40698181, 356.57162223, 357.92223731, 359.47198498, 361.26187077, 363.31851462, 365.69534408, 368.37702774, 371.34721716, 374.6183769 , 378.1660285 , 381.96484768, 385.99483868, 390.26030385, 394.69049148, 399.24365842, 403.87384221, 408.59894593, 413.45464595, 418.47418755, 423.63655112, 428.94428228, 434.27721884, 439.61280652, 444.97968482, 450.40684885, 455.94483866, 461.52235571, 467.1958661 , 473.06703285, 479.251959 , 485.79438363, 492.77912716, 500.37559795, 508.4967332 , 517.09673514, 526.35362615, 536.19368422, 546.50466094, 557.50105248, 569.58653684, 582.60658362, 596.76427144, 612.02938634, 628.294743 , 645.95701503, 665.15358939, 685.72362551, 707.66252671, 731.06423795, 756.22618789, 783.13039436, 812.01211945, 842.87775888, 875.63879572, 910.60764119, 947.88384309, 987.53944796, 1029.93792468, 1075.25180388, 1123.78661895, 1174.93924515, 1227.29031253, 1280.5416204 , 1332.89896725, 1384.9401758 , 1436.0392418 , 1486.54311379, 1535.02352868, 1583.8756369 , 1634.68623234, 1689.86998571, 1752.03689047, 1826.39901326, 1923.23290866, 2055.71013652, 2244.4894969 , 2524.80296907, 2956.24046097, 3632.37313556, 4421.76209939, 5199.9 , 5199.9 , 5199.9 , 5199.9 , 5199.9 , 5199.9 , 5199.9 , 5199.9 , 5199.9 ])
- guess 25(pressure)float64353.4 355.5 ... 5.2e+03 5.2e+03
- units :
- Kelvin
array([ 353.38425553, 355.51313091, 356.67859023, 358.02963585, 359.57947801, 361.36857599, 363.42331169, 365.79682402, 368.47437335, 371.43983556, 374.70535732, 378.24621441, 382.03732404, 386.05966034, 390.31797971, 394.74247218, 399.29263875, 403.92478016, 408.65391445, 413.51309627, 418.53484019, 423.69807639, 429.00530782, 434.33885537, 439.67611541, 445.04459635, 450.47248878, 456.00982155, 461.58787294, 467.26300041, 473.13599748, 479.32208217, 485.86517704, 492.84998271, 500.44911886, 508.57264257, 517.16918149, 526.41360335, 536.24143433, 546.54776105, 557.54542583, 569.63692113, 582.66755271, 596.82598299, 612.08882984, 628.35972237, 646.02653819, 665.22666359, 685.79997288, 707.74205254, 731.15110437, 756.3186002 , 783.231341 , 812.11991723, 842.99282794, 875.76376847, 910.7454488 , 948.03619761, 987.7131773 , 1030.13760384, 1075.48822455, 1124.06632145, 1175.22319601, 1227.56963968, 1280.73888874, 1333.02294375, 1384.97439017, 1436.01057641, 1486.44846686, 1534.90949846, 1583.76510288, 1634.60539368, 1689.84598945, 1752.10653805, 1826.64975587, 1923.98508527, 2057.827216 , 2250.65562864, 2537.73574509, 2972.24349555, 3647.18758285, 4444.03819165, 5199.9 , 5199.9 , 5199.9 , 5199.9 , 5199.9 , 5199.9 , 5199.9 , 5199.9 , 5199.9 ])
- guess 26(pressure)float64357.8 360.0 ... 5.2e+03 5.2e+03
- units :
- Kelvin
array([ 357.81872832, 359.98321608, 361.18314613, 362.55233618, 364.10619024, 365.8621896 , 367.83669816, 370.0707003 , 372.57433794, 375.34091291, 378.36918442, 381.62407046, 385.09063302, 388.79067199, 392.74806967, 396.93269631, 401.35647876, 406.07107022, 410.96996654, 415.97579275, 421.09029147, 426.29027897, 431.57646184, 436.93573988, 442.34343276, 447.77940967, 453.23797769, 458.7476348 , 464.34818782, 470.0914189 , 476.04150066, 482.27637635, 488.84769767, 495.83511834, 503.54650908, 511.77062581, 520.22131329, 528.94051868, 538.25325313, 548.36365771, 559.41494636, 571.75966168, 585.2361808 , 599.42586768, 614.59314811, 631.09721922, 648.95541337, 668.30509447, 689.01625204, 711.09218567, 734.81039868, 760.21145115, 787.48360679, 816.6606948 , 847.83979972, 881.02777768, 916.54990284, 954.45314499, 995.03005304, 1038.54691704, 1085.44413134, 1135.84400346, 1187.17995091, 1239.33242225, 1289.04784974, 1338.24586853, 1386.41588385, 1434.80204316, 1482.45830141, 1530.10200248, 1579.10508346, 1631.19752968, 1688.83434947, 1755.04085468, 1837.20994876, 1955.63325084, 2146.7237978 , 2508.44975824, 3075.79602574, 3638.70395455, 4266.6987772 , 5199.9 , 5199.9 , 5199.9 , 5199.9 , 5199.9 , 5199.9 , 5199.9 , 5199.9 , 5199.9 , 5199.9 ])
- guess 27(pressure)float64357.8 359.9 ... 5.2e+03 5.2e+03
- units :
- Kelvin
array([ 357.78521386, 359.94899993, 361.14816379, 362.51710691, 364.07117878, 365.82851598, 367.80567559, 370.04377766, 372.55202924, 375.32331776, 378.35650792, 381.61642402, 385.08761875, 388.79117802, 392.75094344, 396.93681193, 401.36092395, 406.0748892 , 410.97267062, 415.97740026, 421.09110219, 426.29067357, 431.57685042, 436.93577631, 442.34279351, 447.77809985, 453.23626366, 458.74594364, 464.34613794, 470.08866284, 476.03795202, 482.27224865, 488.84315896, 495.83036891, 503.54066156, 511.76375863, 520.2154931 , 528.93785079, 538.25222282, 548.36265711, 559.41345303, 571.75715113, 585.23155337, 599.42065153, 614.58787779, 631.09033737, 648.94693405, 668.29514033, 689.0048089 , 711.07916465, 734.79436436, 760.19271233, 787.46081713, 816.63421426, 847.80917685, 880.99138693, 916.50561658, 954.39905859, 994.96056994, 1038.45651986, 1085.32102524, 1135.67956523, 1187.01554848, 1239.18348857, 1288.97740309, 1338.22134092, 1386.410224 , 1434.78723968, 1482.41613071, 1530.04817278, 1579.05506409, 1631.16628145, 1688.82495368, 1755.03206997, 1837.0971713 , 1954.70474951, 2140.97133039, 2476.24140768, 2982.00710694, 3529.48454122, 4192.77846209, 5199.9 , 5199.9 , 5199.9 , 5199.9 , 5199.9 , 5199.9 , 5199.9 , 5199.9 , 5199.9 , 5199.9 ])
- guess 28(pressure)float64357.8 359.9 ... 5.2e+03 5.2e+03
- units :
- Kelvin
array([ 357.78521219, 359.94899824, 361.14816207, 362.5171052 , 364.0711771 , 365.82851438, 367.80567415, 370.04377643, 372.55202825, 375.323317 , 378.35650738, 381.6164237 , 385.08761863, 388.79117804, 392.75094355, 396.93681208, 401.3609241 , 406.07488932, 410.9726707 , 415.97740029, 421.09110219, 426.29067355, 431.5768504 , 436.93577628, 442.34279345, 447.77809977, 453.23626356, 458.74594355, 464.34613783, 470.08866271, 476.03795186, 482.27224847, 488.84315877, 495.83036871, 503.54066133, 511.76375837, 520.21549287, 528.93785063, 538.25222269, 548.36265698, 559.41345288, 571.75715095, 585.23155313, 599.42065128, 614.58787753, 631.09033707, 648.94693371, 668.29513995, 689.00480848, 711.0791642 , 734.79436385, 760.19271176, 787.46081647, 816.63421354, 847.80917605, 880.99138603, 916.50561555, 954.3990574 , 994.96056851, 1038.45651811, 1085.32102302, 1135.67956249, 1187.01554584, 1239.18348631, 1288.97740196, 1338.22134046, 1386.41022381, 1434.78723939, 1482.4161301 , 1530.04817203, 1579.05506341, 1631.16628103, 1688.82495352, 1755.03206977, 1837.09716972, 1954.70473661, 2140.97119955, 2476.2394581 , 2981.9965137 , 3529.47409715, 4192.77497404, 5199.9 , 5199.9 , 5199.9 , 5199.9 , 5199.9 , 5199.9 , 5199.9 , 5199.9 , 5199.9 , 5199.9 ])
- guess 29(pressure)float64357.8 360.0 ... 6.097e+03 6.271e+03
- units :
- Kelvin
array([ 357.8003729 , 359.96432481, 361.16354519, 362.53254188, 364.08660576, 365.84383432, 367.8207398 , 370.0583979 , 372.56606391, 375.33638522, 378.36769447, 381.62565278, 385.09553535, 388.79809438, 392.75722606, 396.94276276, 401.36802101, 406.08252852, 410.979904 , 415.98428086, 421.09762296, 426.29710903, 431.5833029 , 436.94229405, 442.3494029 , 447.78479465, 453.24309505, 458.752852 , 464.35315542, 470.09585378, 476.045448 , 482.27985358, 488.85077436, 495.83791694, 503.55010589, 511.77153759, 520.22077592, 528.9411065 , 538.2555705 , 548.3662895 , 559.418262 , 571.76329746, 585.23726921, 599.4257569 , 614.59375271, 631.09667939, 648.95371243, 668.30213707, 689.01191911, 711.08641043, 734.80242995, 760.20141687, 787.47091782, 816.64563112, 847.82195169, 881.006881 , 916.52344206, 954.41923506, 994.98459805, 1038.48445157, 1085.35530154, 1135.71395465, 1187.04988568, 1239.1972765 , 1288.98001959, 1338.21294059, 1386.39957464, 1434.77846317, 1482.41240583, 1530.05021987, 1579.06103716, 1631.17270386, 1688.83263585, 1755.04548552, 1837.15039848, 1954.93083341, 2142.11747785, 2479.67964097, 2984.85945638, 3533.02571976, 4200.30788693, 5199.9 , 5199.9 , 5199.9 , 5332.90857392, 5472.07955418, 5617.71606105, 5770.13531948, 5929.67342639, 6096.69005004, 6271.49333412])
- guess 30(pressure)float64357.8 360.0 ... 6.097e+03 6.271e+03
- units :
- Kelvin
array([ 357.8003729 , 359.96432481, 361.16354519, 362.53254188, 364.08660576, 365.84383432, 367.8207398 , 370.0583979 , 372.56606391, 375.33638522, 378.36769447, 381.62565278, 385.09553535, 388.79809438, 392.75722606, 396.94276276, 401.36802101, 406.08252852, 410.979904 , 415.98428086, 421.09762296, 426.29710903, 431.5833029 , 436.94229405, 442.3494029 , 447.78479465, 453.24309505, 458.752852 , 464.35315542, 470.09585378, 476.045448 , 482.27985358, 488.85077436, 495.83791694, 503.55010589, 511.77153759, 520.22077592, 528.9411065 , 538.2555705 , 548.3662895 , 559.418262 , 571.76329746, 585.23726921, 599.4257569 , 614.59375271, 631.09667939, 648.95371243, 668.30213707, 689.01191911, 711.08641043, 734.80242995, 760.20141687, 787.47091782, 816.64563112, 847.82195169, 881.006881 , 916.52344206, 954.41923506, 994.98459805, 1038.48445157, 1085.35530154, 1135.71395465, 1187.04988568, 1239.1972765 , 1288.98001959, 1338.21294059, 1386.39957464, 1434.77846317, 1482.41240583, 1530.05021987, 1579.06103716, 1631.17270386, 1688.83263585, 1755.04548552, 1837.15039848, 1954.93083341, 2142.11747785, 2479.67964097, 2984.85945638, 3533.02571976, 4200.30788693, 5199.9 , 5199.9 , 5199.9 , 5332.90857392, 5472.07955418, 5617.71606105, 5770.13531948, 5929.67342639, 6096.69005004, 6271.49333412])
- guess 31(pressure)float64357.8 360.0 ... 5.2e+03 5.2e+03
- units :
- Kelvin
array([ 357.81526779, 359.97938323, 361.17865841, 362.54770845, 364.10176398, 365.85888602, 367.83554181, 370.07276372, 372.57985448, 375.34922418, 378.37868348, 381.63471983, 385.10331372, 388.80489064, 392.76340027, 396.94861139, 401.37499588, 406.09003568, 410.98701152, 415.99104133, 421.10403001, 426.30343258, 431.58964353, 436.94869906, 442.35589815, 447.79137419, 453.24980882, 458.75964135, 464.36005198, 470.10292071, 476.0528144 , 482.28732699, 488.8582581 , 495.84533463, 503.55938522, 511.77918083, 520.22596894, 528.94431192, 538.258867 , 548.36986586, 559.42299318, 571.7693414 , 585.24289038, 599.43077919, 614.59953032, 631.10291563, 648.9603771 , 668.30901622, 689.01890996, 711.09353609, 734.81035995, 760.20997382, 787.48084512, 816.65685089, 847.83450482, 881.0221044 , 916.54095579, 954.43905869, 995.00820599, 1038.51189566, 1085.38898057, 1135.7477454 , 1187.08362423, 1239.21084914, 1288.98263575, 1338.2047676 , 1386.38920614, 1434.76994017, 1482.40883374, 1530.05229808, 1579.06695721, 1631.17906904, 1688.84024899, 1755.05875629, 1837.20285433, 1955.15373295, 2143.25161292, 2483.05026488, 2987.67262136, 3536.52166221, 4207.76296474, 5199.9 , 5199.9 , 5199.9 , 5199.9 , 5199.9 , 5199.9 , 5199.9 , 5199.9 , 5199.9 , 5199.9 ])
- guess 32(pressure)float64357.8 360.0 ... 6.097e+03 6.271e+03
- units :
- Kelvin
array([ 357.82990292, 359.99417816, 361.19350787, 362.56260972, 364.1166573 , 365.87367475, 367.8500852 , 370.08687876, 372.59340467, 375.36183828, 378.3894783 , 381.64362798, 385.1109564 , 388.81156915, 392.76946824, 396.95435991, 401.38185099, 406.09741328, 410.99399565, 415.99768398, 421.11032551, 426.30964633, 431.59587441, 436.95499345, 442.36228135, 447.79784055, 453.25640707, 458.76631381, 464.36682979, 470.1098658 , 476.06005349, 482.29467116, 488.86561243, 495.85262422, 503.56850243, 511.78669067, 520.23107361, 528.94746779, 538.2621131 , 548.37338704, 559.42764785, 571.77528465, 585.24841837, 599.43571966, 614.60521216, 631.10904775, 648.96692982, 668.31577959, 689.02578325, 711.10054339, 734.81815634, 760.21838537, 787.49060163, 816.66787656, 847.84683963, 881.03706132, 916.55816258, 954.45853488, 995.03140015, 1038.53885941, 1085.42207113, 1135.78094579, 1187.11677256, 1239.22420787, 1288.98524977, 1338.1968159 , 1386.3791114 , 1434.76166378, 1482.40540923, 1530.05440469, 1579.07282348, 1631.18537657, 1688.84779309, 1755.07188334, 1837.25454916, 1955.37348375, 2144.3737454 , 2486.35306493, 2990.43702235, 3539.96297471, 4215.14150622, 4316.59688029, 5199.9 , 5199.9 , 5332.90857392, 5472.07955418, 5617.71606105, 5770.13531948, 5929.67342639, 6096.69005004, 6271.49333412])
- guess 33(pressure)float64357.8 360.0 ... 6.097e+03 6.271e+03
- units :
- Kelvin
array([ 357.82990292, 359.99417816, 361.19350787, 362.56260972, 364.1166573 , 365.87367475, 367.8500852 , 370.08687876, 372.59340467, 375.36183828, 378.3894783 , 381.64362798, 385.1109564 , 388.81156915, 392.76946824, 396.95435991, 401.38185099, 406.09741328, 410.99399565, 415.99768398, 421.11032551, 426.30964633, 431.59587441, 436.95499345, 442.36228135, 447.79784055, 453.25640707, 458.76631381, 464.36682979, 470.1098658 , 476.06005349, 482.29467116, 488.86561243, 495.85262422, 503.56850243, 511.78669067, 520.23107361, 528.94746779, 538.2621131 , 548.37338704, 559.42764785, 571.77528465, 585.24841837, 599.43571966, 614.60521216, 631.10904775, 648.96692982, 668.31577959, 689.02578325, 711.10054339, 734.81815634, 760.21838537, 787.49060163, 816.66787656, 847.84683963, 881.03706132, 916.55816258, 954.45853488, 995.03140015, 1038.53885941, 1085.42207113, 1135.78094579, 1187.11677256, 1239.22420787, 1288.98524977, 1338.1968159 , 1386.3791114 , 1434.76166378, 1482.40540923, 1530.05440469, 1579.07282348, 1631.18537657, 1688.84779309, 1755.07188334, 1837.25454916, 1955.37348375, 2144.3737454 , 2486.35306493, 2990.43702235, 3539.96297471, 4215.14150622, 4316.59688029, 5199.9 , 5199.9 , 5332.90857392, 5472.07955418, 5617.71606105, 5770.13531948, 5929.67342639, 6096.69005004, 6271.49333412])
- guess 34(pressure)float64357.8 360.0 ... 5.2e+03 5.2e+03
- units :
- Kelvin
array([ 357.84546502, 360.00990994, 361.20929791, 362.57845467, 364.13249387, 365.88940006, 367.86554997, 370.10188815, 372.60781378, 375.3752508 , 378.40095457, 381.65309994, 385.11908349, 388.81867181, 392.77592238, 396.96047485, 401.38914266, 406.10525999, 411.00142311, 416.0047478 , 421.1170203 , 426.3162545 , 431.60250117, 436.96168802, 442.36907052, 447.8047185 , 453.26342526, 458.77341089, 464.37403887, 470.11725267, 476.06775271, 482.30248205, 488.87343416, 495.86037727, 503.57819726, 511.79467646, 520.2365043 , 528.95083052, 538.26557257, 548.37713914, 559.43260401, 571.78160971, 585.25430201, 599.4409795 , 614.61125952, 631.11557356, 648.97390252, 668.3229762 , 689.03309694, 711.10800121, 734.82645199, 760.22733424, 787.50097912, 816.67960284, 847.859957 , 881.05296531, 916.57645848, 954.4792438 , 995.0560623 , 1038.56753044, 1085.4572579 , 1135.81624944, 1187.15202001, 1239.23843761, 1288.98807573, 1338.18844386, 1386.36847547, 1434.75296664, 1482.40185798, 1530.05671324, 1579.07911392, 1631.19214051, 1688.85588306, 1755.08593582, 1837.30968453, 1955.60795102, 2145.57532349, 2489.85590717, 2993.3770667 , 3543.62929118, 4223.04539751, 4350.91020531, 5199.9 , 5199.9 , 5199.9 , 5199.9 , 5199.9 , 5199.9 , 5199.9 , 5199.9 , 5199.9 ])
- guess 35(pressure)float64358.5 360.7 ... 5.2e+03 5.2e+03
- units :
- Kelvin
array([ 358.53406301, 360.70601398, 361.90797824, 363.2795636 , 364.83322926, 366.58521333, 368.54983419, 370.76602276, 373.24538932, 375.96873119, 378.908764 , 382.07222485, 385.47869971, 389.1329576 , 393.06151112, 397.23105319, 401.71178882, 406.45246441, 411.33007564, 416.31730948, 421.41325259, 426.60865341, 431.89572211, 437.25790818, 442.66947573, 448.10905151, 453.57396323, 459.08743848, 464.69302143, 470.44410108, 476.40842073, 482.64809011, 489.21952105, 496.20342446, 504.00715821, 512.14802011, 520.47679368, 529.09961868, 538.41864104, 548.54315513, 559.65189515, 572.06146878, 585.51462951, 599.67370632, 614.87883029, 631.40431293, 649.28241403, 668.64139346, 689.35669383, 711.43797434, 735.19349341, 760.62327547, 787.96012674, 817.19842331, 848.44032147, 881.75661371, 917.38592563, 955.39546192, 996.14716822, 1039.83598165, 1087.01394957, 1137.37811039, 1188.71139632, 1239.86799531, 1289.11309142, 1337.81799495, 1385.89785603, 1434.36813544, 1482.2447157 , 1530.15884054, 1579.35741609, 1631.49138814, 1689.21378939, 1755.70760961, 1839.74868392, 1965.97615734, 2198.64215283, 2644.27659838, 3123.17363633, 3705.51565214, 4571.67118526, 5199.9 , 5199.9 , 5199.9 , 5199.9 , 5199.9 , 5199.9 , 5199.9 , 5199.9 , 5199.9 , 5199.9 ])
- guess 36(pressure)float64358.5 360.7 ... 5.2e+03 5.2e+03
- units :
- Kelvin
array([ 358.54142846, 360.71332514, 361.91523451, 363.28675827, 364.8403317 , 366.59218017, 368.55660899, 370.77253482, 373.25158751, 375.9744699 , 378.91366969, 382.07623656, 385.48208375, 389.1358518 , 393.06408462, 397.23344072, 401.71463449, 406.45547957, 411.33288118, 416.31994038, 421.41571351, 426.61105427, 431.8981011 , 437.26028197, 442.67185277, 448.11142885, 453.57635669, 459.08982807, 464.69541676, 470.44651999, 476.41089893, 482.65056594, 489.22196381, 496.20581503, 504.01001185, 512.15039366, 520.47843618, 529.1006463 , 538.41968896, 548.54428427, 559.65334671, 572.06325541, 585.51629476, 599.67520913, 614.88050824, 631.40608786, 649.28427055, 668.64327742, 689.35857872, 711.43986444, 735.19551588, 760.62538722, 787.96244259, 817.20090283, 848.4429457 , 881.75951544, 917.38899712, 955.39865163, 996.15050759, 1039.83936201, 1087.01725832, 1137.3812479 , 1188.71428496, 1239.86967754, 1289.11311159, 1337.81595625, 1385.8953327 , 1434.36611738, 1482.24380456, 1530.15899752, 1579.35818224, 1631.49220165, 1689.21461288, 1755.70856029, 1839.74763827, 1965.88678593, 2196.81061177, 2633.96026802, 3117.7590957 , 3699.23506534, 4551.54239402, 5199.9 , 5199.9 , 5199.9 , 5199.9 , 5199.9 , 5199.9 , 5199.9 , 5199.9 , 5199.9 , 5199.9 ])
- guess 37(pressure)float64358.5 360.7 ... 6.097e+03 6.271e+03
- units :
- Kelvin
array([ 358.54189378, 360.71379632, 361.91570737, 363.28723308, 364.84080688, 366.59265303, 368.55707558, 370.77298922, 373.25202691, 375.97486758, 378.9139917 , 382.07651357, 385.48232628, 389.13607025, 393.06428933, 397.23363916, 401.7148692 , 406.45572653, 411.33310739, 416.32015054, 421.41591306, 426.61125379, 431.89830451, 437.26048982, 442.67206501, 448.11164714, 453.57657949, 459.0900531 , 464.69564538, 470.44675353, 476.41114018, 482.65081108, 489.22220939, 496.20607659, 504.0102949 , 512.15061684, 520.47860373, 529.10078978, 538.41984373, 548.54445008, 559.65353953, 572.06347968, 585.51650684, 599.67541041, 614.88072692, 631.40631828, 649.28451169, 668.6435246 , 689.35883116, 711.44013597, 735.19580226, 760.62568575, 787.96277211, 817.20126772, 848.44334555, 881.75998926, 917.38954256, 955.39927256, 996.1512528 , 1039.84024067, 1087.01835494, 1137.38234761, 1188.71537287, 1239.87031803, 1289.11358566, 1337.81641265, 1385.89585118, 1434.36673973, 1482.24446247, 1530.15963877, 1579.35880016, 1631.49286607, 1689.21541003, 1755.70976451, 1839.7508761 , 1965.90304713, 2196.93370405, 2634.0756409 , 3117.89816424, 3699.46599979, 3794.09041757, 3890.50362438, 5199.9 , 5199.9 , 5332.90857392, 5472.07955418, 5617.71606105, 5770.13531948, 5929.67342639, 6096.69005004, 6271.49333412])
- guess 38(pressure)float64358.5 360.7 ... 6.097e+03 6.271e+03
- units :
- Kelvin
array([ 358.54189378, 360.71379632, 361.91570737, 363.28723308, 364.84080688, 366.59265303, 368.55707558, 370.77298922, 373.25202691, 375.97486758, 378.9139917 , 382.07651357, 385.48232628, 389.13607025, 393.06428933, 397.23363916, 401.7148692 , 406.45572653, 411.33310739, 416.32015054, 421.41591306, 426.61125379, 431.89830451, 437.26048982, 442.67206501, 448.11164714, 453.57657949, 459.0900531 , 464.69564538, 470.44675353, 476.41114018, 482.65081108, 489.22220939, 496.20607659, 504.0102949 , 512.15061684, 520.47860373, 529.10078978, 538.41984373, 548.54445008, 559.65353953, 572.06347968, 585.51650684, 599.67541041, 614.88072692, 631.40631828, 649.28451169, 668.6435246 , 689.35883116, 711.44013597, 735.19580226, 760.62568575, 787.96277211, 817.20126772, 848.44334555, 881.75998926, 917.38954256, 955.39927256, 996.1512528 , 1039.84024067, 1087.01835494, 1137.38234761, 1188.71537287, 1239.87031803, 1289.11358566, 1337.81641265, 1385.89585118, 1434.36673973, 1482.24446247, 1530.15963877, 1579.35880016, 1631.49286607, 1689.21541003, 1755.70976451, 1839.7508761 , 1965.90304713, 2196.93370405, 2634.0756409 , 3117.89816424, 3699.46599979, 3794.09041757, 3890.50362438, 5199.9 , 5199.9 , 5332.90857392, 5472.07955418, 5617.71606105, 5770.13531948, 5929.67342639, 6096.69005004, 6271.49333412])
- guess 39(pressure)float64358.5 360.7 ... 5.2e+03 5.2e+03
- units :
- Kelvin
array([ 358.54472116, 360.71665937, 361.91858021, 363.29011804, 364.84369399, 366.5955264 , 368.55991055, 370.77575027, 373.25469682, 375.97728406, 378.91594861, 382.0781972 , 385.48380042, 389.13739814, 393.0655338 , 397.2348455 , 401.71629589, 406.45722763, 411.33448242, 416.32142814, 421.41712627, 426.61246677, 431.89954111, 437.26175345, 442.6733553 , 448.11297416, 453.57793394, 459.09142104, 464.69703518, 470.44817324, 476.41260677, 482.6523013 , 489.22370228, 496.2076667 , 504.01201533, 512.15197356, 520.47962259, 529.10166248, 538.42078509, 548.54545849, 559.65471199, 572.06484325, 585.51779636, 599.67663437, 614.8820566 , 631.40771927, 649.28597784, 668.64502752, 689.36036604, 711.44178687, 735.19754335, 760.62750071, 787.96477539, 817.20348584, 848.44577608, 881.76286907, 917.39285743, 955.40304604, 996.15578124, 1039.84557968, 1087.02501787, 1137.38902939, 1188.72198311, 1239.87421056, 1289.11646728, 1337.81918608, 1385.89900107, 1434.37051938, 1482.24845758, 1530.16353277, 1579.36255302, 1631.49690248, 1689.22025409, 1755.7170842 , 1839.77055819, 1966.00191962, 2197.68239205, 2634.77720052, 3118.74354144, 3700.86973461, 3795.4920507 , 3917.42530481, 5199.9 , 5199.9 , 5199.9 , 5199.9 , 5199.9 , 5199.9 , 5199.9 , 5199.9 , 5199.9 ])
- guess 40(pressure)float64358.7 360.8 ... 5.2e+03 5.2e+03
- units :
- Kelvin
array([ 358.65417213, 360.82749103, 362.02979036, 363.40179683, 364.95545564, 366.70675546, 368.66965293, 370.88263049, 373.35804855, 376.07082519, 378.9916988 , 382.14336834, 385.54086198, 389.18879832, 393.11370464, 397.28154019, 401.77151983, 406.51533187, 411.38770654, 416.37088046, 421.46408621, 426.65941778, 431.94740635, 437.31066456, 442.72329833, 448.1643392 , 453.63036028, 459.14436955, 464.75082945, 470.50312538, 476.46937326, 482.70998215, 489.28148651, 496.2692136 , 504.07860583, 512.20448638, 520.51905859, 529.13544128, 538.45722096, 548.58448947, 559.70009285, 572.11762088, 585.56770817, 599.72400813, 614.93352246, 631.46194505, 649.34272577, 668.70319827, 689.41977352, 711.50568475, 735.26493194, 760.69774807, 788.04231099, 817.28933669, 848.53984743, 881.8743287 , 917.52115472, 955.54909201, 996.331045 , 1040.05221377, 1087.28288847, 1137.64762875, 1188.97781226, 1240.02486097, 1289.22799296, 1337.92652425, 1386.02090822, 1434.51679848, 1482.40307506, 1530.31423629, 1579.50779327, 1631.65311479, 1689.40772098, 1756.00035351, 1840.53223111, 1969.82742562, 2226.62695585, 2661.90884994, 3151.43984326, 3755.15485349, 3849.68518104, 4946.93408007, 5199.9 , 5199.9 , 5199.9 , 5199.9 , 5199.9 , 5199.9 , 5199.9 , 5199.9 , 5199.9 ])
- guess 41(pressure)float64358.7 360.8 ... 5.2e+03 5.2e+03
- units :
- Kelvin
array([ 358.65567703, 360.82899334, 362.03128312, 363.40328028, 364.95692257, 366.70819617, 368.67105359, 370.88397271, 373.35932498, 376.07195433, 378.99258034, 382.14410425, 385.54148816, 389.18934858, 393.11421044, 397.28202221, 401.7720934 , 406.51592896, 411.38824315, 416.37137227, 421.46454822, 426.65987589, 431.94786931, 437.31113329, 442.7237725 , 448.1648221 , 453.6308484 , 459.14485813, 464.75132116, 470.50362251, 476.46988069, 482.71049206, 489.28199231, 496.26974339, 504.07916733, 512.20493642, 520.51940757, 529.1357475 , 538.45754594, 548.58483293, 559.70047984, 572.11805611, 585.56812376, 599.72440695, 614.93394624, 631.4623852 , 649.34318001, 668.70365948, 689.42024029, 711.50617431, 735.26543778, 760.69826631, 788.04286323, 817.28992627, 848.54047271, 881.87502831, 917.52192172, 955.54992627, 996.33198482, 1040.05325846, 1087.28408352, 1137.64879441, 1188.97891284, 1240.02558523, 1289.22855014, 1337.92704849, 1386.02147126, 1434.5174295 , 1482.40371884, 1530.3148485 , 1579.50836505, 1631.65369145, 1689.40835388, 1756.00117634, 1840.53387783, 1969.82025842, 2226.1329365 , 2661.60183342, 3151.11347378, 3754.48582913, 3849.01741683, 4788.35028532, 5199.9 , 5199.9 , 5199.9 , 5199.9 , 5199.9 , 5199.9 , 5199.9 , 5199.9 , 5199.9 ])
- guess 42(pressure)float64358.7 360.8 ... 5.2e+03 5.2e+03
- units :
- Kelvin
array([ 358.65569895, 360.82901493, 362.03130433, 363.40330114, 364.95694293, 366.70821589, 368.67107245, 370.88399046, 373.35934155, 376.0719686 , 378.99259102, 382.14411286, 385.54149526, 389.18935465, 393.11421591, 397.28202732, 401.77209954, 406.51593528, 411.3882487 , 416.37137728, 421.46455286, 426.65988045, 431.94787386, 437.31113785, 442.72377705, 448.16482669, 453.63085299, 459.14486267, 464.75132568, 470.50362702, 476.46988524, 482.71049657, 489.28199673, 496.26974795, 504.07917208, 512.20494024, 520.51941057, 529.13575016, 538.45754872, 548.58483583, 559.70048303, 572.11805961, 585.56812711, 599.72441017, 614.9339496 , 631.46238864, 649.34318352, 668.70366301, 689.42024382, 711.50617793, 735.26544145, 760.69827001, 788.04286706, 817.28993025, 848.54047681, 881.87503271, 917.52192637, 955.54993116, 996.33199009, 1040.05326408, 1087.2840896 , 1137.64880019, 1188.97891811, 1240.0255889 , 1289.22855303, 1337.92705115, 1386.021474 , 1434.51743243, 1482.40372174, 1530.31485119, 1579.50836749, 1631.65369378, 1689.40835625, 1756.0011791 , 1840.53388241, 1969.8202282 , 2226.13178976, 2661.60134037, 3151.11309219, 3754.48522447, 3849.0168133 , 4784.3914611 , 5199.9 , 5199.9 , 5199.9 , 5199.9 , 5199.9 , 5199.9 , 5199.9 , 5199.9 , 5199.9 ])
- guess 43(pressure)float64358.7 360.8 ... 6.097e+03 6.271e+03
- units :
- Kelvin
array([ 358.65582173, 360.82913913, 362.03142913, 363.40342665, 364.95706888, 366.70834182, 368.6711978 , 370.88411415, 373.35946304, 376.07208276, 378.99269215, 382.1442068 , 385.54158376, 389.18943946, 393.11429876, 397.28210941, 401.7721888 , 406.51602703, 411.38833675, 416.37146279, 421.46463706, 426.65996527, 431.94795996, 437.31122524, 442.7238657 , 448.16491689, 453.63094444, 459.14495497, 464.75141916, 470.50372201, 476.46998236, 482.71059545, 489.28209623, 496.26985927, 504.07927481, 512.20502965, 520.51949318, 529.13582947, 538.45763121, 548.58492106, 559.70057519, 572.1181595 , 585.56822553, 599.72450731, 614.93405168, 631.46249443, 649.34329285, 668.70377501, 689.42035847, 711.50629833, 735.26556675, 760.6983995 , 788.04300434, 817.29007605, 848.5406311 , 881.87520248, 917.52211122, 955.55013203, 996.33221604, 1040.05351735, 1087.28438666, 1137.64910186, 1188.97922101, 1240.02580704, 1289.22873384, 1337.92719274, 1386.02159629, 1434.51752482, 1482.40379866, 1530.31492776, 1579.50846553, 1631.65384945, 1689.40860005, 1756.0016342 , 1840.5351787 , 1969.82787131, 2226.20312841, 2661.65954157, 3151.17170095, 3754.58313338, 3849.11453799, 3945.37904855, 4043.32540961, 5199.9 , 5332.90857392, 5472.07955418, 5617.71606105, 5770.13531948, 5929.67342639, 6096.69005004, 6271.49333412])
- guess 44(pressure)float64358.7 360.8 ... 6.097e+03 6.271e+03
- units :
- Kelvin
array([ 358.65582173, 360.82913913, 362.03142913, 363.40342665, 364.95706888, 366.70834182, 368.6711978 , 370.88411415, 373.35946304, 376.07208276, 378.99269215, 382.1442068 , 385.54158376, 389.18943946, 393.11429876, 397.28210941, 401.7721888 , 406.51602703, 411.38833675, 416.37146279, 421.46463706, 426.65996527, 431.94795996, 437.31122524, 442.7238657 , 448.16491689, 453.63094444, 459.14495497, 464.75141916, 470.50372201, 476.46998236, 482.71059545, 489.28209623, 496.26985927, 504.07927481, 512.20502965, 520.51949318, 529.13582947, 538.45763121, 548.58492106, 559.70057519, 572.1181595 , 585.56822553, 599.72450731, 614.93405168, 631.46249443, 649.34329285, 668.70377501, 689.42035847, 711.50629833, 735.26556675, 760.6983995 , 788.04300434, 817.29007605, 848.5406311 , 881.87520248, 917.52211122, 955.55013203, 996.33221604, 1040.05351735, 1087.28438666, 1137.64910186, 1188.97922101, 1240.02580704, 1289.22873384, 1337.92719274, 1386.02159629, 1434.51752482, 1482.40379866, 1530.31492776, 1579.50846553, 1631.65384945, 1689.40860005, 1756.0016342 , 1840.5351787 , 1969.82787131, 2226.20312841, 2661.65954157, 3151.17170095, 3754.58313338, 3849.11453799, 3945.37904855, 4043.32540961, 5199.9 , 5332.90857392, 5472.07955418, 5617.71606105, 5770.13531948, 5929.67342639, 6096.69005004, 6271.49333412])
- guess 45(pressure)float64358.7 360.8 ... 5.2e+03 5.2e+03
- units :
- Kelvin
array([ 358.65679251, 360.8301206 , 362.03241624, 363.40441861, 364.95806469, 366.70933723, 368.67218875, 370.8850919 , 373.36042353, 376.07298521, 378.99349179, 382.14494957, 385.54228353, 389.19011005, 393.11495388, 397.28275844, 401.77289453, 406.51675249, 411.38903288, 416.37213887, 421.46530274, 426.6606359 , 431.94864066, 437.31191619, 442.72456654, 448.16562999, 453.63166744, 459.14568473, 464.75215821, 470.50447297, 476.4707502 , 482.71137721, 489.28288286, 496.2707394 , 504.08008699, 512.20573656, 520.52014628, 529.13645652, 538.45828336, 548.58559482, 559.70130378, 572.11894925, 585.56900364, 599.7252752 , 614.93485869, 631.46333076, 649.34415725, 668.70466051, 689.4212649 , 711.50725016, 735.26655731, 760.69942324, 788.0440897 , 817.29122882, 848.54185088, 881.87654469, 917.52357256, 955.55171997, 996.33400224, 1040.05551948, 1087.28673477, 1137.65148632, 1188.98161526, 1240.0275314 , 1289.2301632 , 1337.92831206, 1386.02256323, 1434.51825567, 1482.40440755, 1530.31553411, 1579.50924174, 1631.65508119, 1689.41052847, 1756.0052333 , 1840.5454288 , 1969.88831668, 2226.7674894 , 2662.11997176, 3151.63528719, 3755.35780411, 3849.88774876, 3946.15001693, 4096.8699798 , 5199.9 , 5199.9 , 5199.9 , 5199.9 , 5199.9 , 5199.9 , 5199.9 , 5199.9 ])
- guess 46(pressure)float64358.7 360.8 ... 6.097e+03 6.271e+03
- units :
- Kelvin
array([ 358.65773971, 360.8310766 , 362.03337806, 363.40538577, 364.95903458, 366.71030761, 368.67315427, 370.88604477, 373.36135963, 376.07386504, 378.99427171, 382.14567428, 385.54296649, 389.19076461, 393.11559337, 397.28339192, 401.7735832 , 406.51746031, 411.38971209, 416.37279851, 421.46595218, 426.66129009, 431.94930458, 437.31259004, 442.72525 , 448.16632538, 453.63237245, 459.14639632, 464.75287886, 470.50520522, 476.47149891, 482.71213949, 489.2836499 , 496.27159757, 504.0808789 , 512.20642596, 520.52078325, 529.13706788, 538.45891913, 548.5862516 , 559.7020141 , 572.11971925, 585.56976224, 599.72602378, 614.93564545, 631.46414613, 649.345 , 668.70552385, 689.42214865, 711.50817823, 735.26752317, 760.70042147, 788.04514803, 817.29235289, 848.54304027, 881.87785334, 917.52499715, 955.55326771, 996.33574272, 1040.05746973, 1087.28902089, 1137.65380754, 1188.98394581, 1240.02921105, 1289.23155595, 1337.92940327, 1386.02350686, 1434.51897156, 1482.40500699, 1530.31613296, 1579.51000701, 1631.65629001, 1689.41241648, 1756.0087511 , 1840.55543709, 1969.94740914, 2227.32064595, 2662.57125823, 3152.08913025, 3249.66100533, 3347.98615076, 3447.13342483, 3547.07491119, 5199.9 , 5332.90857392, 5472.07955418, 5617.71606105, 5770.13531948, 5929.67342639, 6096.69005004, 6271.49333412])
- guess 47(pressure)float64358.7 360.8 ... 6.097e+03 6.271e+03
- units :
- Kelvin
array([ 358.65773971, 360.8310766 , 362.03337806, 363.40538577, 364.95903458, 366.71030761, 368.67315427, 370.88604477, 373.36135963, 376.07386504, 378.99427171, 382.14567428, 385.54296649, 389.19076461, 393.11559337, 397.28339192, 401.7735832 , 406.51746031, 411.38971209, 416.37279851, 421.46595218, 426.66129009, 431.94930458, 437.31259004, 442.72525 , 448.16632538, 453.63237245, 459.14639632, 464.75287886, 470.50520522, 476.47149891, 482.71213949, 489.2836499 , 496.27159757, 504.0808789 , 512.20642596, 520.52078325, 529.13706788, 538.45891913, 548.5862516 , 559.7020141 , 572.11971925, 585.56976224, 599.72602378, 614.93564545, 631.46414613, 649.345 , 668.70552385, 689.42214865, 711.50817823, 735.26752317, 760.70042147, 788.04514803, 817.29235289, 848.54304027, 881.87785334, 917.52499715, 955.55326771, 996.33574272, 1040.05746973, 1087.28902089, 1137.65380754, 1188.98394581, 1240.02921105, 1289.23155595, 1337.92940327, 1386.02350686, 1434.51897156, 1482.40500699, 1530.31613296, 1579.51000701, 1631.65629001, 1689.41241648, 1756.0087511 , 1840.55543709, 1969.94740914, 2227.32064595, 2662.57125823, 3152.08913025, 3249.66100533, 3347.98615076, 3447.13342483, 3547.07491119, 5199.9 , 5332.90857392, 5472.07955418, 5617.71606105, 5770.13531948, 5929.67342639, 6096.69005004, 6271.49333412])
- guess 48(pressure)float64358.7 360.8 ... 5.2e+03 5.2e+03
- units :
- Kelvin
array([ 358.6587475 , 360.83209735, 362.03440351, 363.40641749, 364.96006968, 366.71134275, 368.67418435, 370.8870621 , 373.36235888, 376.07480467, 378.99510509, 382.14644901, 385.54369679, 389.19146471, 393.11627742, 397.28406955, 401.77431977, 406.51821732, 411.39043852, 416.37350401, 421.46664671, 426.66198964, 431.95001448, 437.3133105 , 442.7259807 , 448.16706879, 453.63312615, 459.14715703, 464.75364924, 470.50598801, 476.47229929, 482.71295438, 489.28446988, 496.27251497, 504.08172544, 512.20716307, 520.52146434, 529.13772139, 538.45959866, 548.58695351, 559.70277332, 572.12054235, 585.57057309, 599.72682383, 614.93648637, 631.46501766, 649.34590082, 668.70644667, 689.4230933 , 711.50917031, 735.26855569, 760.70148861, 788.04627946, 817.29355458, 848.54431177, 881.87925219, 917.52651973, 955.55492162, 996.33760206, 1040.05955248, 1087.29146113, 1137.65628493, 1188.98643295, 1240.03100481, 1289.23304381, 1337.93056959, 1386.02451649, 1434.51974034, 1482.4056539 , 1530.31678122, 1579.51083402, 1631.65759052, 1689.41444295, 1756.01252063, 1840.56615063, 1970.01074314, 2227.91503456, 2663.05617977, 3152.57623415, 3250.14159321, 3348.46184042, 3447.60409178, 3603.14997277, 5199.9 , 5199.9 , 5199.9 , 5199.9 , 5199.9 , 5199.9 , 5199.9 , 5199.9 ])
- guess 49(pressure)float64358.7 360.8 ... 6.097e+03 6.271e+03
- units :
- Kelvin
array([ 358.65974716, 360.83310815, 362.03541986, 363.40743921, 364.96109502, 366.71236848, 368.6752051 , 370.88806982, 373.36334916, 376.07573607, 378.99593161, 382.14721762, 385.54442156, 389.19215963, 393.11695642, 397.28474213, 401.77505064, 406.51896838, 411.39115927, 416.37420396, 421.46733573, 426.66268353, 431.95071856, 437.31402498, 442.72670527, 448.16780595, 453.63387347, 459.14791129, 464.75441309, 470.50676415, 476.47309287, 482.71376235, 489.28528291, 496.27342455, 504.08256475, 512.20789405, 520.52213981, 529.13836929, 538.46027229, 548.58764926, 559.70352596, 572.12135839, 585.57137693, 599.7276169 , 614.93732 , 631.46588165, 649.34679386, 668.70736156, 689.42402984, 711.51015392, 735.26957942, 760.70254669, 788.04740131, 817.2947461 , 848.54557246, 881.88063901, 917.52802897, 955.55656074, 996.33944421, 1040.06161524, 1087.29387663, 1137.65873685, 1188.98889432, 1240.03278135, 1289.23451793, 1337.9317258 , 1386.02551851, 1434.52050635, 1482.40630189, 1530.31743269, 1579.51166362, 1631.65888888, 1689.41646099, 1756.01626778, 1840.57678859, 1970.0737141 , 2228.5076825 , 2663.53967632, 2763.83199495, 2865.08355806, 2967.10174741, 3069.64626953, 3172.80857205, 5199.9 , 5332.90857392, 5472.07955418, 5617.71606105, 5770.13531948, 5929.67342639, 6096.69005004, 6271.49333412])
- guess 50(pressure)float64358.7 360.8 ... 6.097e+03 6.271e+03
- units :
- Kelvin
array([ 358.65974716, 360.83310815, 362.03541986, 363.40743921, 364.96109502, 366.71236848, 368.6752051 , 370.88806982, 373.36334916, 376.07573607, 378.99593161, 382.14721762, 385.54442156, 389.19215963, 393.11695642, 397.28474213, 401.77505064, 406.51896838, 411.39115927, 416.37420396, 421.46733573, 426.66268353, 431.95071856, 437.31402498, 442.72670527, 448.16780595, 453.63387347, 459.14791129, 464.75441309, 470.50676415, 476.47309287, 482.71376235, 489.28528291, 496.27342455, 504.08256475, 512.20789405, 520.52213981, 529.13836929, 538.46027229, 548.58764926, 559.70352596, 572.12135839, 585.57137693, 599.7276169 , 614.93732 , 631.46588165, 649.34679386, 668.70736156, 689.42402984, 711.51015392, 735.26957942, 760.70254669, 788.04740131, 817.2947461 , 848.54557246, 881.88063901, 917.52802897, 955.55656074, 996.33944421, 1040.06161524, 1087.29387663, 1137.65873685, 1188.98889432, 1240.03278135, 1289.23451793, 1337.9317258 , 1386.02551851, 1434.52050635, 1482.40630189, 1530.31743269, 1579.51166362, 1631.65888888, 1689.41646099, 1756.01626778, 1840.57678859, 1970.0737141 , 2228.5076825 , 2663.53967632, 2763.83199495, 2865.08355806, 2967.10174741, 3069.64626953, 3172.80857205, 5199.9 , 5332.90857392, 5472.07955418, 5617.71606105, 5770.13531948, 5929.67342639, 6096.69005004, 6271.49333412])
- guess 51(pressure)float64358.7 360.8 ... 5.2e+03 5.2e+03
- units :
- Kelvin
array([ 358.66063658, 360.83400718, 362.03632338, 363.40834794, 364.96200704, 366.71328076, 368.67611296, 370.88896624, 373.36423018, 376.07656496, 378.99666765, 382.14790229, 385.54506739, 389.19277897, 393.11756161, 397.28534155, 401.77570188, 406.51963754, 411.39180141, 416.37482758, 421.46794954, 426.66330162, 431.95134563, 437.31466127, 442.7273505 , 448.16846234, 453.63453891, 459.14858288, 464.75509321, 470.50745521, 476.47379945, 482.71448174, 489.28600681, 496.27423439, 504.08331201, 512.20854503, 520.52274139, 529.13894613, 538.46087196, 548.58826857, 559.70419599, 572.12208493, 585.57209255, 599.72832285, 614.93806212, 631.46665083, 649.34758892, 668.70817606, 689.42486363, 711.51102967, 735.27049092, 760.70348879, 788.04840022, 817.29580704, 848.54669496, 881.88187368, 917.52937241, 955.55801951, 996.34108315, 1040.0634498 , 1087.29602371, 1137.66091601, 1188.99108168, 1240.03436137, 1289.2358295 , 1337.93275513, 1386.0264116 , 1434.52119183, 1482.40688479, 1530.31802058, 1579.51241091, 1631.66005292, 1689.41826572, 1756.01961288, 1840.58627426, 1970.12993963, 2229.03835852, 2663.97294852, 2764.26514545, 2865.51689298, 2967.53182428, 3070.07328538, 3232.9475141 , 5199.9 , 5199.9 , 5199.9 , 5199.9 , 5199.9 , 5199.9 , 5199.9 , 5199.9 ])
- guess 52(pressure)float64358.7 360.8 ... 6.097e+03 6.271e+03
- units :
- Kelvin
array([ 358.66153417, 360.83491296, 362.03723477, 363.4092645 , 364.96292645, 366.71420063, 368.67702847, 370.8898702 , 373.36511875, 376.07740115, 378.99741051, 382.14859363, 385.54571965, 389.19340456, 393.11817294, 397.28594702, 401.77635953, 406.5203132 , 411.39244982, 416.37545725, 421.46856927, 426.66392559, 431.95197861, 437.31530349, 442.7280017 , 448.16912479, 453.63521045, 459.14926064, 464.75577955, 470.50815258, 476.47451249, 482.71520772, 489.28673735, 496.27505162, 504.08406608, 512.20920208, 520.52334863, 529.13952822, 538.46147703, 548.58889339, 559.70487205, 572.12281808, 585.57281463, 599.72903512, 614.93881092, 631.46742694, 649.34839117, 668.70899794, 689.42570499, 711.51191341, 735.27141075, 760.70443952, 788.04940831, 817.29687771, 848.54782773, 881.88311952, 917.53072782, 955.55949102, 996.34273593, 1040.06529924, 1087.29818713, 1137.66311145, 1188.99328522, 1240.03595424, 1289.23715221, 1337.93379378, 1386.02731373, 1434.52188672, 1482.40747845, 1530.318621 , 1579.51317292, 1631.66123493, 1689.4200942 , 1756.02299654, 1840.59585929, 1970.18682309, 2229.57664181, 2324.95460932, 2422.2466538 , 2521.51827696, 2622.81070787, 2725.30663646, 2829.14753657, 5199.9 , 5332.90857392, 5472.07955418, 5617.71606105, 5770.13531948, 5929.67342639, 6096.69005004, 6271.49333412])
- guess 53(pressure)float64358.7 360.8 ... 6.097e+03 6.271e+03
- units :
- Kelvin
array([ 358.66153417, 360.83491296, 362.03723477, 363.4092645 , 364.96292645, 366.71420063, 368.67702847, 370.8898702 , 373.36511875, 376.07740115, 378.99741051, 382.14859363, 385.54571965, 389.19340456, 393.11817294, 397.28594702, 401.77635953, 406.5203132 , 411.39244982, 416.37545725, 421.46856927, 426.66392559, 431.95197861, 437.31530349, 442.7280017 , 448.16912479, 453.63521045, 459.14926064, 464.75577955, 470.50815258, 476.47451249, 482.71520772, 489.28673735, 496.27505162, 504.08406608, 512.20920208, 520.52334863, 529.13952822, 538.46147703, 548.58889339, 559.70487205, 572.12281808, 585.57281463, 599.72903512, 614.93881092, 631.46742694, 649.34839117, 668.70899794, 689.42570499, 711.51191341, 735.27141075, 760.70443952, 788.04940831, 817.29687771, 848.54782773, 881.88311952, 917.53072782, 955.55949102, 996.34273593, 1040.06529924, 1087.29818713, 1137.66311145, 1188.99328522, 1240.03595424, 1289.23715221, 1337.93379378, 1386.02731373, 1434.52188672, 1482.40747845, 1530.318621 , 1579.51317292, 1631.66123493, 1689.4200942 , 1756.02299654, 1840.59585929, 1970.18682309, 2229.57664181, 2324.95460932, 2422.2466538 , 2521.51827696, 2622.81070787, 2725.30663646, 2829.14753657, 5199.9 , 5332.90857392, 5472.07955418, 5617.71606105, 5770.13531948, 5929.67342639, 6096.69005004, 6271.49333412])
- guess 54(pressure)float64358.7 360.8 ... 5.2e+03 5.2e+03
- units :
- Kelvin
array([ 358.66224622, 360.83563386, 362.03795907, 363.40999329, 364.96365792, 366.71493225, 368.67775724, 370.89059013, 373.36582698, 376.07806898, 378.99800595, 382.14914924, 385.54624507, 389.19390943, 393.11866687, 397.28643651, 401.77688988, 406.52085775, 411.39297323, 416.37596617, 421.46907051, 426.66443019, 431.95249029, 437.31582243, 442.72852772, 448.16965965, 453.6357525 , 459.14980761, 464.75633331, 470.50871504, 476.47508728, 482.71579271, 489.28732602, 496.2757081 , 504.08467335, 512.20973383, 520.52384166, 529.14000162, 538.46196851, 548.58940046, 559.70541941, 572.12341032, 585.57339847, 599.72961152, 614.9394162 , 631.46805391, 649.34903893, 668.70966142, 689.4263841 , 711.5126261 , 735.27215215, 760.70520561, 788.05021982, 817.29773875, 848.54873794, 881.88411872, 917.53181325, 955.56066779, 996.34405478, 1040.06677206, 1087.29990504, 1137.66485514, 1188.99503609, 1240.03723102, 1289.23821978, 1337.93464152, 1386.02805728, 1434.5224723 , 1482.40798936, 1530.31914188, 1579.51382492, 1631.66222044, 1689.42159339, 1756.02571632, 1840.60355226, 1970.22993748, 2230.04344764, 2325.43347393, 2422.73336413, 2522.01311078, 2623.30824809, 2725.80718312, 2893.47054492, 5199.9 , 5199.9 , 5199.9 , 5199.9 , 5199.9 , 5199.9 , 5199.9 , 5199.9 ])
- guess 55(pressure)float64358.7 360.8 ... 6.097e+03 6.271e+03
- units :
- Kelvin
array([ 358.66298197, 360.8363767 , 362.03870672, 363.41074462, 364.96441213, 366.71568676, 368.67850862, 370.89133248, 373.36655728, 376.07875775, 378.9986202 , 382.1497225 , 385.54678722, 389.1944304 , 393.1191765 , 397.28694152, 401.777437 , 406.52141947, 411.3935131 , 416.37649105, 421.4695874 , 426.66495049, 431.95301785, 437.31635745, 442.72907 , 448.17021105, 453.63631129, 459.15037147, 464.75690416, 470.50929487, 476.47567983, 482.71639579, 489.28793289, 496.27638496, 504.0852994 , 512.21028199, 520.52434985, 529.14048942, 538.46247493, 548.58992292, 559.70598349, 572.12402076, 585.57400018, 599.73020551, 614.94004001, 631.4687001 , 649.34970657, 668.71034528, 689.42708408, 711.51336077, 735.27291645, 760.70599539, 788.05105647, 817.2986265 , 848.54967642, 881.88514895, 917.53293233, 955.56188093, 996.3454142 , 1040.0682899 , 1087.30167492, 1137.66665135, 1188.99683956, 1240.03854649, 1289.23931974, 1337.93551496, 1386.02882372, 1434.52307705, 1482.4085184 , 1530.3196822 , 1579.51450093, 1631.66324018, 1689.42314303, 1756.02852586, 1840.6114965 , 1970.2745111 , 2058.51241729, 2149.3587151 , 2242.80383086, 2338.9181921 , 2437.06280663, 2537.41461359, 2639.81829234, 5199.9 , 5332.90857392, 5472.07955418, 5617.71606105, 5770.13531948, 5929.67342639, 6096.69005004, 6271.49333412])
- guess 56(pressure)float64358.7 360.8 ... 6.097e+03 6.271e+03
- units :
- Kelvin
array([ 358.66298197, 360.8363767 , 362.03870672, 363.41074462, 364.96441213, 366.71568676, 368.67850862, 370.89133248, 373.36655728, 376.07875775, 378.9986202 , 382.1497225 , 385.54678722, 389.1944304 , 393.1191765 , 397.28694152, 401.777437 , 406.52141947, 411.3935131 , 416.37649105, 421.4695874 , 426.66495049, 431.95301785, 437.31635745, 442.72907 , 448.17021105, 453.63631129, 459.15037147, 464.75690416, 470.50929487, 476.47567983, 482.71639579, 489.28793289, 496.27638496, 504.0852994 , 512.21028199, 520.52434985, 529.14048942, 538.46247493, 548.58992292, 559.70598349, 572.12402076, 585.57400018, 599.73020551, 614.94004001, 631.4687001 , 649.34970657, 668.71034528, 689.42708408, 711.51336077, 735.27291645, 760.70599539, 788.05105647, 817.2986265 , 848.54967642, 881.88514895, 917.53293233, 955.56188093, 996.3454142 , 1040.0682899 , 1087.30167492, 1137.66665135, 1188.99683956, 1240.03854649, 1289.23931974, 1337.93551496, 1386.02882372, 1434.52307705, 1482.4085184 , 1530.3196822 , 1579.51450093, 1631.66324018, 1689.42314303, 1756.02852586, 1840.6114965 , 1970.2745111 , 2058.51241729, 2149.3587151 , 2242.80383086, 2338.9181921 , 2437.06280663, 2537.41461359, 2639.81829234, 5199.9 , 5332.90857392, 5472.07955418, 5617.71606105, 5770.13531948, 5929.67342639, 6096.69005004, 6271.49333412])
- guess 57(pressure)float64358.7 360.8 ... 5.2e+03 5.2e+03
- units :
- Kelvin
array([ 358.66543509, 360.83885611, 362.04120286, 363.41325796, 364.96694339, 366.71823533, 368.68107309, 370.8939094 , 373.36914532, 376.08133449, 379.00116001, 382.15225183, 385.54931406, 389.1969628 , 393.12172269, 397.28950702, 401.78005907, 406.52407643, 411.39617542, 416.37916298, 421.47227272, 426.66765634, 431.95574602, 437.31910751, 442.7318417 , 448.17300605, 453.6391287 , 459.15320946, 464.75976442, 470.51217998, 476.47859406, 482.71933872, 489.29090049, 496.27943093, 504.08833101, 512.21328009, 520.52734566, 529.14349993, 538.46553399, 548.59303174, 559.70916582, 572.12728429, 585.57730472, 599.73355411, 614.94346533, 631.47219986, 649.35328371, 668.71399939, 689.43081944, 711.51719711, 735.27685587, 760.71004132, 788.05523362, 817.30294693, 848.55414927, 881.88981696, 917.53780443, 955.56697227, 996.35077638, 1040.07393963, 1087.30769438, 1137.67287237, 1189.00325368, 1240.04478204, 1289.24561907, 1337.94191559, 1386.03548099, 1434.53004056, 1482.41597986, 1530.32789164, 1579.52384105, 1631.67435699, 1689.43705398, 1756.04837502, 1840.63416838, 1970.52281161, 2058.76878499, 2149.62204697, 2243.0743029 , 2339.19367306, 2437.34344164, 2537.7005818 , 2704.81746803, 5199.9 , 5199.9 , 5199.9 , 5199.9 , 5199.9 , 5199.9 , 5199.9 , 5199.9 ])
- guess 58(pressure)float64358.7 360.8 ... 3.398e+03 3.512e+03
- units :
- Kelvin
array([ 358.66799822, 360.84144812, 362.04381162, 363.41588537, 364.96958918, 366.72089955, 368.68375381, 370.89660315, 373.37185065, 376.08402751, 379.00381327, 382.15489336, 385.55195227, 389.19960617, 393.12437981, 397.29218369, 401.78279591, 406.52684982, 411.39895321, 416.38194981, 421.47507269, 426.67047734, 431.95859013, 437.32197437, 442.7347311 , 448.17591987, 453.64206597, 459.15616819, 464.76274649, 470.51518816, 476.48163298, 482.72240784, 489.29399533, 496.28261016, 504.09149324, 512.21640428, 520.53046524, 529.14663247, 538.4687173 , 548.59626695, 559.71247948, 572.13068456, 585.58074664, 599.73704078, 614.94703301, 631.47584568, 649.35701064, 668.71780667, 689.4347115 , 711.52119546, 735.28096231, 760.71425916, 788.05958952, 817.30745342, 848.55881564, 881.89468905, 917.54289089, 955.57228866, 996.35637745, 1040.07984235, 1087.31398524, 1137.67937108, 1189.00995126, 1240.05127995, 1289.25217443, 1337.94856354, 1386.04238836, 1434.53725633, 1482.42370707, 1530.33639206, 1579.53351552, 1631.68588488, 1689.45149638, 1756.06902279, 1840.65795462, 1970.78180589, 2059.03619348, 2149.89671899, 2243.35642202, 2339.48101596, 2437.63615974, 2537.99886199, 2640.40765428, 2744.27867324, 2849.8283354 , 2956.92161865, 3065.15315411, 3174.69445734, 3285.68252848, 3398.01194546, 3511.73876712])
- guess 59(pressure)float64358.7 360.8 ... 3.398e+03 3.512e+03
- units :
- Kelvin
array([ 358.66799822, 360.84144812, 362.04381162, 363.41588537, 364.96958918, 366.72089955, 368.68375381, 370.89660315, 373.37185065, 376.08402751, 379.00381327, 382.15489336, 385.55195227, 389.19960617, 393.12437981, 397.29218369, 401.78279591, 406.52684982, 411.39895321, 416.38194981, 421.47507269, 426.67047734, 431.95859013, 437.32197437, 442.7347311 , 448.17591987, 453.64206597, 459.15616819, 464.76274649, 470.51518816, 476.48163298, 482.72240784, 489.29399533, 496.28261016, 504.09149324, 512.21640428, 520.53046524, 529.14663247, 538.4687173 , 548.59626695, 559.71247948, 572.13068456, 585.58074664, 599.73704078, 614.94703301, 631.47584568, 649.35701064, 668.71780667, 689.4347115 , 711.52119546, 735.28096231, 760.71425916, 788.05958952, 817.30745342, 848.55881564, 881.89468905, 917.54289089, 955.57228866, 996.35637745, 1040.07984235, 1087.31398524, 1137.67937108, 1189.00995126, 1240.05127995, 1289.25217443, 1337.94856354, 1386.04238836, 1434.53725633, 1482.42370707, 1530.33639206, 1579.53351552, 1631.68588488, 1689.45149638, 1756.06902279, 1840.65795462, 1970.78180589, 2059.03619348, 2149.89671899, 2243.35642202, 2339.48101596, 2437.63615974, 2537.99886199, 2640.40765428, 2744.27867324, 2849.8283354 , 2956.92161865, 3065.15315411, 3174.69445734, 3285.68252848, 3398.01194546, 3511.73876712])
- guess 60(pressure)float64358.7 360.8 ... 3.476e+03 3.59e+03
- units :
- Kelvin
array([ 358.67024387, 360.84371819, 362.04609736, 363.4181865 , 364.97190712, 366.72323317, 368.68610195, 370.89896273, 373.37422013, 376.08638581, 379.00613553, 382.15720467, 385.55425999, 389.20191773, 393.12670279, 397.29452319, 401.78518904, 406.52927501, 411.40138115, 416.38438475, 421.47751832, 426.67294098, 431.96107381, 437.32447785, 442.73725423, 448.17846443, 453.64463109, 459.15875207, 464.76535086, 470.51781553, 476.48428754, 482.72508905, 489.29669898, 496.28538999, 504.09425633, 512.21913131, 520.53318617, 529.14936257, 538.47149187, 548.59908687, 559.71536959, 572.1336521 , 585.58374953, 599.74008166, 614.95014558, 631.47902697, 649.36026316, 668.72112948, 689.43810842, 711.52468613, 735.28454797, 760.71794241, 788.06339451, 817.31139102, 848.56289378, 881.89894892, 917.54733946, 955.57693928, 996.36127886, 1040.0850089 , 1087.31949326, 1137.68505852, 1189.01581008, 1240.05695193, 1289.25788822, 1337.95434622, 1386.04839011, 1434.54351746, 1482.43040778, 1530.34376197, 1579.54190638, 1631.69589541, 1689.46405351, 1756.08701248, 1840.678866 , 1971.00811355, 2059.26985311, 2150.13672495, 2243.60293474, 2339.73209248, 2437.89193237, 2538.25949406, 2640.67056617, 2821.23288932, 2927.48034801, 3034.66415556, 3143.02824519, 3252.7490167 , 3363.70549259, 3476.02573227, 3589.77484186])
- guess 61(pressure)float64358.7 360.8 ... 3.553e+03 3.667e+03
- units :
- Kelvin
array([ 358.6726661 , 360.84616671, 362.04856211, 363.42066916, 364.97440694, 366.72575035, 368.68863477, 370.90150758, 373.37677583, 376.08892887, 379.00863869, 382.15969534, 385.55674612, 389.2044074 , 393.12920421, 397.29704185, 401.78776641, 406.53188702, 411.40399507, 416.38700533, 421.48014968, 426.6755914 , 431.96374562, 437.32717089, 442.7399684 , 448.18120177, 453.64739063, 459.16153181, 464.76815273, 470.52064232, 476.48714392, 482.72797434, 489.29960842, 496.28838372, 504.09723027, 512.22206374, 520.53611007, 529.1522943 , 538.47447157, 548.60211539, 559.71847519, 572.13684272, 585.58697719, 599.74334918, 614.95349112, 631.48244689, 649.36376009, 668.72470213, 689.44176086, 711.5284403 , 735.28840489, 760.72190463, 788.06748878, 817.31562903, 848.56728383, 881.90353645, 917.5521314 , 955.5819498 , 996.36656122, 1040.09057815, 1087.32543224, 1137.69118853, 1189.0221223 , 1240.06305129, 1289.26402463, 1337.9605454 , 1386.05481787, 1434.55021486, 1482.43757142, 1530.35163986, 1579.55087848, 1631.70661089, 1689.47750987, 1756.10632581, 1840.70149457, 1971.25169999, 2059.52135249, 2150.3950547 , 2243.86826753, 2340.00233678, 2438.16723062, 2538.54002207, 2640.9535471 , 2898.18864752, 3004.41891389, 3111.62197532, 3220.05196903, 3329.71735127, 3440.58076568, 3552.83392671, 3666.56654219])
- guess 62(pressure)float64358.7 360.8 ... 3.629e+03 3.743e+03
- units :
- Kelvin
array([ 358.67526978, 360.84880028, 362.05121298, 363.42333844, 364.97709546, 366.72845742, 368.69135869, 370.90424445, 373.37952422, 376.0916632 , 379.01132892, 382.16237136, 385.55941654, 389.20708092, 393.1318897 , 397.29974523, 401.79053399, 406.53469193, 411.40680082, 416.38981721, 421.48297227, 426.67843405, 431.96661108, 437.33005903, 442.74287919, 448.18413753, 453.6503503 , 459.16451314, 464.77115793, 470.52367446, 476.49020819, 482.7310699 , 489.30272987, 496.29159833, 504.10042156, 512.22520734, 520.53924222, 529.15543246, 538.47766134, 548.60535755, 559.72180187, 572.14026257, 585.59043565, 599.74684917, 614.95707584, 631.48611192, 649.36750816, 668.72853154, 689.44567592, 711.53246551, 735.29254094, 760.72615397, 788.07188103, 817.32017668, 848.57199555, 881.90846229, 917.55727813, 955.58733237, 996.37223776, 1040.09656432, 1087.33181776, 1137.69777659, 1189.02890327, 1240.06959007, 1289.27059388, 1337.96716874, 1386.06167812, 1434.55735338, 1482.44520227, 1530.36003013, 1579.56043753, 1631.71804087, 1689.49188096, 1756.12699339, 1840.72591942, 1971.51310658, 2059.79125048, 2150.67228219, 2244.1530098 , 2340.29234901, 2438.46266569, 2538.84106869, 2641.25722501, 2975.14606336, 3081.28553502, 3188.43967694, 3296.80709107, 3406.29715789, 3517.00843524, 3629.13912564, 3743.04813765])
- guess 63(pressure)float64358.7 360.9 ... 3.705e+03 3.819e+03
- units :
- Kelvin
array([ 358.67806386, 360.85162484, 362.05405637, 363.42620197, 364.9799796 , 366.73136118, 368.69428057, 370.90718006, 373.3824721 , 376.09459537, 379.01421223, 382.16523849, 385.56227677, 389.20994362, 393.13476442, 397.30263834, 401.79349714, 406.53769519, 411.40980354, 416.39282531, 421.48599082, 426.68147361, 431.96967481, 437.33314693, 442.74599127, 448.18727647, 453.6535149 , 459.16770092, 464.77437137, 470.52691696, 476.49348548, 482.73438099, 489.30606864, 496.29503991, 504.10383576, 512.22856684, 520.54258682, 529.15878072, 538.48106495, 548.60881722, 559.72535407, 572.14391671, 585.59412974, 599.75058629, 614.96090476, 631.49002734, 649.37151289, 668.73262337, 689.44985941, 711.53676798, 735.29696268, 760.73069725, 788.07657863, 817.32504188, 848.57703735, 881.91373574, 917.56278968, 955.59309775, 996.37832029, 1040.10298016, 1087.33866388, 1137.70483658, 1189.03616656, 1240.07657824, 1289.27760383, 1337.97422118, 1386.06897433, 1434.56493447, 1482.45330085, 1530.36893308, 1579.57058464, 1631.73018971, 1689.50717623, 1756.14903827, 1840.75221677, 1971.79280188, 2060.08003082, 2150.96890415, 2244.45767162, 2340.60264851, 2438.77876647, 2539.16317268, 2641.58214312, 3052.10525596, 3158.08229752, 3265.12128929, 3373.2359823 , 3482.48993856, 3592.99227233, 3705.1321138 , 3819.17128599])
- guess 64(pressure)float64358.7 360.9 ... 3.4e+03 3.513e+03
- units :
- Kelvin
array([ 358.68105156, 360.85464664, 362.05709759, 363.4292656 , 364.9830644 , 366.73446783, 368.69740609, 370.91032034, 373.38562544, 376.09773111, 379.01729413, 382.16830202, 385.56533193, 389.21300045, 393.13783324, 397.30572594, 401.79666108, 406.54090213, 411.41300825, 416.39603441, 421.4892099 , 426.68471458, 431.97294135, 437.33643913, 442.74930924, 448.19062324, 453.65688917, 459.17109993, 464.77779788, 470.53037474, 476.49698089, 482.73791284, 489.30962999, 496.2987146 , 504.10747839, 512.23214688, 520.54614787, 529.16234247, 538.48468595, 548.61249803, 559.72913603, 572.14781006, 585.59806416, 599.75456497, 614.96498273, 631.49419826, 649.37577962, 668.73698314, 689.45431702, 711.54135384, 735.30167658, 760.73554123, 788.08158887, 817.3302325 , 848.58241763, 881.91936614, 917.56867621, 955.59925684, 996.38482082, 1040.10983872, 1087.34598506, 1137.71238266, 1189.043926 , 1240.08402556, 1289.28506188, 1337.98170679, 1386.07670882, 1434.57295818, 1482.46186607, 1530.37834718, 1579.58131895, 1631.74305978, 1689.52340309, 1756.17248172, 1840.78046735, 1972.09125754, 2060.38818035, 2151.28542051, 2244.78276636, 2340.93375794, 2439.11606522, 2539.50687624, 2641.92884813, 2745.81387875, 2851.37854127, 2958.47434313, 3066.70918647, 3176.25468968, 3287.24275867, 3399.5725828 , 3513.30040381])
- guess 65(pressure)float64358.7 360.9 ... 3.4e+03 3.513e+03
- units :
- Kelvin
array([ 358.68105156, 360.85464664, 362.05709759, 363.4292656 , 364.9830644 , 366.73446783, 368.69740609, 370.91032034, 373.38562544, 376.09773111, 379.01729413, 382.16830202, 385.56533193, 389.21300045, 393.13783324, 397.30572594, 401.79666108, 406.54090213, 411.41300825, 416.39603441, 421.4892099 , 426.68471458, 431.97294135, 437.33643913, 442.74930924, 448.19062324, 453.65688917, 459.17109993, 464.77779788, 470.53037474, 476.49698089, 482.73791284, 489.30962999, 496.2987146 , 504.10747839, 512.23214688, 520.54614787, 529.16234247, 538.48468595, 548.61249803, 559.72913603, 572.14781006, 585.59806416, 599.75456497, 614.96498273, 631.49419826, 649.37577962, 668.73698314, 689.45431702, 711.54135384, 735.30167658, 760.73554123, 788.08158887, 817.3302325 , 848.58241763, 881.91936614, 917.56867621, 955.59925684, 996.38482082, 1040.10983872, 1087.34598506, 1137.71238266, 1189.043926 , 1240.08402556, 1289.28506188, 1337.98170679, 1386.07670882, 1434.57295818, 1482.46186607, 1530.37834718, 1579.58131895, 1631.74305978, 1689.52340309, 1756.17248172, 1840.78046735, 1972.09125754, 2060.38818035, 2151.28542051, 2244.78276636, 2340.93375794, 2439.11606522, 2539.50687624, 2641.92884813, 2745.81387875, 2851.37854127, 2958.47434313, 3066.70918647, 3176.25468968, 3287.24275867, 3399.5725828 , 3513.30040381])
- guess 66(pressure)float64358.8 360.9 ... 3.409e+03 3.523e+03
- units :
- Kelvin
array([ 358.76324961, 360.93775339, 362.14077195, 363.51351037, 365.0679275 , 366.81991777, 368.783379 , 370.9966926 , 373.47235353, 376.18395421, 379.10198331, 382.25245407, 385.64922256, 389.29690827, 393.22204358, 397.39042575, 401.88350126, 406.6289275 , 411.50092317, 416.48402873, 421.57744363, 426.77353294, 432.06245379, 437.42665164, 442.84022713, 448.28233556, 453.74935866, 459.26424791, 464.87170454, 470.62514686, 476.59279965, 482.83474174, 489.4072669 , 496.39956786, 504.20736854, 512.33019407, 520.64358284, 529.25970185, 538.58367538, 548.71312818, 559.83261194, 572.25441785, 585.70575218, 599.86341805, 615.07659862, 631.60838267, 649.49260737, 668.85636532, 689.57638338, 711.6669759 , 735.43083364, 760.86827685, 788.2189324 , 817.47256913, 848.72999208, 882.0738883 , 917.73028413, 955.7683915 , 996.56341014, 1040.29831844, 1087.547257 , 1137.91972452, 1189.25701308, 1240.28800056, 1289.48895933, 1338.18583364, 1386.28732997, 1434.7910757 , 1482.69452064, 1530.63400563, 1579.87296831, 1632.09327868, 1689.96566794, 1756.81309838, 1841.56095509, 1980.27739146, 2068.83998717, 2159.96641 , 2253.69874019, 2350.01425388, 2448.36588521, 2548.93194286, 2651.43566606, 2755.40768397, 2861.06554497, 2968.17642359, 3076.43132169, 3186.00249536, 3296.98996543, 3409.32178368, 3523.05533263])
- guess 67(pressure)float64358.8 361.0 ... 3.343e+03 3.457e+03
- units :
- Kelvin
array([ 358.79405829, 360.9689702 , 362.1722068 , 363.5452349 , 365.09988753, 366.85210242, 368.81573313, 371.02910167, 373.50482115, 376.21563939, 379.13171042, 382.2811185 , 385.67696673, 389.32387447, 393.24839039, 397.41623314, 401.91122805, 406.65716962, 411.527795 , 416.50981848, 421.60236727, 426.79820665, 432.08714431, 437.45145217, 442.86520425, 448.30767185, 453.77500733, 459.29009949, 464.8978949 , 470.65180871, 476.62017263, 482.86271129, 489.43545192, 496.43164433, 504.23686825, 512.35571551, 520.66644165, 529.27997404, 538.60457709, 548.73452835, 559.85680309, 572.28163658, 585.73204034, 599.88873263, 615.10380923, 631.63688398, 649.52231973, 668.88691579, 689.607757 , 711.70045226, 735.46599373, 760.90481131, 788.25814247, 817.51451725, 848.77449028, 882.12284949, 917.78303092, 955.82478995, 996.62510087, 1040.36490567, 1087.62052674, 1137.99214703, 1189.32825948, 1240.34152583, 1289.53237764, 1338.21509256, 1386.30958386, 1434.80384944, 1482.70319294, 1530.64209717, 1579.88596633, 1632.12359859, 1690.02303411, 1756.94142633, 1841.95064803, 1925.93298411, 2012.72410812, 2102.31796564, 2194.47918606, 2289.31293551, 2386.51736002, 2485.89707929, 2587.56583302, 2690.93411099, 2795.94757454, 2902.74395343, 3010.84295447, 3120.22174473, 3231.05510657, 3343.35485879, 3457.03223501])
- guess 68(pressure)float64358.8 361.0 ... 3.343e+03 3.457e+03
- units :
- Kelvin
array([ 358.79405829, 360.9689702 , 362.1722068 , 363.5452349 , 365.09988753, 366.85210242, 368.81573313, 371.02910167, 373.50482115, 376.21563939, 379.13171042, 382.2811185 , 385.67696673, 389.32387447, 393.24839039, 397.41623314, 401.91122805, 406.65716962, 411.527795 , 416.50981848, 421.60236727, 426.79820665, 432.08714431, 437.45145217, 442.86520425, 448.30767185, 453.77500733, 459.29009949, 464.8978949 , 470.65180871, 476.62017263, 482.86271129, 489.43545192, 496.43164433, 504.23686825, 512.35571551, 520.66644165, 529.27997404, 538.60457709, 548.73452835, 559.85680309, 572.28163658, 585.73204034, 599.88873263, 615.10380923, 631.63688398, 649.52231973, 668.88691579, 689.607757 , 711.70045226, 735.46599373, 760.90481131, 788.25814247, 817.51451725, 848.77449028, 882.12284949, 917.78303092, 955.82478995, 996.62510087, 1040.36490567, 1087.62052674, 1137.99214703, 1189.32825948, 1240.34152583, 1289.53237764, 1338.21509256, 1386.30958386, 1434.80384944, 1482.70319294, 1530.64209717, 1579.88596633, 1632.12359859, 1690.02303411, 1756.94142633, 1841.95064803, 1925.93298411, 2012.72410812, 2102.31796564, 2194.47918606, 2289.31293551, 2386.51736002, 2485.89707929, 2587.56583302, 2690.93411099, 2795.94757454, 2902.74395343, 3010.84295447, 3120.22174473, 3231.05510657, 3343.35485879, 3457.03223501])
- guess 69(pressure)float64359.0 361.2 ... 3.357e+03 3.471e+03
- units :
- Kelvin
array([ 358.97420245, 361.15111916, 362.35558712, 363.72996198, 365.28611383, 367.03990842, 369.00522865, 371.22034468, 373.69790621, 376.41031561, 379.32756942, 382.47852973, 385.87601547, 389.52466585, 393.45105247, 397.62085212, 402.11865605, 406.86693802, 411.73921325, 416.7229057 , 421.81708149, 427.0145972 , 432.3051907 , 437.67112697, 443.08650991, 448.53067268, 453.99972566, 459.51651107, 465.12606274, 470.88186267, 476.8522982 , 483.096994 , 489.67190169, 496.67152583, 504.47833142, 512.59863338, 520.91148036, 529.52746408, 538.8555673 , 548.98928782, 560.11615429, 572.5460315 , 586.00077312, 600.16201132, 615.38259738, 631.92136699, 649.812812 , 669.18365883, 689.91114944, 712.01129639, 735.78474764, 761.23201383, 788.59489472, 817.8616858 , 849.13294171, 882.49419776, 918.16819841, 956.22482544, 997.04163618, 1040.7986721 , 1088.07321222, 1138.4621557 , 1189.81622816, 1240.84423124, 1290.05565902, 1338.76408906, 1386.89328493, 1435.43352767, 1483.39496734, 1531.41997907, 1580.79017361, 1633.22739209, 1691.50948986, 1758.85286777, 1852.52708689, 1936.85853121, 2024.0072765 , 2113.91136023, 2206.39056196, 2301.55006801, 2398.98867895, 2498.61038657, 2600.52807748, 2704.02257706, 2809.17030989, 2916.10840749, 3024.24320929, 3133.66498774, 3244.54939291, 3356.85927221, 3470.55148934])
- guess 70(pressure)float64359.0 361.2 ... 3.357e+03 3.47e+03
- units :
- Kelvin
array([ 358.97654094, 361.15344811, 362.3579108 , 363.73227939, 365.28842414, 367.04220999, 369.00752036, 371.22262492, 373.70017434, 376.41256667, 379.32979778, 382.48073989, 385.8782086 , 389.5268437 , 393.45321764, 397.62300689, 402.12081093, 406.86908978, 411.74135678, 416.72504296, 421.81921377, 427.01672648, 432.30731753, 437.67325119, 443.08863123, 448.53279116, 454.00184116, 459.51862343, 465.1281723 , 470.88396975, 476.85440364, 483.09909814, 489.67400446, 496.67363577, 504.48043629, 512.60073265, 520.91357846, 529.52956379, 538.85767543, 548.99140606, 560.1182877 , 572.54818249, 586.00293706, 600.16418875, 615.38479257, 631.92357951, 649.81504141, 669.18590368, 689.91340844, 712.01356954, 735.78703308, 761.23431023, 788.59720324, 817.86400724, 849.13527735, 882.49655241, 918.17057495, 956.22722783, 997.04407034, 1040.80114095, 1088.07572018, 1138.46469743, 1189.81880298, 1240.84683062, 1290.05829688, 1338.76677859, 1386.89604582, 1435.4363805 , 1483.39793336, 1531.42307527, 1580.79340008, 1633.23067791, 1691.51255464, 1758.85234859, 1852.44382583, 1936.77252479, 2023.9184578 , 2113.82010361, 2206.29680637, 2301.45375229, 2398.89052578, 2498.51033438, 2600.42607145, 2703.91958513, 2809.06626832, 2916.00325732, 3024.13778542, 3133.5592331 , 3244.44324364, 3356.75305047, 3470.44515761])
- guess 71(pressure)float64359.1 361.3 ... 3.342e+03 3.455e+03
- units :
- Kelvin
array([ 359.07429562, 361.25244897, 362.45770106, 363.8329158 , 365.38994954, 367.1445995 , 369.11068792, 371.32644082, 373.80431435, 376.51485398, 379.42878466, 382.57790291, 385.97379484, 389.62106718, 393.54635663, 397.71517614, 402.21784453, 406.96768791, 411.83702742, 416.81841876, 421.91073545, 427.10783762, 432.39865995, 437.76506089, 443.18108477, 448.62635163, 454.09641998, 459.61396405, 465.22461359, 470.98185134, 476.9543204 , 483.20079757, 489.77656617, 496.78592419, 504.58703125, 512.69824486, 521.00252295, 529.61854052, 538.94894345, 549.08363997, 560.21759726, 572.65528073, 586.10810388, 600.2674054 , 615.4933692 , 632.03608248, 649.93135654, 669.30518355, 690.03570024, 712.14214464, 735.92093729, 761.37288101, 788.7437653 , 818.01882082, 849.29803967, 882.67205026, 918.35792179, 956.42591533, 997.25714904, 1041.02743942, 1088.31902247, 1138.70780344, 1190.06125547, 1241.05033355, 1290.24033922, 1338.91595895, 1387.03077949, 1435.54957065, 1483.50527712, 1531.53675575, 1580.93403872, 1633.44505184, 1691.84777892, 1759.52156378, 1840.69776587, 1924.63869688, 2011.38740572, 2100.94444129, 2193.06792083, 2287.86300784, 2385.03958615, 2484.39053768, 2586.02970245, 2689.3828964 , 2794.38032908, 2901.15980006, 3009.25442166, 3118.62798954, 3229.45518226, 3341.75361128, 3455.42911291])
- guess 72(pressure)float64359.1 361.3 ... 3.342e+03 3.455e+03
- units :
- Kelvin
array([ 359.07429562, 361.25244897, 362.45770106, 363.8329158 , 365.38994954, 367.1445995 , 369.11068792, 371.32644082, 373.80431435, 376.51485398, 379.42878466, 382.57790291, 385.97379484, 389.62106718, 393.54635663, 397.71517614, 402.21784453, 406.96768791, 411.83702742, 416.81841876, 421.91073545, 427.10783762, 432.39865995, 437.76506089, 443.18108477, 448.62635163, 454.09641998, 459.61396405, 465.22461359, 470.98185134, 476.9543204 , 483.20079757, 489.77656617, 496.78592419, 504.58703125, 512.69824486, 521.00252295, 529.61854052, 538.94894345, 549.08363997, 560.21759726, 572.65528073, 586.10810388, 600.2674054 , 615.4933692 , 632.03608248, 649.93135654, 669.30518355, 690.03570024, 712.14214464, 735.92093729, 761.37288101, 788.7437653 , 818.01882082, 849.29803967, 882.67205026, 918.35792179, 956.42591533, 997.25714904, 1041.02743942, 1088.31902247, 1138.70780344, 1190.06125547, 1241.05033355, 1290.24033922, 1338.91595895, 1387.03077949, 1435.54957065, 1483.50527712, 1531.53675575, 1580.93403872, 1633.44505184, 1691.84777892, 1759.52156378, 1840.69776587, 1924.63869688, 2011.38740572, 2100.94444129, 2193.06792083, 2287.86300784, 2385.03958615, 2484.39053768, 2586.02970245, 2689.3828964 , 2794.38032908, 2901.15980006, 3009.25442166, 3118.62798954, 3229.45518226, 3341.75361128, 3455.42911291])
- guess 73(pressure)float64359.2 361.4 ... 3.348e+03 3.462e+03
- units :
- Kelvin
array([ 359.20493416, 361.38473102, 362.5908505 , 363.96711981, 365.52523086, 367.28103623, 369.24823951, 371.46522297, 373.94358743, 376.65304536, 379.56672859, 382.71583391, 386.1118827 , 389.75943068, 393.68518564, 397.8545912 , 402.36063552, 407.11224442, 411.98115987, 416.96244553, 422.05489572, 427.25276095, 432.54460113, 437.91209127, 443.32926223, 448.77586643, 454.2472374 , 459.76596667, 465.3779514 , 471.13671054, 477.11100796, 483.35925772, 489.9364588 , 496.95132295, 504.75072758, 512.8590454 , 521.16133782, 529.77938657, 539.11258245, 549.24966662, 560.38844022, 572.83146805, 586.28585821, 600.44689238, 615.67757685, 632.22463844, 650.12438813, 669.50251725, 690.23756138, 712.35012454, 736.13496555, 761.59300969, 788.97182565, 818.25542896, 849.54358578, 882.92935215, 918.62735572, 956.70787822, 997.55381037, 1041.33887127, 1088.64806505, 1139.04602465, 1190.4086779 , 1241.39008835, 1290.5837299 , 1339.2648425 , 1387.39761383, 1435.9424501 , 1483.94046727, 1532.03632936, 1581.53705911, 1634.24167239, 1692.93197986, 1764.13400987, 1845.47500672, 1929.57375866, 2016.48413806, 2106.18149313, 2198.44879574, 2293.39121918, 2390.67385616, 2490.1343832 , 2591.886257 , 2695.29681633, 2800.35523206, 2907.19903643, 3015.31019922, 3124.70353206, 3235.55410815, 3347.85744192, 3461.53995871])
- guess 74(pressure)float64359.3 361.5 ... 3.349e+03 3.463e+03
- units :
- Kelvin
array([ 359.28676472, 361.4675495 , 362.67431479, 364.05127264, 365.61010538, 367.36664423, 369.3345049 , 371.55221897, 374.03031786, 376.73739469, 379.64986073, 382.79799615, 386.19326378, 389.84025124, 393.76521927, 397.93709618, 402.44481531, 407.19495761, 412.06204223, 417.04192855, 422.13340846, 427.33129437, 432.62357375, 437.9916296 , 443.40945768, 448.85700752, 454.32925433, 459.84867616, 465.46157281, 471.22147142, 477.19730426, 483.44693235, 490.02490409, 497.0465477 , 504.84215863, 512.9445116 , 521.24128866, 529.86020302, 539.19529724, 549.33334864, 560.47710766, 572.92564622, 586.37914911, 600.53933 , 615.77398672, 632.32411584, 650.22688868, 669.60750719, 690.34510143, 712.46242784, 736.25145745, 761.71330286, 789.09821709, 818.38819226, 849.68261631, 883.07804919, 918.78534552, 956.87491256, 997.73210033, 1041.52775416, 1088.85047082, 1139.24967208, 1190.61316642, 1241.56883834, 1290.74967443, 1339.41184741, 1387.53876797, 1436.07482725, 1484.07570693, 1532.18481652, 1581.71722896, 1634.49633218, 1693.32126963, 1765.23025685, 1846.61040246, 1930.74664076, 2017.69542071, 2107.42609228, 2199.72754396, 2294.70495124, 2392.01274755, 2491.49927099, 2593.27788679, 2696.70202027, 2801.77487288, 2908.63391286, 3016.74894418, 3126.14691559, 3237.00299362, 3349.30743709, 3462.99156832])
- guess 75(pressure)float64359.3 361.5 ... 3.349e+03 3.463e+03
- units :
- Kelvin
array([ 359.28676472, 361.4675495 , 362.67431479, 364.05127264, 365.61010538, 367.36664423, 369.3345049 , 371.55221897, 374.03031786, 376.73739469, 379.64986073, 382.79799615, 386.19326378, 389.84025124, 393.76521927, 397.93709618, 402.44481531, 407.19495761, 412.06204223, 417.04192855, 422.13340846, 427.33129437, 432.62357375, 437.9916296 , 443.40945768, 448.85700752, 454.32925433, 459.84867616, 465.46157281, 471.22147142, 477.19730426, 483.44693235, 490.02490409, 497.0465477 , 504.84215863, 512.9445116 , 521.24128866, 529.86020302, 539.19529724, 549.33334864, 560.47710766, 572.92564622, 586.37914911, 600.53933 , 615.77398672, 632.32411584, 650.22688868, 669.60750719, 690.34510143, 712.46242784, 736.25145745, 761.71330286, 789.09821709, 818.38819226, 849.68261631, 883.07804919, 918.78534552, 956.87491256, 997.73210033, 1041.52775416, 1088.85047082, 1139.24967208, 1190.61316642, 1241.56883834, 1290.74967443, 1339.41184741, 1387.53876797, 1436.07482725, 1484.07570693, 1532.18481652, 1581.71722896, 1634.49633218, 1693.32126963, 1765.23025685, 1846.61040246, 1930.74664076, 2017.69542071, 2107.42609228, 2199.72754396, 2294.70495124, 2392.01274755, 2491.49927099, 2593.27788679, 2696.70202027, 2801.77487288, 2908.63391286, 3016.74894418, 3126.14691559, 3237.00299362, 3349.30743709, 3462.99156832])
- guess 76(pressure)float64359.3 361.5 ... 3.35e+03 3.463e+03
- units :
- Kelvin
array([ 359.31787725, 361.4991235 , 362.70611173, 364.08335063, 365.64247984, 367.39926699, 369.36732838, 371.58527654, 374.06292936, 376.76823255, 379.67967074, 382.82694961, 386.22148886, 389.86791532, 393.79213584, 397.9658084 , 402.47389978, 407.22236534, 412.08812981, 417.0670117 , 422.15781133, 427.3556131 , 432.64805229, 438.01632919, 443.4344157 , 448.88236704, 454.35495985, 459.8746147 , 465.48785505, 471.24820925, 477.2247001 , 483.47488331, 490.05305982, 497.07830245, 504.87132267, 512.96993274, 521.26354591, 529.88272335, 539.21860392, 549.35692865, 560.5029878 , 572.95409563, 586.40670512, 600.56602616, 615.8023386 , 632.35362761, 650.25750914, 669.63891886, 690.37730122, 712.49657576, 736.28720985, 761.75039818, 789.13787535, 818.43052993, 849.72752267, 883.12743219, 918.83898907, 956.93264029, 997.79521502, 1041.595879 , 1088.92542898, 1139.3238531 , 1190.68612789, 1241.62518638, 1290.7971703 , 1339.44853686, 1387.57131835, 1436.10288339, 1484.10394874, 1532.21652779, 1581.75742319, 1634.55649026, 1693.41574168, 1765.50099204, 1846.89080496, 1931.03629973, 2017.99456188, 2107.7334595 , 2200.04334283, 2295.02938791, 2392.3433949 , 2491.83633579, 2593.62155325, 2697.04903553, 2802.12545019, 2908.98824961, 3017.10423262, 3126.50334611, 3237.36077964, 3349.66549385, 3463.35002068])
- flux_emission(wavelength)float646.807e+04 3.997e+05 ... 1.398e+03
- units :
- erg/cm**2/s/cm
array([6.80668108e+04, 3.99662941e+05, 1.50894456e+06, 4.14887736e+06, 1.38146187e+07, 3.44503980e+07, 6.63202638e+07, 1.36835909e+08, 2.72633362e+08, 4.63819847e+08, 6.85754389e+08, 8.99421240e+08, 1.15792930e+09, 1.48914500e+09, 2.06288182e+09, 2.68498283e+09, 3.39403808e+09, 4.43457207e+09, 5.51258870e+09, 4.33140491e+09, 8.17590988e+09, 9.26182956e+09, 8.72302406e+09, 8.61480277e+09, 7.81005503e+09, 6.55145226e+09, 5.88893155e+09, 5.68375359e+09, 4.97004308e+09, 5.98851057e+09, 6.28422718e+09, 8.39441148e+09, 9.73263113e+09, 9.16491786e+09, 8.80390022e+09, 1.33447724e+10, 1.35040406e+10, 1.26776835e+10, 1.39601389e+10, 1.70057846e+10, 2.39095467e+10, 2.90497333e+10, 3.39411277e+10, 4.55296210e+10, 5.55678502e+10, 5.63083575e+10, 5.82710291e+10, 6.57083069e+10, 6.93097815e+10, 6.65443506e+10, 7.37145413e+10, 7.72550414e+10, 8.19935699e+10, 9.08970534e+10, 1.00490414e+11, 1.10676305e+11, 9.08309473e+10, 7.23421701e+10, 5.22337435e+10, 3.63164223e+10, 2.11819005e+10, 1.34024501e+10, 9.91081893e+09, 8.59840860e+09, 9.07879770e+09, 1.21986577e+10, 8.26955674e+09, 8.10204339e+09, 1.13051028e+10, 1.91859424e+10, 2.75567421e+10, 3.82032501e+10, 4.21736964e+10, 4.07650412e+10, 3.42187964e+10, 2.46274115e+10, 1.96847630e+10, 2.15913747e+10, 1.65491048e+10, 2.38232363e+10, ... 1.49946884e+11, 3.73376607e+11, 5.50689169e+11, 5.13280031e+11, 4.31726881e+11, 3.25053777e+11, 2.38269403e+11, 1.66099965e+11, 1.09021720e+11, 8.39300148e+10, 6.50117577e+10, 3.05561305e+10, 1.96747726e+10, 2.04590127e+10, 2.06643874e+10, 2.25654303e+10, 1.66414752e+10, 7.47625966e+10, 6.71576300e+11, 1.01785254e+12, 1.30512901e+12, 1.24518692e+12, 1.11537121e+12, 9.60846481e+11, 6.45275064e+11, 3.57230076e+11, 1.53411501e+11, 1.75079365e+11, 1.83146668e+11, 3.84415600e+11, 7.75415711e+11, 7.21532493e+11, 6.14522673e+11, 4.44371681e+11, 3.39520040e+11, 2.97356932e+11, 2.07645112e+11, 1.73582967e+11, 9.86237950e+10, 1.94042269e+11, 1.54200648e+11, 1.08254253e+11, 9.49671893e+10, 6.61706903e+10, 4.34197418e+10, 2.52625274e+10, 1.50370477e+10, 7.76506875e+09, 1.62199783e+09, 1.90747148e+08, 2.87441330e+07, 3.92646194e+08, 1.04072260e+09, 1.31225240e+09, 1.12001997e+09, 6.16221352e+08, 2.53889460e+08, 6.89385823e+07, 2.24493006e+07, 7.35442699e+06, 2.71798708e+06, 8.82380135e+05, 1.21247103e+05, 2.27477282e+05, 3.23715929e+05, 3.99282323e+05, 6.58966872e+05, 1.24172729e+06, 8.94402020e+08, 3.15816065e+09, 3.33926667e+09, 1.59752406e+09, 7.78830171e+08, 1.25886949e+08, 1.84695885e+07, 4.88647687e+06, 7.24937654e+04, 6.61583715e+04, 1.55648736e+04, 1.39817290e+03])
- e-(pressure)float644.5e-38 4.5e-38 ... 2.532e-07
- units :
- v/v
array([4.50000000e-38, 4.50000000e-38, 4.50000000e-38, 4.50000000e-38, 4.50000000e-38, 4.50000000e-38, 4.50000000e-38, 4.50000000e-38, 4.50000000e-38, 4.50000000e-38, 4.50000000e-38, 4.50000000e-38, 4.50000000e-38, 4.50000000e-38, 4.50000000e-38, 4.50000000e-38, 4.50000000e-38, 4.50000000e-38, 4.50000000e-38, 4.50000000e-38, 4.50000000e-38, 4.50000000e-38, 4.50000000e-38, 4.50000000e-38, 4.50000000e-38, 4.50000000e-38, 4.50000000e-38, 4.50000000e-38, 4.50000000e-38, 4.50000000e-38, 8.17802917e-38, 3.83982353e-37, 1.62359223e-36, 6.28247232e-36, 2.70002813e-35, 1.25776554e-34, 5.85755859e-34, 2.49308504e-33, 1.06944259e-32, 5.09601886e-32, 2.63205162e-31, 1.52734398e-30, 1.07677377e-29, 7.56624812e-29, 5.81728007e-28, 4.84052773e-27, 4.30014334e-26, 4.30348896e-25, 4.29665093e-24, 4.99918613e-23, 6.60857319e-22, 8.32485966e-21, 1.11170558e-19, 1.06640618e-18, 8.10839688e-18, 5.00144541e-17, 2.77548343e-16, 1.34708732e-15, 5.61351975e-15, 1.78899071e-14, 5.71791392e-14, 1.67024011e-13, 4.44460567e-13, 1.07313862e-12, 2.33141320e-12, 4.74188027e-12, 9.06345249e-12, 1.66327031e-11, 2.89649655e-11, 4.84052737e-11, 7.93320700e-11, 1.29750053e-10, 2.17453701e-10, 3.94521978e-10, 7.32320941e-10, 1.30701049e-09, 2.25397960e-09, 3.76384181e-09, 6.08983995e-09, 9.57179977e-09, 1.46128265e-08, 2.16684304e-08, 3.12449847e-08, 4.38476739e-08, 6.00234557e-08, 8.03610488e-08, 1.05266422e-07, 1.35209842e-07, 1.69380453e-07, 2.08676955e-07, 2.53208491e-07])
- H2(pressure)float640.8362 0.8362 ... 0.819 0.8153
- units :
- v/v
array([0.83619389, 0.83619158, 0.83618918, 0.8361872 , 0.83618571, 0.83618479, 0.83618452, 0.83618352, 0.83618209, 0.83617997, 0.83617765, 0.83617581, 0.83617448, 0.83617369, 0.83617242, 0.83617096, 0.83616946, 0.83616801, 0.83616718, 0.83616696, 0.83616719, 0.83616706, 0.83616717, 0.83616723, 0.83616723, 0.83616714, 0.83616714, 0.83616756, 0.83616736, 0.83616717, 0.83616674, 0.83616644, 0.83616706, 0.83616869, 0.83616996, 0.83617083, 0.83617165, 0.83617097, 0.83617092, 0.83617332, 0.83617518, 0.83617588, 0.83617674, 0.83617761, 0.83617989, 0.83618442, 0.83619112, 0.83618871, 0.83618568, 0.83618698, 0.8361915 , 0.8361939 , 0.83619248, 0.83619448, 0.83619954, 0.83619803, 0.83619647, 0.83619634, 0.83619978, 0.83621723, 0.83621996, 0.83626472, 0.83630445, 0.83635997, 0.83639513, 0.83643137, 0.83646671, 0.83650602, 0.83654259, 0.83655722, 0.83657 , 0.83656914, 0.83657577, 0.8365597 , 0.83649726, 0.83639837, 0.83628711, 0.83613959, 0.83591685, 0.8355865 , 0.8351479 , 0.83454375, 0.83367126, 0.83255477, 0.83116156, 0.82946304, 0.8275073 , 0.8253742 , 0.82240483, 0.81903859, 0.81534601])
- H(pressure)float647.189e-28 1.026e-27 ... 0.02197
- units :
- v/v
array([7.18884791e-28, 1.02567594e-27, 1.19897068e-27, 1.44732355e-27, 1.80698869e-27, 2.33842333e-27, 3.14153947e-27, 4.40825774e-27, 6.46202584e-27, 9.82109704e-27, 1.53899785e-26, 2.49556224e-26, 4.18801316e-26, 7.26469309e-26, 1.30546390e-25, 2.41330431e-25, 4.64325454e-25, 9.13131247e-25, 1.79688900e-24, 3.53363032e-24, 6.93902639e-24, 1.35928418e-23, 2.65043467e-23, 5.12786928e-23, 9.80873645e-23, 1.85049851e-22, 3.44346894e-22, 6.33847482e-22, 1.16077809e-21, 2.12787994e-21, 3.93399253e-21, 7.37459686e-21, 1.40723364e-20, 2.76284279e-20, 5.75096738e-20, 1.20422419e-19, 2.50483499e-19, 5.23837501e-19, 1.13912986e-18, 2.58060325e-18, 6.16290024e-18, 1.57536207e-17, 4.16639368e-17, 1.10692749e-16, 3.02247261e-16, 8.54295548e-16, 2.48189617e-15, 7.40953513e-15, 2.23260436e-14, 6.75292562e-14, 2.06483524e-13, 6.32661815e-13, 1.94851707e-12, 5.97461601e-12, 1.81601642e-11, 5.45620827e-11, 1.61902929e-10, 4.72197899e-10, 1.35957357e-09, 3.85472666e-09, 1.08095252e-08, 2.94872786e-08, 7.47398280e-08, 1.73195698e-07, 3.62776755e-07, 7.10654901e-07, 1.31079974e-06, 2.32205913e-06, 3.91627389e-06, 6.37115096e-06, 1.01698307e-05, 1.62363541e-05, 2.65322692e-05, 4.69015571e-05, 8.48788803e-05, 1.48128616e-04, 2.49869810e-04, 4.08060793e-04, 6.45535464e-04, 9.91749862e-04, 1.47979008e-03, 2.14723093e-03, 3.03744051e-03, 4.18580778e-03, 5.63178712e-03, 7.41477689e-03, 9.55354974e-03, 1.20690518e-02, 1.49749272e-02, 1.82764241e-02, 2.19652731e-02])
- H+(pressure)float644.5e-38 4.5e-38 ... 6.658e-16
- units :
- v/v
array([4.50000000e-38, 4.50000000e-38, 4.50000000e-38, 4.50000000e-38, 4.50000000e-38, 4.50000000e-38, 4.50000000e-38, 4.50000000e-38, 4.50000000e-38, 4.50000000e-38, 4.50000000e-38, 4.50000000e-38, 4.50000000e-38, 4.50000000e-38, 4.50000000e-38, 4.50000000e-38, 4.50000000e-38, 4.50000000e-38, 4.50000000e-38, 4.50000000e-38, 4.50000000e-38, 4.50000000e-38, 4.50000000e-38, 4.50000000e-38, 4.50000000e-38, 4.50000000e-38, 4.50000000e-38, 4.50000000e-38, 4.50000000e-38, 4.50000000e-38, 4.50004677e-38, 4.50017544e-38, 4.50030731e-38, 4.50044433e-38, 4.50052029e-38, 4.50055312e-38, 4.50058569e-38, 4.50058925e-38, 4.50056100e-38, 4.50052056e-38, 4.50054559e-38, 4.50058217e-38, 4.50057355e-38, 4.50055847e-38, 4.50056159e-38, 4.50057548e-38, 4.50059994e-38, 4.50059833e-38, 4.50058502e-38, 4.50057603e-38, 4.50058781e-38, 4.50059037e-38, 4.50058034e-38, 4.50058947e-38, 4.50059998e-38, 4.50053245e-38, 4.50051375e-38, 4.50055086e-38, 4.50056646e-38, 4.50058206e-38, 4.50059766e-38, 4.50058790e-38, 4.50057366e-38, 4.50053352e-38, 4.50050386e-38, 4.50050000e-38, 4.50050000e-38, 4.50050000e-38, 4.50050000e-38, 4.86484299e-38, 5.22676999e-38, 3.65876511e-37, 7.36385518e-36, 2.92049357e-34, 1.37887848e-32, 5.25471029e-31, 1.63489678e-29, 4.19125219e-28, 8.88715554e-27, 1.58332949e-25, 2.37088952e-24, 3.01327100e-23, 3.30378105e-22, 3.10709150e-21, 2.53918833e-20, 1.82844980e-19, 1.15958196e-18, 6.54833900e-18, 3.35689946e-17, 1.56370752e-16, 6.65784962e-16])
- H-(pressure)float644.5e-38 4.5e-38 ... 3.699e-08
- units :
- v/v
array([4.50000000e-38, 4.50000000e-38, 4.50000000e-38, 4.50000000e-38, 4.50000000e-38, 4.50000000e-38, 4.50000000e-38, 4.50000000e-38, 4.50000000e-38, 4.50000000e-38, 4.50000000e-38, 4.50000000e-38, 4.50000000e-38, 4.50000000e-38, 4.50000000e-38, 4.50000000e-38, 4.50000000e-38, 4.50000000e-38, 4.50000000e-38, 4.50000000e-38, 4.50000000e-38, 4.50000000e-38, 4.50000000e-38, 4.50000000e-38, 4.50000000e-38, 4.50000000e-38, 4.50000000e-38, 4.50000000e-38, 4.50000000e-38, 4.50000000e-38, 4.50004677e-38, 4.50017544e-38, 4.50030731e-38, 4.50044433e-38, 4.50052029e-38, 4.50055312e-38, 4.50058569e-38, 4.50058925e-38, 4.50056100e-38, 4.50052056e-38, 4.50054559e-38, 4.50058217e-38, 4.50057355e-38, 4.72226954e-38, 1.55292988e-37, 4.75601411e-37, 1.38070491e-36, 3.07696449e-35, 6.87936150e-34, 1.79150036e-32, 5.32355593e-31, 1.50592182e-29, 4.51751283e-28, 9.67847744e-27, 1.63197437e-25, 2.20635308e-24, 2.65711124e-23, 2.76172460e-22, 2.43650262e-21, 1.61868493e-20, 1.07128551e-19, 6.33387715e-19, 3.26567919e-18, 1.44299789e-17, 5.41455811e-17, 1.82301990e-16, 5.57334786e-16, 1.59356247e-15, 4.20418583e-15, 1.04048977e-14, 2.49592593e-14, 5.95797083e-14, 1.47291348e-13, 4.08750624e-13, 1.17032701e-12, 3.14908361e-12, 8.01401467e-12, 1.93482103e-11, 4.43743843e-11, 9.70527106e-11, 2.02532021e-10, 4.03656601e-10, 7.70414928e-10, 1.40916472e-09, 2.47918194e-09, 4.21137598e-09, 6.91356473e-09, 1.10047891e-08, 1.69074454e-08, 2.53088289e-08, 3.69891433e-08])
- H2-(pressure)float644.5e-38 4.5e-38 ... 4.568e-10
- units :
- v/v
array([4.50000000e-38, 4.50000000e-38, 4.50000000e-38, 4.50000000e-38, 4.50000000e-38, 4.50000000e-38, 4.50000000e-38, 4.50000000e-38, 4.50000000e-38, 4.50000000e-38, 4.50000000e-38, 4.50000000e-38, 4.50000000e-38, 4.50000000e-38, 4.50000000e-38, 4.50000000e-38, 4.50000000e-38, 4.50000000e-38, 4.50000000e-38, 4.50000000e-38, 4.50000000e-38, 4.50000000e-38, 4.50000000e-38, 4.50000000e-38, 4.50000000e-38, 4.50000000e-38, 4.50000000e-38, 4.50000000e-38, 4.50000000e-38, 4.50000000e-38, 4.50004677e-38, 4.50017544e-38, 4.50030731e-38, 4.50044433e-38, 4.50052029e-38, 4.50055312e-38, 4.50058569e-38, 4.50058925e-38, 4.50056100e-38, 4.50052056e-38, 4.50054559e-38, 4.50058217e-38, 4.50057355e-38, 4.50055847e-38, 4.50056159e-38, 4.50057548e-38, 4.50059994e-38, 4.50059833e-38, 4.50058502e-38, 4.50057603e-38, 4.50058781e-38, 1.92341482e-37, 4.58219041e-36, 1.25247370e-34, 3.83272183e-33, 9.34857663e-32, 2.01954805e-30, 3.73571880e-29, 5.82998279e-28, 6.80039917e-27, 7.86514444e-26, 8.02491610e-25, 6.92043434e-24, 4.92168744e-23, 2.84806317e-22, 1.43704667e-21, 6.41873805e-21, 2.63543363e-20, 9.77939014e-20, 3.35236404e-19, 1.10490220e-18, 3.62342707e-18, 1.24256180e-17, 4.94236151e-17, 2.04787295e-16, 7.85281792e-16, 2.80748906e-15, 9.39340095e-15, 2.94562397e-14, 8.70098365e-14, 2.42238650e-13, 6.36632262e-13, 1.58508311e-12, 3.74119099e-12, 8.40739238e-12, 1.80780664e-11, 3.72269420e-11, 7.37235602e-11, 1.39829801e-10, 2.56608101e-10, 4.56818682e-10])
- H2+(pressure)float644.5e-38 4.5e-38 ... 6.835e-16
- units :
- v/v
array([4.50000000e-38, 4.50000000e-38, 4.50000000e-38, 4.50000000e-38, 4.50000000e-38, 4.50000000e-38, 4.50000000e-38, 4.50000000e-38, 4.50000000e-38, 4.50000000e-38, 4.50000000e-38, 4.50000000e-38, 4.50000000e-38, 4.50000000e-38, 4.50000000e-38, 4.50000000e-38, 4.50000000e-38, 4.50000000e-38, 4.50000000e-38, 4.50000000e-38, 4.50000000e-38, 4.50000000e-38, 4.50000000e-38, 4.50000000e-38, 4.50000000e-38, 4.50000000e-38, 4.50000000e-38, 4.50000000e-38, 4.50000000e-38, 4.50000000e-38, 4.50004677e-38, 4.50017544e-38, 4.50030731e-38, 4.50044433e-38, 4.50052029e-38, 4.50055312e-38, 4.50058569e-38, 4.50058925e-38, 4.50056100e-38, 4.50052056e-38, 4.50054559e-38, 4.50058217e-38, 4.50057355e-38, 4.50055847e-38, 4.50056159e-38, 4.50057548e-38, 4.50059994e-38, 4.50059833e-38, 4.50058502e-38, 4.50057603e-38, 4.50058781e-38, 4.50059037e-38, 4.50058034e-38, 4.50058947e-38, 4.50059998e-38, 4.50053245e-38, 4.50051375e-38, 4.50055086e-38, 4.50056646e-38, 4.50058206e-38, 4.50059766e-38, 4.50058790e-38, 4.50057366e-38, 4.50053352e-38, 4.50050386e-38, 4.50050000e-38, 4.50050000e-38, 4.50050000e-38, 4.50050000e-38, 4.72329031e-38, 4.93841693e-38, 3.55206713e-37, 8.00858667e-36, 3.09724332e-34, 1.39162197e-32, 5.08471118e-31, 1.52754842e-29, 3.80695161e-28, 7.89931091e-27, 1.38545998e-25, 2.05490565e-24, 2.60173870e-23, 2.85647746e-22, 2.70452218e-21, 2.23591717e-20, 1.63597425e-19, 1.05892084e-18, 6.12785195e-18, 3.22949708e-17, 1.55156657e-16, 6.83464256e-16])
- H3+(pressure)float644.5e-38 4.5e-38 ... 1.387e-12
- units :
- v/v
array([4.50000000e-38, 4.50000000e-38, 4.50000000e-38, 4.50000000e-38, 4.50000000e-38, 4.50000000e-38, 4.50000000e-38, 4.50000000e-38, 4.50000000e-38, 4.50000000e-38, 4.50000000e-38, 4.50000000e-38, 4.50000000e-38, 4.50000000e-38, 4.50000000e-38, 4.50000000e-38, 4.50000000e-38, 4.50000000e-38, 4.50000000e-38, 4.50000000e-38, 4.50000000e-38, 4.50000000e-38, 4.50000000e-38, 4.50000000e-38, 4.50000000e-38, 4.50000000e-38, 4.50000000e-38, 4.50000000e-38, 4.50000000e-38, 4.50000000e-38, 4.50004677e-38, 4.50017544e-38, 4.50030731e-38, 4.50044433e-38, 4.50052029e-38, 4.50055312e-38, 4.50058569e-38, 4.50058925e-38, 4.50056100e-38, 4.50052056e-38, 4.50054559e-38, 4.50058217e-38, 4.50057355e-38, 4.50055847e-38, 4.50056159e-38, 4.50057548e-38, 4.50059994e-38, 4.50059833e-38, 4.50058502e-38, 4.50057603e-38, 4.50058781e-38, 4.50059037e-38, 4.50058034e-38, 4.50058947e-38, 4.50059998e-38, 4.50053245e-38, 4.50051375e-38, 4.50055086e-38, 4.50056646e-38, 4.50058206e-38, 4.50059766e-38, 5.39272898e-38, 6.78985316e-38, 1.26115723e-36, 2.97531219e-35, 5.53981630e-34, 8.21247463e-33, 1.05350524e-31, 1.12241400e-30, 1.05035307e-29, 9.15481079e-29, 8.04183292e-28, 7.76027213e-27, 1.02861022e-25, 1.50306694e-24, 1.91209430e-23, 2.13023997e-22, 2.08910564e-21, 1.80690052e-20, 1.39227653e-19, 9.55592199e-19, 5.88099617e-18, 3.28161043e-17, 1.65288957e-16, 7.57984491e-16, 3.19339073e-15, 1.23556348e-14, 4.42236717e-14, 1.48554019e-13, 4.67836893e-13, 1.38665843e-12])
- He(pressure)float640.1624 0.1624 ... 0.1607 0.1604
- units :
- v/v
array([0.16242 , 0.16242082, 0.16242148, 0.16242196, 0.16242223, 0.16242226, 0.16242199, 0.16242212, 0.16242261, 0.16242341, 0.1624241 , 0.16242439, 0.16242426, 0.16242365, 0.16242324, 0.16242321, 0.162424 , 0.16242556, 0.16242712, 0.16242868, 0.16243002, 0.16242917, 0.16242779, 0.16242705, 0.16242694, 0.16242745, 0.16242716, 0.16242612, 0.16242544, 0.16242475, 0.16242488, 0.16242644, 0.162428 , 0.16242956, 0.162429 , 0.16242714, 0.1624253 , 0.1624253 , 0.16242672, 0.16242814, 0.16242544, 0.16242187, 0.16242264, 0.16242415, 0.16242384, 0.16242245, 0.16242 , 0.16242041, 0.16242183, 0.1624224 , 0.16242122, 0.16242 , 0.16242 , 0.16242 , 0.16242 , 0.16242 , 0.16242 , 0.16241929, 0.16241679, 0.16241223, 0.16241036, 0.16240028, 0.16239243, 0.16237973, 0.16237123, 0.16236183, 0.16235295, 0.16234345, 0.16233462, 0.16232888, 0.16232239, 0.16231647, 0.16230934, 0.16229053, 0.1622748 , 0.16226564, 0.16225499, 0.16223591, 0.16220765, 0.16217594, 0.16213435, 0.16208205, 0.16200807, 0.16190791, 0.16178364, 0.16163328, 0.16147185, 0.16127881, 0.16102511, 0.16073516, 0.16040853])
- H2O(pressure)float640.0008191 0.0008191 ... 0.0005521
- units :
- v/v
array([0.00081907, 0.00081908, 0.00081908, 0.00081908, 0.00081909, 0.00081909, 0.00081909, 0.00081909, 0.0008191 , 0.0008191 , 0.00081909, 0.00081909, 0.00081909, 0.00081909, 0.00081909, 0.00081909, 0.00081909, 0.00081909, 0.00081909, 0.00081909, 0.00081909, 0.00081909, 0.00081909, 0.00081909, 0.00081909, 0.00081909, 0.00081909, 0.00081909, 0.00081909, 0.0008191 , 0.0008191 , 0.0008191 , 0.0008191 , 0.00081909, 0.00081909, 0.0008191 , 0.0008191 , 0.0008191 , 0.00081909, 0.00081909, 0.00081908, 0.00081909, 0.00081909, 0.00081909, 0.00081908, 0.00081908, 0.00081908, 0.00081908, 0.00081908, 0.00081907, 0.00081906, 0.00081904, 0.00081901, 0.00081892, 0.00081892, 0.00081854, 0.0008176 , 0.00081583, 0.00081254, 0.00079861, 0.00079801, 0.00076493, 0.00073649, 0.00068762, 0.00065804, 0.00062853, 0.0006067 , 0.00057958, 0.00055016, 0.00053263, 0.00051926, 0.00051413, 0.00050239, 0.00049906, 0.00051921, 0.00054656, 0.00054815, 0.00054089, 0.00053242, 0.00052428, 0.0005171 , 0.00051574, 0.00052005, 0.00052214, 0.00052271, 0.00052255, 0.00052222, 0.00052216, 0.00053356, 0.00054363, 0.00055212])
- CH4(pressure)float640.0004653 0.0004653 ... 2.475e-05
- units :
- v/v
array([4.65266106e-04, 4.65267600e-04, 4.65269341e-04, 4.65270837e-04, 4.65272052e-04, 4.65272949e-04, 4.65273486e-04, 4.65274245e-04, 4.65275221e-04, 4.65276341e-04, 4.65277235e-04, 4.65277653e-04, 4.65277561e-04, 4.65276930e-04, 4.65276487e-04, 4.65276411e-04, 4.65276535e-04, 4.65276427e-04, 4.65275700e-04, 4.65274362e-04, 4.65272788e-04, 4.65274605e-04, 4.65277249e-04, 4.65278667e-04, 4.65278889e-04, 4.65277956e-04, 4.65276938e-04, 4.65276444e-04, 4.65277850e-04, 4.65278552e-04, 4.65278853e-04, 4.65278365e-04, 4.65276168e-04, 4.65272130e-04, 4.65273684e-04, 4.65277193e-04, 4.65278853e-04, 4.65278442e-04, 4.65275639e-04, 4.65270397e-04, 4.65273938e-04, 4.65281556e-04, 4.65280618e-04, 4.65278233e-04, 4.65276268e-04, 4.65273124e-04, 4.65268821e-04, 4.65266538e-04, 4.65263971e-04, 4.65259700e-04, 4.65256804e-04, 4.65235052e-04, 4.65209342e-04, 4.65125309e-04, 4.65126302e-04, 4.64744710e-04, 4.63802841e-04, 4.62022618e-04, 4.58697849e-04, 4.44025753e-04, 4.43725641e-04, 4.09327085e-04, 3.80790440e-04, 3.24259080e-04, 2.93201530e-04, 2.54584987e-04, 2.36968565e-04, 2.16797663e-04, 1.89047676e-04, 1.61644513e-04, 1.43228165e-04, 1.26032169e-04, 1.12828643e-04, 9.22678982e-05, 6.82646849e-05, 4.96380006e-05, 3.96418322e-05, 3.32915685e-05, 2.89950590e-05, 2.59562756e-05, 2.38467706e-05, 2.19384411e-05, 2.02445464e-05, 1.94418629e-05, 1.92797978e-05, 1.96157382e-05, 2.04213860e-05, 2.16816981e-05, 2.20761395e-05, 2.30923498e-05, 2.47537638e-05])
- CO(pressure)float641.348e-17 1.459e-17 ... 0.0003995
- units :
- v/v
array([1.34786528e-17, 1.45856922e-17, 1.30455668e-17, 1.20321367e-17, 1.14606903e-17, 1.12971907e-17, 1.15415950e-17, 1.22924543e-17, 1.36507562e-17, 1.57141496e-17, 1.86425986e-17, 2.28550536e-17, 2.89582734e-17, 3.78755814e-17, 5.12477885e-17, 7.12529975e-17, 1.03117750e-16, 1.52624888e-16, 2.26038165e-16, 3.34547120e-16, 4.94460286e-16, 7.30093340e-16, 1.07497836e-15, 1.57085981e-15, 2.27030455e-15, 3.23755095e-15, 4.56554730e-15, 6.37411048e-15, 8.85499718e-15, 1.23133656e-14, 1.72803681e-14, 2.46199125e-14, 3.56907035e-14, 5.31967317e-14, 8.40899027e-14, 1.33839783e-13, 2.11637271e-13, 3.36931349e-13, 5.58016393e-13, 9.62065247e-13, 1.75168527e-12, 3.41103511e-12, 6.88630243e-12, 1.39734996e-11, 2.92494567e-11, 6.33588105e-11, 1.41045112e-10, 3.24340975e-10, 7.52744361e-10, 1.75825001e-09, 4.16037288e-09, 9.88197479e-09, 2.36463866e-08, 5.64347886e-08, 1.33711395e-07, 3.13543529e-07, 7.25381453e-07, 1.64636007e-06, 3.67300433e-06, 7.76924696e-06, 1.70589349e-05, 3.22434758e-05, 5.73074036e-05, 8.24566964e-05, 1.16570738e-04, 1.47357222e-04, 1.89845119e-04, 2.27993967e-04, 2.46680118e-04, 2.56989251e-04, 2.73885699e-04, 2.93889418e-04, 3.23686304e-04, 3.56673835e-04, 3.80092548e-04, 3.87321613e-04, 3.97977303e-04, 4.08405172e-04, 4.18887722e-04, 4.27870754e-04, 4.35349952e-04, 4.35442297e-04, 4.30046935e-04, 4.28288040e-04, 4.28425728e-04, 4.29386438e-04, 4.30596647e-04, 4.31745908e-04, 4.17596734e-04, 4.07196223e-04, 3.99532336e-04])
- NH3(pressure)float641.785e-05 1.895e-05 ... 1.436e-05
- units :
- v/v
array([1.78532944e-05, 1.89491549e-05, 2.09838900e-05, 2.31437738e-05, 2.54266236e-05, 2.78269970e-05, 3.03422692e-05, 3.24076601e-05, 3.42516592e-05, 3.58491948e-05, 3.72490899e-05, 3.86656794e-05, 4.01158973e-05, 4.16292697e-05, 4.23742386e-05, 4.28754913e-05, 4.30460580e-05, 4.28672343e-05, 4.30802754e-05, 4.37010300e-05, 4.44805654e-05, 4.38801260e-05, 4.32323098e-05, 4.31757413e-05, 4.37251435e-05, 4.49080966e-05, 4.57354817e-05, 4.64729874e-05, 4.61733808e-05, 4.65662452e-05, 4.71797663e-05, 4.74740057e-05, 4.80867406e-05, 4.89628481e-05, 4.77104181e-05, 4.62231839e-05, 4.56042420e-05, 4.50861354e-05, 4.43411451e-05, 4.38230717e-05, 4.24326615e-05, 3.99842664e-05, 3.71246622e-05, 3.48849455e-05, 3.22556019e-05, 2.99280265e-05, 2.79052197e-05, 2.52018013e-05, 2.26329313e-05, 2.03761563e-05, 1.83068681e-05, 1.64658139e-05, 1.47506675e-05, 1.32008061e-05, 1.17995999e-05, 1.04813137e-05, 9.36056535e-06, 8.39599851e-06, 7.54634893e-06, 6.78971412e-06, 6.13427442e-06, 5.54717365e-06, 5.11305808e-06, 4.81639778e-06, 4.65538295e-06, 4.57339781e-06, 4.56232966e-06, 4.59681266e-06, 4.67694573e-06, 4.80280048e-06, 4.95958149e-06, 5.12486522e-06, 5.26922985e-06, 5.31818165e-06, 5.33168821e-06, 5.38792671e-06, 5.49872244e-06, 5.66286921e-06, 5.88306134e-06, 6.16090527e-06, 6.50253024e-06, 6.89846310e-06, 7.34451636e-06, 7.87457558e-06, 8.49543889e-06, 9.21556871e-06, 1.00501652e-05, 1.10141623e-05, 1.19889933e-05, 1.30989571e-05, 1.43619896e-05])
- N2(pressure)float645.849e-05 5.662e-05 ... 5.57e-05
- units :
- v/v
array([5.84915025e-05, 5.66216428e-05, 5.47289085e-05, 5.32112416e-05, 5.20886351e-05, 5.13899986e-05, 5.11521715e-05, 4.97828800e-05, 4.83492085e-05, 4.68441347e-05, 4.53673540e-05, 4.45121026e-05, 4.42892417e-05, 4.47289349e-05, 4.41403166e-05, 4.35789197e-05, 4.31810440e-05, 4.25650183e-05, 4.27418593e-05, 4.37163142e-05, 4.49870087e-05, 4.35018080e-05, 4.19422077e-05, 4.14060549e-05, 4.18205215e-05, 4.31758277e-05, 4.36552079e-05, 4.37510865e-05, 4.18243945e-05, 4.12140415e-05, 4.11857416e-05, 4.09176636e-05, 4.15252702e-05, 4.31380120e-05, 4.22189510e-05, 4.10017344e-05, 4.11690319e-05, 4.16739007e-05, 4.25532215e-05, 4.47137910e-05, 4.63367667e-05, 4.69409173e-05, 4.70288776e-05, 4.83940512e-05, 4.90465441e-05, 5.08725604e-05, 5.39840136e-05, 5.47571197e-05, 5.51644450e-05, 5.61181987e-05, 5.72868772e-05, 5.87936597e-05, 6.01655794e-05, 6.14720998e-05, 6.24883246e-05, 6.25884703e-05, 6.30834277e-05, 6.37157024e-05, 6.43451633e-05, 6.47874636e-05, 6.54287301e-05, 6.54137730e-05, 6.55354887e-05, 6.56260451e-05, 6.57956997e-05, 6.59073096e-05, 6.60561173e-05, 6.61679656e-05, 6.59375861e-05, 6.57150244e-05, 6.55862123e-05, 6.55179679e-05, 6.55307027e-05, 6.56065910e-05, 6.55121122e-05, 6.52013547e-05, 6.49832517e-05, 6.48228168e-05, 6.47000240e-05, 6.45992601e-05, 6.45157819e-05, 6.41677590e-05, 6.34636690e-05, 6.28290350e-05, 6.22419039e-05, 6.16845603e-05, 6.11469399e-05, 6.06183216e-05, 5.89129535e-05, 5.72756486e-05, 5.57007228e-05])
- PH3(pressure)float647.995e-29 1.345e-28 ... 4.262e-08
- units :
- v/v
array([7.99453834e-29, 1.34468032e-28, 1.89477019e-28, 2.74707534e-28, 4.10351606e-28, 6.32774063e-28, 1.00865445e-27, 1.67156402e-27, 2.88036342e-27, 5.11824733e-27, 9.33645298e-27, 1.75512189e-26, 3.40049640e-26, 6.78273996e-26, 1.39585916e-25, 2.94578372e-25, 6.42788729e-25, 1.42742662e-24, 3.17121562e-24, 7.04024120e-24, 1.56087202e-23, 3.44817330e-23, 7.57135072e-23, 1.65113886e-22, 3.56501080e-22, 7.60471394e-22, 1.59861290e-21, 3.32668921e-21, 6.89219432e-21, 1.42908687e-20, 2.98105123e-20, 6.27908099e-20, 1.34303755e-19, 2.94408233e-19, 6.77335276e-19, 1.56428874e-18, 3.59208796e-18, 8.26930161e-18, 1.96500303e-17, 4.83550223e-17, 1.23974947e-16, 3.36681629e-16, 9.37454754e-16, 2.61771855e-15, 7.43129450e-15, 2.17169351e-14, 6.49362181e-14, 1.96764066e-13, 6.00806959e-13, 1.83086915e-12, 5.60634762e-12, 1.71279476e-11, 5.22952485e-11, 1.58274287e-10, 4.74041031e-10, 1.39613079e-09, 4.05532443e-09, 1.15545212e-08, 3.22934577e-08, 8.53724507e-08, 2.15467539e-07, 3.47727532e-07, 4.59725317e-07, 4.59064383e-07, 4.42438129e-07, 4.19472549e-07, 4.02586466e-07, 3.82737873e-07, 3.61998276e-07, 3.38877873e-07, 3.18297677e-07, 2.96423973e-07, 2.75114034e-07, 2.47019237e-07, 2.17511439e-07, 1.89750268e-07, 1.65773265e-07, 1.45219371e-07, 1.27778051e-07, 1.12931719e-07, 1.00633456e-07, 8.98691092e-08, 7.99823014e-08, 7.18412255e-08, 6.51536377e-08, 5.96794373e-08, 5.53417858e-08, 5.19927806e-08, 4.81861876e-08, 4.50791234e-08, 4.26193816e-08])
- H2S(pressure)float642.539e-05 2.539e-05 ... 1.883e-05
- units :
- v/v
array([2.53916106e-05, 2.53916785e-05, 2.53917861e-05, 2.53918874e-05, 2.53919819e-05, 2.53920693e-05, 2.53921491e-05, 2.53922123e-05, 2.53922610e-05, 2.53922932e-05, 2.53923135e-05, 2.53923259e-05, 2.53923305e-05, 2.53923276e-05, 2.53923243e-05, 2.53923205e-05, 2.53922953e-05, 2.53922544e-05, 2.53922132e-05, 2.53921723e-05, 2.53921295e-05, 2.53921644e-05, 2.53923068e-05, 2.53924491e-05, 2.53925915e-05, 2.53927338e-05, 2.53926938e-05, 2.53926121e-05, 2.53925439e-05, 2.53924753e-05, 2.53933823e-05, 2.53957242e-05, 2.53977161e-05, 2.53993708e-05, 2.54013559e-05, 2.54033695e-05, 2.54050848e-05, 2.54069755e-05, 2.54092828e-05, 2.54117337e-05, 2.54146666e-05, 2.54178313e-05, 2.54206037e-05, 2.54234152e-05, 2.54254148e-05, 2.54277013e-05, 2.54302452e-05, 2.54315545e-05, 2.54330266e-05, 2.54340236e-05, 2.54346176e-05, 2.54353433e-05, 2.54359903e-05, 2.54399353e-05, 2.54437182e-05, 2.54800447e-05, 2.55973746e-05, 2.58635103e-05, 2.65046700e-05, 2.67585949e-05, 2.71479918e-05, 2.72539561e-05, 2.72520049e-05, 2.72474858e-05, 2.72440805e-05, 2.72376062e-05, 2.72320224e-05, 2.72180109e-05, 2.72050515e-05, 2.71332505e-05, 2.70604295e-05, 2.66264088e-05, 2.62093437e-05, 2.52517089e-05, 2.42837068e-05, 2.37932743e-05, 2.36711740e-05, 2.36071478e-05, 2.34895408e-05, 2.33145768e-05, 2.31033025e-05, 2.28561493e-05, 2.25360156e-05, 2.21571393e-05, 2.17340722e-05, 2.12862070e-05, 2.08473783e-05, 2.04435217e-05, 1.99024570e-05, 1.93562147e-05, 1.88268534e-05])
- TiO(pressure)float644.5e-38 4.5e-38 ... 4.258e-08
- units :
- v/v
array([4.50000000e-38, 4.50000000e-38, 4.50000000e-38, 4.50000000e-38, 4.50000000e-38, 4.50000000e-38, 4.50000000e-38, 4.50000000e-38, 4.50000000e-38, 4.50000000e-38, 4.50000000e-38, 4.50000000e-38, 4.50000000e-38, 4.50000000e-38, 4.50000000e-38, 4.50000000e-38, 4.50000000e-38, 4.50000000e-38, 4.50000000e-38, 4.50000000e-38, 4.50000000e-38, 4.50000000e-38, 4.50000000e-38, 4.50000000e-38, 4.50000000e-38, 4.50000000e-38, 4.50000000e-38, 4.50000000e-38, 4.50000000e-38, 4.50000000e-38, 4.50000000e-38, 4.50000000e-38, 4.50000000e-38, 4.50000000e-38, 4.50000000e-38, 4.50000000e-38, 4.50000000e-38, 4.50000000e-38, 4.50000000e-38, 4.50000000e-38, 4.50000000e-38, 4.50000000e-38, 4.50000000e-38, 4.50000000e-38, 4.50000000e-38, 4.50000000e-38, 4.50000337e-38, 4.50024477e-38, 4.50047639e-38, 1.00995859e-37, 3.83857113e-37, 3.96275644e-36, 1.60785553e-34, 6.38213621e-33, 2.45172214e-31, 8.97045327e-30, 3.13603526e-28, 1.03055648e-26, 3.22467718e-25, 9.55233640e-24, 2.69734604e-22, 7.08416074e-21, 1.46669857e-19, 2.31335676e-18, 2.60841389e-17, 2.36389643e-16, 1.76015564e-15, 1.14614117e-14, 6.43958484e-14, 2.99002443e-13, 1.27485936e-12, 5.34415844e-12, 2.38968701e-11, 1.31193946e-10, 7.45529355e-10, 3.75812606e-09, 1.74400615e-08, 6.87845528e-08, 1.44983547e-07, 1.40413576e-07, 1.34074151e-07, 1.26227383e-07, 1.17241815e-07, 1.07163056e-07, 9.63719711e-08, 8.53412347e-08, 7.46866478e-08, 6.47739498e-08, 5.65351542e-08, 4.91414698e-08, 4.25794307e-08])
- VO(pressure)float644.5e-38 4.5e-38 ... 3.798e-10
- units :
- v/v
array([4.50000000e-38, 4.50000000e-38, 4.50000000e-38, 4.50000000e-38, 4.50000000e-38, 4.50000000e-38, 4.50000000e-38, 4.50000000e-38, 4.50000000e-38, 4.50000000e-38, 4.50000000e-38, 4.50000000e-38, 4.50000000e-38, 4.50000000e-38, 4.50000000e-38, 4.50000000e-38, 4.50000000e-38, 4.50000000e-38, 4.50000000e-38, 4.50000000e-38, 4.50000000e-38, 4.50000000e-38, 4.50000000e-38, 4.50000000e-38, 4.50000000e-38, 4.50000000e-38, 4.50000000e-38, 4.50000000e-38, 4.50000000e-38, 4.50000000e-38, 4.50000000e-38, 4.50000000e-38, 4.50000000e-38, 4.50000000e-38, 4.50000000e-38, 4.50000000e-38, 4.50000000e-38, 4.50000000e-38, 4.50000000e-38, 4.50000000e-38, 4.50000000e-38, 4.50000000e-38, 4.50000000e-38, 4.50000000e-38, 4.50000000e-38, 4.50000000e-38, 4.66539449e-38, 5.61323683e-37, 5.99056716e-36, 9.81846243e-35, 2.51002084e-33, 6.42050203e-32, 1.65602568e-30, 4.18814177e-29, 1.02887477e-27, 2.42029179e-26, 5.46752932e-25, 1.16910293e-23, 2.39306338e-22, 4.65684031e-21, 8.71032083e-20, 1.51810067e-18, 2.14636654e-17, 2.37555511e-16, 1.96288397e-15, 1.33743797e-14, 7.67503061e-14, 3.93458154e-13, 1.76648610e-12, 7.07932974e-12, 2.67792606e-11, 9.99949087e-11, 3.98752947e-10, 1.87111088e-09, 4.83545382e-09, 6.42073137e-09, 5.54108599e-09, 4.56255258e-09, 3.65370342e-09, 2.88430884e-09, 2.27368849e-09, 1.81432995e-09, 1.47074386e-09, 1.19824212e-09, 9.82545456e-10, 8.11837622e-10, 6.77280648e-10, 5.70614397e-10, 4.95476485e-10, 4.32720459e-10, 3.79804153e-10])
- Fe(pressure)float644.5e-38 4.5e-38 ... 4.872e-05
- units :
- v/v
array([4.50000000e-38, 4.50000000e-38, 4.50000000e-38, 4.50000000e-38, 4.50000000e-38, 4.50000000e-38, 4.50000000e-38, 4.50000000e-38, 4.50000000e-38, 4.50000000e-38, 4.50000000e-38, 4.50000000e-38, 4.50000000e-38, 4.50000000e-38, 4.50000000e-38, 4.50000000e-38, 4.50000000e-38, 4.50000000e-38, 4.50000000e-38, 4.50000000e-38, 4.50000000e-38, 4.50000000e-38, 4.50000000e-38, 4.50000000e-38, 4.50000000e-38, 4.50000000e-38, 4.50000000e-38, 4.50000000e-38, 4.50000000e-38, 4.50000000e-38, 9.99775113e-38, 8.46418076e-37, 6.91448361e-36, 5.60277598e-35, 3.02526663e-34, 1.21364902e-33, 4.80779665e-33, 1.92239226e-32, 8.26964574e-32, 3.84346427e-31, 1.97004273e-30, 1.14801681e-29, 7.12063408e-29, 4.45247290e-28, 2.92150369e-27, 2.04578463e-26, 1.50848714e-25, 1.16435812e-24, 9.14457222e-24, 7.20934988e-23, 5.78498231e-22, 4.64617901e-21, 3.75435733e-20, 2.99541121e-19, 2.34499160e-18, 1.78294057e-17, 1.31885629e-16, 9.39782726e-16, 6.49446490e-15, 4.28794208e-14, 2.77217796e-13, 1.67842520e-12, 8.85917416e-12, 3.93564054e-11, 1.45521818e-10, 4.74401626e-10, 1.38718997e-09, 3.75647126e-09, 9.30798351e-09, 2.15533129e-08, 4.81713412e-08, 1.07261788e-07, 2.48220610e-07, 6.53881871e-07, 1.74684539e-06, 4.28692930e-06, 9.85458486e-06, 2.08611381e-05, 3.56617396e-05, 5.24065783e-05, 5.34668036e-05, 5.32062969e-05, 5.29042820e-05, 5.25542814e-05, 5.21715173e-05, 5.17567609e-05, 5.13144410e-05, 5.08475968e-05, 5.01763883e-05, 4.94657592e-05, 4.87249639e-05])
- FeH(pressure)float644.5e-38 4.5e-38 ... 5.209e-06
- units :
- v/v
array([4.50000000e-38, 4.50000000e-38, 4.50000000e-38, 4.50000000e-38, 4.50000000e-38, 4.50000000e-38, 4.50000000e-38, 4.50000000e-38, 4.50000000e-38, 4.50000000e-38, 4.50000000e-38, 4.50000000e-38, 4.50000000e-38, 4.50000000e-38, 4.50000000e-38, 4.50000000e-38, 4.50000000e-38, 4.50000000e-38, 4.50000000e-38, 4.50000000e-38, 4.50000000e-38, 4.50000000e-38, 4.50000000e-38, 4.50000000e-38, 4.50000000e-38, 4.50000000e-38, 4.50000000e-38, 4.50000000e-38, 4.50000000e-38, 4.50000000e-38, 4.50004677e-38, 4.50017544e-38, 4.50030731e-38, 4.50044433e-38, 4.50052029e-38, 4.50055312e-38, 4.50058569e-38, 8.57865923e-38, 2.70863706e-37, 8.45995141e-37, 5.22648647e-36, 3.86008398e-35, 3.04929330e-34, 2.42991912e-33, 2.03986080e-32, 1.83682553e-31, 1.74872519e-30, 1.74998467e-29, 1.78432098e-28, 1.82759263e-27, 1.90857513e-26, 1.99599991e-25, 2.10240678e-24, 2.18614087e-23, 2.22824668e-22, 2.20386364e-21, 2.11789693e-20, 1.95692956e-19, 1.75178343e-18, 1.49685386e-17, 1.25039356e-16, 9.76550579e-16, 6.57814334e-15, 3.68441827e-14, 1.69106906e-13, 6.78155822e-13, 2.41676429e-12, 7.93436873e-12, 2.36553342e-11, 6.55876285e-11, 1.75059347e-10, 4.65937216e-10, 1.29457583e-09, 4.15496309e-09, 1.35920443e-08, 4.06384843e-08, 1.13269073e-07, 2.89350624e-07, 5.94102410e-07, 1.04396719e-06, 1.26771599e-06, 1.49456922e-06, 1.75292816e-06, 2.04460420e-06, 2.37285474e-06, 2.74093305e-06, 3.15094321e-06, 3.60646250e-06, 4.09464454e-06, 4.62804899e-06, 5.20923683e-06])
- CrH(pressure)float644.5e-38 4.5e-38 ... 6.421e-08
- units :
- v/v
array([4.50000000e-38, 4.50000000e-38, 4.50000000e-38, 4.50000000e-38, 4.50000000e-38, 4.50000000e-38, 4.50000000e-38, 4.50000000e-38, 4.50000000e-38, 4.50000000e-38, 4.50000000e-38, 4.50000000e-38, 4.50000000e-38, 4.50000000e-38, 4.50000000e-38, 4.50000000e-38, 4.50000000e-38, 4.50000000e-38, 4.50000000e-38, 4.50000000e-38, 4.50000000e-38, 4.50000000e-38, 4.50000000e-38, 4.50000000e-38, 4.50000000e-38, 4.50000000e-38, 4.50000000e-38, 4.50000000e-38, 4.50000000e-38, 4.50000000e-38, 4.50004677e-38, 4.50017544e-38, 4.50030731e-38, 4.50044433e-38, 6.02879963e-38, 9.24371466e-38, 1.33524364e-37, 3.70211034e-37, 1.89352187e-36, 1.05071506e-35, 6.46176143e-35, 4.54889456e-34, 3.41872608e-33, 2.59113771e-32, 2.06549664e-31, 1.76322714e-30, 1.58932350e-29, 1.50312702e-28, 1.44780797e-27, 1.40041513e-26, 1.38023267e-25, 1.36214584e-24, 1.35359366e-23, 1.32838055e-22, 1.27850419e-21, 1.19584571e-20, 1.08814285e-19, 9.53607182e-19, 8.11113785e-18, 3.94862902e-17, 1.83784008e-16, 1.49732165e-15, 1.24047918e-14, 7.94827952e-14, 4.06680333e-13, 1.74266532e-12, 6.55222398e-12, 2.21878188e-11, 6.76382439e-11, 1.89326486e-10, 5.07136404e-10, 1.17902216e-09, 3.00160821e-09, 4.44972958e-09, 5.85046080e-09, 7.17326299e-09, 8.70815187e-09, 1.04785422e-08, 1.25067030e-08, 1.48198366e-08, 1.74415251e-08, 2.03882135e-08, 2.36726122e-08, 2.73201049e-08, 3.13522010e-08, 3.57934657e-08, 4.06607891e-08, 4.59821564e-08, 5.16107122e-08, 5.76792488e-08, 6.42107329e-08])
- Na(pressure)float644.5e-38 4.5e-38 ... 3.052e-06
- units :
- v/v
array([4.50000000e-38, 4.50000000e-38, 4.50000000e-38, 4.50000000e-38, 4.50000000e-38, 4.50000000e-38, 4.50000000e-38, 4.50000000e-38, 4.50000000e-38, 4.50000000e-38, 4.50000000e-38, 4.50000000e-38, 4.50000000e-38, 4.50000000e-38, 4.50000000e-38, 4.50000000e-38, 4.50000000e-38, 4.50000000e-38, 4.50000000e-38, 4.50000000e-38, 4.50000000e-38, 4.50000000e-38, 4.50000000e-38, 4.50000000e-38, 4.50000000e-38, 4.50000000e-38, 4.50000000e-38, 4.50000000e-38, 4.50000000e-38, 4.50000000e-38, 2.97972987e-36, 2.86965591e-31, 3.36510650e-26, 5.67977749e-21, 1.26345096e-18, 3.00920734e-18, 7.10725846e-18, 1.68778320e-17, 4.20522626e-17, 1.10306534e-16, 3.08375212e-16, 9.38703741e-16, 2.97416199e-15, 9.47075727e-15, 3.10696144e-14, 1.06412498e-13, 3.77121707e-13, 1.37342950e-12, 5.05952778e-12, 1.86563914e-11, 6.95129223e-11, 2.58849228e-10, 9.66407643e-10, 3.57276311e-09, 1.30332859e-08, 4.65365195e-08, 1.62839980e-07, 5.48113502e-07, 1.74670066e-06, 2.26042653e-06, 3.15799613e-06, 3.46638696e-06, 3.47192501e-06, 3.48768308e-06, 3.50199860e-06, 3.52737896e-06, 3.55215415e-06, 3.57889924e-06, 3.60416058e-06, 3.62275363e-06, 3.63975001e-06, 3.65164793e-06, 3.66218741e-06, 3.66715910e-06, 3.66716936e-06, 3.66151098e-06, 3.65301318e-06, 3.64195730e-06, 3.62867612e-06, 3.61296934e-06, 3.59512326e-06, 3.57217225e-06, 3.54155805e-06, 3.50680353e-06, 3.46734550e-06, 3.42233203e-06, 3.37205967e-06, 3.31683505e-06, 3.23696578e-06, 3.14830563e-06, 3.05195033e-06])
- K(pressure)float644.5e-38 4.5e-38 ... 1.13e-07
- units :
- v/v
array([4.50000000e-38, 4.50000000e-38, 4.50000000e-38, 4.50000000e-38, 4.50000000e-38, 4.50000000e-38, 4.50000000e-38, 4.50000000e-38, 4.50000000e-38, 4.50000000e-38, 4.50000000e-38, 4.50000000e-38, 4.50000000e-38, 4.50000000e-38, 4.50000000e-38, 4.50000000e-38, 4.50000000e-38, 4.50000000e-38, 4.50000000e-38, 4.50000000e-38, 4.50000000e-38, 4.50000000e-38, 4.50000000e-38, 4.50000000e-38, 4.50000000e-38, 4.50000000e-38, 4.50000000e-38, 4.50000000e-38, 4.50000000e-38, 4.50000000e-38, 6.19125927e-37, 7.62206249e-34, 9.70756947e-31, 1.41272215e-27, 6.32485282e-26, 2.59966392e-25, 1.04539290e-24, 4.32959553e-24, 1.96410079e-23, 9.58110155e-23, 5.44350996e-22, 3.56437529e-21, 2.60887440e-20, 1.92150436e-19, 1.57455996e-18, 1.37799597e-17, 1.26823082e-16, 1.29224436e-15, 1.33964817e-14, 1.40306464e-13, 1.51030426e-12, 1.44977644e-11, 1.39890513e-10, 7.96257115e-10, 3.06252525e-09, 9.76431688e-09, 2.67917623e-08, 6.12603827e-08, 1.10575862e-07, 1.26775816e-07, 1.47963168e-07, 1.58525181e-07, 1.64208923e-07, 1.71218868e-07, 1.77702417e-07, 1.85961093e-07, 1.94128415e-07, 2.02349146e-07, 2.10270424e-07, 2.16301729e-07, 2.21918244e-07, 2.26126837e-07, 2.30040569e-07, 2.32436389e-07, 2.33654497e-07, 2.33491815e-07, 2.32504485e-07, 2.30736359e-07, 2.28121588e-07, 2.24470362e-07, 2.19862195e-07, 2.13836322e-07, 2.05775946e-07, 1.96456170e-07, 1.86027104e-07, 1.74743229e-07, 1.63216415e-07, 1.51867103e-07, 1.38150780e-07, 1.25058938e-07, 1.12982083e-07])
- Rb(pressure)float644.5e-38 4.5e-38 ... 1.653e-10
- units :
- v/v
array([4.50000000e-38, 4.50000000e-38, 4.50000000e-38, 4.50000000e-38, 4.50000000e-38, 4.50000000e-38, 4.50000000e-38, 4.50000000e-38, 4.50000000e-38, 4.50000000e-38, 4.50000000e-38, 4.50000000e-38, 4.50000000e-38, 4.50000000e-38, 4.50000000e-38, 4.50000000e-38, 4.50000000e-38, 4.50000000e-38, 4.50000000e-38, 4.50000000e-38, 4.50000000e-38, 4.50000000e-38, 4.50000000e-38, 4.50000000e-38, 4.50000000e-38, 4.50000000e-38, 4.50000000e-38, 4.50000000e-38, 4.50000000e-38, 4.50000000e-38, 7.39155071e-37, 1.48166324e-33, 3.11015359e-30, 7.60612299e-27, 4.11316889e-25, 1.63132517e-24, 6.33176744e-24, 2.53052654e-23, 1.10588580e-22, 5.18771788e-22, 2.82787865e-21, 1.77135760e-20, 1.23843301e-19, 8.47400292e-19, 3.43067127e-18, 1.62220171e-17, 8.83934478e-17, 3.38261861e-16, 1.30518660e-15, 5.08809960e-15, 2.02336159e-14, 8.05499723e-14, 3.23367430e-13, 1.25988470e-12, 4.83307510e-12, 1.56315021e-11, 4.39097381e-11, 1.03720658e-10, 1.95539976e-10, 2.26592999e-10, 2.68068014e-10, 2.88882895e-10, 2.99872986e-10, 3.14037510e-10, 3.27209313e-10, 3.44449673e-10, 3.61576853e-10, 3.79100408e-10, 3.96226736e-10, 4.09365662e-10, 4.21642410e-10, 4.30776788e-10, 4.39245215e-10, 4.44087621e-10, 4.46045175e-10, 4.44535969e-10, 4.40651985e-10, 4.34386251e-10, 4.25562729e-10, 4.13705171e-10, 3.99377902e-10, 3.81601038e-10, 3.59174358e-10, 3.34744531e-10, 3.09111241e-10, 2.83189344e-10, 2.58325480e-10, 2.35131885e-10, 2.09153471e-10, 1.85808356e-10, 1.65337193e-10])
- Cs(pressure)float644.5e-38 4.5e-38 ... 4.583e-12
- units :
- v/v
array([4.50000000e-38, 4.50000000e-38, 4.50000000e-38, 4.50000000e-38, 4.50000000e-38, 4.50000000e-38, 4.50000000e-38, 4.50000000e-38, 4.50000000e-38, 4.50000000e-38, 4.50000000e-38, 4.50000000e-38, 4.50000000e-38, 4.50000000e-38, 4.50000000e-38, 4.50000000e-38, 4.50000000e-38, 4.50000000e-38, 4.50000000e-38, 4.50000000e-38, 4.50000000e-38, 4.50000000e-38, 4.50000000e-38, 4.50000000e-38, 4.50000000e-38, 4.50000000e-38, 4.50000000e-38, 4.50000000e-38, 4.50000000e-38, 4.50000000e-38, 6.91772276e-37, 1.15563174e-33, 2.01243789e-30, 4.05339506e-27, 1.86454433e-25, 6.70951842e-25, 2.47471518e-24, 7.31890539e-24, 1.96241681e-23, 5.94581934e-23, 1.68370351e-22, 5.08887056e-22, 1.67014921e-21, 5.49607928e-21, 1.97049196e-20, 7.31027247e-20, 2.78146361e-19, 1.15018533e-18, 4.79860447e-18, 2.02316585e-17, 8.70722422e-17, 3.75309654e-16, 1.63218369e-15, 6.91144072e-15, 2.88032466e-14, 1.04220118e-13, 3.41233577e-13, 9.87282632e-13, 2.50006372e-12, 3.37453965e-12, 4.76304153e-12, 5.75932259e-12, 6.51562437e-12, 7.48555817e-12, 8.46460950e-12, 9.80207628e-12, 1.12362856e-11, 1.28860900e-11, 1.46664540e-11, 1.62016209e-11, 1.77506989e-11, 1.89732221e-11, 2.01722955e-11, 2.08626478e-11, 2.11418795e-11, 2.09190416e-11, 2.03629763e-11, 1.95250845e-11, 1.84519712e-11, 1.71735361e-11, 1.58090453e-11, 1.43335591e-11, 1.27515141e-11, 1.12722981e-11, 9.92596724e-12, 8.72702003e-12, 7.69510098e-12, 6.80700784e-12, 5.92571594e-12, 5.19127776e-12, 4.58287888e-12])
- CO2(pressure)float648.148e-17 8.119e-17 ... 3.29e-08
- units :
- v/v
array([8.14788432e-17, 8.11881986e-17, 6.94044053e-17, 6.08154281e-17, 5.46879058e-17, 5.05529185e-17, 4.80946252e-17, 4.73138189e-17, 4.81364892e-17, 5.04385788e-17, 5.41654570e-17, 5.97278115e-17, 6.76346493e-17, 7.85746491e-17, 9.38146054e-17, 1.14493714e-16, 1.44418212e-16, 1.85607057e-16, 2.38659496e-16, 3.06714343e-16, 3.93733465e-16, 5.05350071e-16, 6.47601628e-16, 8.24828807e-16, 1.04121290e-15, 1.30018957e-15, 1.61052951e-15, 1.97929233e-15, 2.42270778e-15, 2.96779201e-15, 3.66440375e-15, 4.58399364e-15, 5.81581408e-15, 7.54716363e-15, 1.02829271e-14, 1.40967745e-14, 1.92225495e-14, 2.63787437e-14, 3.74097690e-14, 5.48240301e-14, 8.41402192e-14, 1.36448597e-13, 2.28263625e-13, 3.83611565e-13, 6.63103262e-13, 1.17899816e-12, 2.14402831e-12, 4.02005682e-12, 7.59522943e-12, 1.44579856e-11, 2.78670584e-11, 5.39732160e-11, 1.05395387e-10, 2.05813742e-10, 4.00095277e-10, 7.73170427e-10, 1.47844130e-09, 2.78375142e-09, 5.16530072e-09, 9.02369074e-09, 1.66687828e-08, 2.56358258e-08, 3.76940505e-08, 4.42446464e-08, 5.31173381e-08, 5.76185453e-08, 6.49620247e-08, 6.80854032e-08, 6.43556120e-08, 6.01231632e-08, 5.80527379e-08, 5.73937382e-08, 5.73475219e-08, 5.78145345e-08, 5.89567885e-08, 5.85323913e-08, 5.61465690e-08, 5.32114637e-08, 5.05463284e-08, 4.80681135e-08, 4.58184976e-08, 4.36044929e-08, 4.15950931e-08, 3.99997150e-08, 3.86663966e-08, 3.75240203e-08, 3.65423322e-08, 3.56993700e-08, 3.44968708e-08, 3.35969638e-08, 3.28977653e-08])
- HCN(pressure)float648.375e-26 1.067e-25 ... 1.03e-06
- units :
- v/v
array([8.37548651e-26, 1.06703057e-25, 1.11862001e-25, 1.21357233e-25, 1.36515924e-25, 1.59653359e-25, 1.94506177e-25, 2.44467312e-25, 3.20290282e-25, 4.33706599e-25, 6.04556615e-25, 8.76858444e-25, 1.32418442e-24, 2.08047385e-24, 3.34388618e-24, 5.52563891e-24, 9.49823761e-24, 1.66166205e-23, 2.93583388e-23, 5.23161826e-23, 9.33826015e-23, 1.61042691e-22, 2.75759566e-22, 4.74220533e-22, 8.15848503e-22, 1.40024547e-21, 2.34336134e-21, 3.86224878e-21, 6.18649064e-21, 1.00705060e-20, 1.66321208e-20, 2.77230030e-20, 4.75083410e-20, 8.46534291e-20, 1.54659283e-19, 2.82849718e-19, 5.22639190e-19, 9.73539739e-19, 1.88885287e-18, 3.86575780e-18, 8.24561335e-18, 1.85508949e-17, 4.27820314e-17, 1.00372011e-16, 2.39044019e-16, 5.95096663e-16, 1.53788947e-15, 3.96781270e-15, 1.02914499e-14, 2.68512953e-14, 7.07545638e-14, 1.86929902e-13, 4.94369246e-13, 1.29760713e-12, 3.36519201e-12, 8.53243204e-12, 2.13878005e-11, 5.26079147e-11, 1.27117290e-10, 2.93752216e-10, 6.94560735e-10, 1.46585187e-09, 2.91679325e-09, 4.88038360e-09, 7.90634797e-09, 1.15456342e-08, 1.71082430e-08, 2.39843201e-08, 3.05584068e-08, 3.69219746e-08, 4.54570296e-08, 5.55794449e-08, 7.06340092e-08, 8.80059469e-08, 1.01130292e-07, 1.10294689e-07, 1.28189148e-07, 1.52184349e-07, 1.82047577e-07, 2.17942118e-07, 2.60751851e-07, 3.03820615e-07, 3.45665111e-07, 3.99596996e-07, 4.66435236e-07, 5.47267991e-07, 6.43787762e-07, 7.58334475e-07, 8.31303588e-07, 9.20955821e-07, 1.02979153e-06])
- C2H2(pressure)float644.5e-38 4.5e-38 ... 2.95e-07
- units :
- v/v
array([4.50000000e-38, 4.50000000e-38, 4.50000000e-38, 4.50000000e-38, 4.50000000e-38, 4.50000000e-38, 4.50000000e-38, 4.50000000e-38, 4.50000000e-38, 4.50000000e-38, 4.50000000e-38, 4.50000000e-38, 4.50000000e-38, 4.50000000e-38, 4.50000000e-38, 4.50000000e-38, 4.50000000e-38, 4.50000000e-38, 4.50000000e-38, 4.50000000e-38, 4.50000000e-38, 4.50000000e-38, 4.50000000e-38, 4.50000000e-38, 4.50000000e-38, 4.50000000e-38, 4.50000000e-38, 4.50000000e-38, 4.50000000e-38, 4.50000000e-38, 1.97579265e-37, 1.02614924e-35, 4.92247683e-34, 2.29361928e-32, 2.08809114e-31, 6.40637631e-31, 1.94239199e-30, 5.95744317e-30, 1.96154254e-29, 6.94617402e-29, 2.71128794e-28, 1.19497689e-27, 5.61447039e-27, 2.66149766e-26, 1.33170968e-25, 7.08868087e-25, 3.96397454e-24, 2.34323860e-23, 1.40835463e-22, 8.55025546e-22, 5.30697828e-21, 3.31303595e-20, 2.09306666e-19, 1.31446744e-18, 8.13982316e-18, 4.94122075e-17, 2.92815657e-16, 1.67734907e-15, 9.33089642e-15, 4.79854479e-14, 2.57815767e-13, 1.12087799e-12, 4.33639881e-12, 1.19152437e-11, 3.07338148e-11, 6.45581548e-11, 1.39705517e-10, 2.70691601e-10, 4.35741817e-10, 6.30388094e-10, 9.45264581e-10, 1.39415641e-09, 2.21462311e-09, 3.35819459e-09, 4.32693696e-09, 5.02503937e-09, 6.59506904e-09, 8.99403621e-09, 1.24138468e-08, 1.71105171e-08, 2.34986243e-08, 3.07041777e-08, 3.84907864e-08, 4.97823883e-08, 6.56772545e-08, 8.76310802e-08, 1.17665021e-07, 1.58456667e-07, 1.90408157e-07, 2.34496896e-07, 2.95024141e-07])
- C2H4(pressure)float644.5e-38 4.5e-38 ... 7.684e-09
- units :
- v/v
array([4.50000000e-38, 4.50000000e-38, 4.50000000e-38, 4.50000000e-38, 4.50000000e-38, 4.50000000e-38, 4.50000000e-38, 4.50000000e-38, 4.50000000e-38, 4.50000000e-38, 4.50000000e-38, 4.50000000e-38, 4.50000000e-38, 4.50000000e-38, 4.50000000e-38, 4.50000000e-38, 4.50000000e-38, 4.50000000e-38, 4.50000000e-38, 4.50000000e-38, 4.50000000e-38, 4.50000000e-38, 4.50000000e-38, 4.50000000e-38, 4.50000000e-38, 4.50000000e-38, 4.50000000e-38, 4.50000000e-38, 4.50000000e-38, 4.50000000e-38, 1.88818054e-36, 5.18323278e-32, 1.67918814e-27, 7.44788979e-23, 8.21156928e-21, 1.51252939e-20, 2.76843339e-20, 5.09888414e-20, 9.75477059e-20, 1.94022958e-19, 4.06602075e-19, 9.09275926e-19, 2.10461977e-18, 4.89489195e-18, 1.17224380e-17, 2.90180150e-17, 7.37519993e-17, 1.93214660e-16, 5.10703449e-16, 1.35768103e-15, 3.65334668e-15, 9.86339366e-15, 2.68086942e-14, 7.26500050e-14, 1.95474105e-13, 5.20213171e-13, 1.36545697e-12, 3.51443180e-12, 8.87933002e-12, 2.10156215e-11, 5.24480229e-11, 1.08495661e-10, 2.12468993e-10, 3.18984543e-10, 4.89373188e-10, 6.47442458e-10, 9.28228264e-10, 1.23383181e-09, 1.42005049e-09, 1.51494388e-09, 1.70257990e-09, 1.88350023e-09, 2.20247700e-09, 2.30702395e-09, 2.01624628e-09, 1.63870187e-09, 1.54988583e-09, 1.56628558e-09, 1.64613436e-09, 1.77148243e-09, 1.94701302e-09, 2.08414607e-09, 2.18625537e-09, 2.41754811e-09, 2.78117911e-09, 3.29339926e-09, 3.99401890e-09, 4.93664557e-09, 5.51802762e-09, 6.40386517e-09, 7.68367418e-09])
- C2H6(pressure)float644.5e-38 4.5e-38 ... 4.955e-11
- units :
- v/v
array([4.50000000e-38, 4.50000000e-38, 4.50000000e-38, 4.50000000e-38, 4.50000000e-38, 4.50000000e-38, 4.50000000e-38, 4.50000000e-38, 4.50000000e-38, 4.50000000e-38, 4.50000000e-38, 4.50000000e-38, 4.50000000e-38, 4.50000000e-38, 4.50000000e-38, 4.50000000e-38, 4.50000000e-38, 4.50000000e-38, 4.50000000e-38, 4.50000000e-38, 4.50000000e-38, 4.50000000e-38, 4.50000000e-38, 4.50000000e-38, 4.50000000e-38, 4.50000000e-38, 4.50000000e-38, 4.50000000e-38, 4.50000000e-38, 4.50000000e-38, 6.85367938e-36, 6.93297887e-30, 9.89659904e-24, 2.45158809e-17, 1.13852555e-14, 1.47097940e-14, 1.89657775e-14, 2.45095889e-14, 3.20775921e-14, 4.25215090e-14, 5.73708543e-14, 7.90791286e-14, 1.10300580e-13, 1.54112518e-13, 2.17612723e-13, 3.10654471e-13, 4.47365402e-13, 6.51604666e-13, 9.51889718e-13, 1.39425503e-12, 2.05167966e-12, 3.02406089e-12, 4.47038454e-12, 6.60584062e-12, 9.74497294e-12, 1.43238847e-11, 2.09335237e-11, 3.03377017e-11, 4.35302146e-11, 5.91450444e-11, 8.53411385e-11, 1.04035557e-10, 1.25912855e-10, 1.23955243e-10, 1.33077900e-10, 1.28794214e-10, 1.40414856e-10, 1.45820521e-10, 1.35337453e-10, 1.19259620e-10, 1.12112694e-10, 1.03841088e-10, 1.00245176e-10, 8.26571422e-11, 5.61094791e-11, 3.62918401e-11, 2.79480752e-11, 2.35004572e-11, 2.09898940e-11, 1.95747448e-11, 1.90054210e-11, 1.82999620e-11, 1.75521111e-11, 1.80453099e-11, 1.95958636e-11, 2.22027096e-11, 2.61139722e-11, 3.16909659e-11, 3.51366688e-11, 4.08480782e-11, 4.95498071e-11])
- SiO(pressure)float644.5e-38 4.5e-38 ... 3.882e-05
- units :
- v/v
array([4.50000000e-38, 4.50000000e-38, 4.50000000e-38, 4.50000000e-38, 4.50000000e-38, 4.50000000e-38, 4.50000000e-38, 4.50000000e-38, 4.50000000e-38, 4.50000000e-38, 4.50000000e-38, 4.50000000e-38, 4.50000000e-38, 4.50000000e-38, 4.50000000e-38, 4.50000000e-38, 4.50000000e-38, 4.50000000e-38, 4.50000000e-38, 4.50000000e-38, 4.50000000e-38, 4.50000000e-38, 4.50000000e-38, 4.50000000e-38, 4.50000000e-38, 4.50000000e-38, 4.50000000e-38, 4.50000000e-38, 4.50000000e-38, 4.50000000e-38, 4.50004677e-38, 4.50017544e-38, 4.50030731e-38, 4.50044433e-38, 4.50052029e-38, 4.50055312e-38, 4.50058569e-38, 4.50058925e-38, 4.50056100e-38, 4.50052056e-38, 1.12547141e-37, 2.72557492e-37, 2.73033381e-36, 3.62915983e-35, 5.13895209e-34, 7.95750537e-33, 1.32277605e-31, 2.33192174e-30, 4.21000978e-29, 7.62408549e-28, 1.41211381e-26, 2.61482702e-25, 4.87374172e-24, 8.91403204e-23, 1.58690300e-21, 2.71143522e-20, 4.41518364e-19, 6.77084011e-18, 9.98731137e-17, 1.41849090e-15, 1.92676174e-14, 2.51434642e-13, 2.71334905e-12, 2.38173812e-11, 1.58620830e-10, 8.85676543e-10, 4.21347860e-09, 1.82423074e-08, 7.04244925e-08, 2.43172952e-07, 7.94697150e-07, 2.54782486e-06, 8.73104811e-06, 2.18206570e-05, 4.33331936e-05, 5.71887420e-05, 6.13071506e-05, 6.13495878e-05, 6.13511768e-05, 6.12924403e-05, 6.11526429e-05, 6.08455723e-05, 6.02745964e-05, 5.94250502e-05, 5.82021141e-05, 5.65095863e-05, 5.42965280e-05, 5.15338116e-05, 4.76757004e-05, 4.33815895e-05, 3.88150398e-05])
- MgH(pressure)float644.5e-38 4.5e-38 ... 4.017e-06
- units :
- v/v
array([4.50000000e-38, 4.50000000e-38, 4.50000000e-38, 4.50000000e-38, 4.50000000e-38, 4.50000000e-38, 4.50000000e-38, 4.50000000e-38, 4.50000000e-38, 4.50000000e-38, 4.50000000e-38, 4.50000000e-38, 4.50000000e-38, 4.50000000e-38, 4.50000000e-38, 4.50000000e-38, 4.50000000e-38, 4.50000000e-38, 4.50000000e-38, 4.50000000e-38, 4.50000000e-38, 4.50000000e-38, 4.50000000e-38, 4.50000000e-38, 4.50000000e-38, 4.50000000e-38, 4.50000000e-38, 4.50000000e-38, 4.50000000e-38, 4.50000000e-38, 4.50004677e-38, 4.50017544e-38, 4.50030731e-38, 4.50044433e-38, 4.50052029e-38, 4.50055312e-38, 4.50058569e-38, 4.50058925e-38, 4.50056100e-38, 4.50052056e-38, 4.50054559e-38, 4.50058217e-38, 4.50057355e-38, 4.50055847e-38, 4.50056159e-38, 4.50057548e-38, 4.65723211e-38, 5.07857638e-37, 5.26278297e-36, 8.66067451e-35, 2.19173749e-33, 5.55399427e-32, 1.42011079e-30, 3.56612056e-29, 8.71060416e-28, 2.04358078e-26, 4.64083065e-25, 1.00554723e-23, 2.09152704e-22, 4.17233451e-21, 7.94002493e-20, 1.44183845e-18, 2.12938635e-17, 2.52134794e-16, 2.21553925e-15, 1.61833387e-14, 9.94194660e-14, 5.49818652e-13, 2.67829906e-12, 1.15543892e-11, 4.69356395e-11, 1.86779946e-10, 7.98202493e-10, 5.19734837e-09, 3.18377006e-08, 1.06130507e-07, 1.74577689e-07, 2.33812128e-07, 3.09458623e-07, 4.05037844e-07, 5.24289458e-07, 6.71248568e-07, 8.50439084e-07, 1.06625600e-06, 1.32371955e-06, 1.62855853e-06, 1.98538894e-06, 2.40025654e-06, 2.87169450e-06, 3.40885634e-06, 4.01659515e-06])
- OCS(pressure)float641.013e-22 1.074e-22 ... 4.346e-10
- units :
- v/v
array([1.01291719e-22, 1.07411748e-22, 9.50086014e-23, 8.65317624e-23, 8.12648708e-23, 7.88505420e-23, 7.91578423e-23, 8.26785724e-23, 8.98600666e-23, 1.01111259e-22, 1.17112530e-22, 1.39958889e-22, 1.72600737e-22, 2.19399466e-22, 2.88051727e-22, 3.88121516e-22, 5.43619216e-22, 7.78282824e-22, 1.11488838e-21, 1.59608476e-21, 2.28194524e-21, 3.26104733e-21, 4.65041237e-21, 6.58394574e-21, 9.22359295e-21, 1.27571832e-20, 1.74705929e-20, 2.37018492e-20, 3.20029539e-20, 4.32514447e-20, 5.89941086e-20, 8.16962956e-20, 1.15031372e-19, 1.66338039e-19, 2.54667206e-19, 3.92653247e-19, 6.01611635e-19, 9.28405449e-19, 1.48898405e-18, 2.48216966e-18, 4.36642663e-18, 8.19541179e-18, 1.59454895e-17, 3.11873524e-17, 6.29866039e-17, 1.31495283e-16, 2.81882427e-16, 6.25365287e-16, 1.39984400e-15, 3.15832737e-15, 7.22551518e-15, 1.66135585e-14, 3.85353977e-14, 8.93194859e-14, 2.05778977e-13, 4.71065910e-13, 1.06890413e-12, 2.39757723e-12, 5.37227463e-12, 1.12779719e-11, 2.47057008e-11, 4.62398952e-11, 8.11912999e-11, 1.15772837e-10, 1.62441946e-10, 2.04233491e-10, 2.61910911e-10, 3.13441771e-10, 3.38162096e-10, 3.50889734e-10, 3.72627044e-10, 3.93387173e-10, 4.26615894e-10, 4.53651231e-10, 4.66120272e-10, 4.67013957e-10, 4.79445557e-10, 4.93151470e-10, 5.06167935e-10, 5.16517335e-10, 5.24454573e-10, 5.22944690e-10, 5.13458798e-10, 5.07219920e-10, 5.02411256e-10, 4.98103414e-10, 4.94348082e-10, 4.91321594e-10, 4.68171146e-10, 4.49547033e-10, 4.34608312e-10])
- Li(pressure)float644.5e-38 4.5e-38 ... 2.194e-09
- units :
- v/v
array([4.50000000e-38, 4.50000000e-38, 4.50000000e-38, 4.50000000e-38, 4.50000000e-38, 4.50000000e-38, 4.50000000e-38, 4.50000000e-38, 4.50000000e-38, 4.50000000e-38, 4.50000000e-38, 4.50000000e-38, 4.50000000e-38, 4.50000000e-38, 4.50000000e-38, 4.50000000e-38, 4.50000000e-38, 4.50000000e-38, 4.50000000e-38, 4.50000000e-38, 4.50000000e-38, 4.50000000e-38, 4.50000000e-38, 4.50000000e-38, 4.50000000e-38, 4.50000000e-38, 4.50000000e-38, 4.50000000e-38, 4.50000000e-38, 4.50000000e-38, 1.49890573e-37, 3.75188575e-36, 8.90501729e-35, 2.08928850e-33, 1.99442074e-32, 1.01836929e-31, 5.12123218e-31, 2.60350556e-30, 1.44540094e-29, 8.81107468e-29, 6.04148376e-28, 4.83703172e-27, 4.17285978e-26, 3.63456508e-25, 3.35136826e-24, 3.34276474e-23, 3.54865427e-22, 3.97363310e-21, 4.54384859e-20, 5.20940672e-19, 6.09308877e-18, 6.83452525e-17, 7.67572145e-16, 6.31433638e-15, 4.26758621e-14, 1.84057430e-13, 6.70267443e-13, 1.97711891e-12, 5.23080374e-12, 1.02643915e-11, 2.04006239e-11, 3.54928231e-11, 5.74955676e-11, 8.83862411e-11, 1.28450897e-10, 1.87437883e-10, 2.64826143e-10, 3.77586326e-10, 5.23679397e-10, 6.96606044e-10, 9.13623448e-10, 1.14783960e-09, 1.44732151e-09, 1.74696346e-09, 2.01013304e-09, 2.18241084e-09, 2.31690095e-09, 2.41559770e-09, 2.48142971e-09, 2.52195585e-09, 2.54677607e-09, 2.54841012e-09, 2.52716579e-09, 2.50289512e-09, 2.47590043e-09, 2.44636612e-09, 2.41494114e-09, 2.38206257e-09, 2.31960371e-09, 2.25686701e-09, 2.19424230e-09])
- LiOH(pressure)float644.5e-38 4.5e-38 ... 5.348e-11
- units :
- v/v
array([4.50000000e-38, 4.50000000e-38, 4.50000000e-38, 4.50000000e-38, 4.50000000e-38, 4.50000000e-38, 4.50000000e-38, 4.50000000e-38, 4.50000000e-38, 4.50000000e-38, 4.50000000e-38, 4.50000000e-38, 4.50000000e-38, 4.50000000e-38, 4.50000000e-38, 4.50000000e-38, 4.50000000e-38, 4.50000000e-38, 4.50000000e-38, 4.50000000e-38, 4.50000000e-38, 4.50000000e-38, 4.50000000e-38, 4.50000000e-38, 4.50000000e-38, 4.50000000e-38, 4.50000000e-38, 4.50000000e-38, 4.50000000e-38, 4.50000000e-38, 1.02187809e-36, 5.18050020e-33, 2.97174003e-29, 2.18190982e-25, 1.63835722e-23, 5.28764001e-23, 1.68812080e-22, 5.43257157e-22, 1.86171003e-21, 6.81904504e-21, 2.71693604e-20, 1.20886685e-19, 5.67480056e-19, 2.68248419e-18, 1.32144658e-17, 6.88442599e-17, 3.74956587e-16, 2.12309869e-15, 1.22027982e-14, 7.02772459e-14, 4.10627385e-13, 2.30122826e-12, 1.28926303e-11, 5.31631083e-11, 1.81361627e-10, 3.98807984e-10, 7.47073496e-10, 1.14720216e-09, 1.59374634e-09, 1.64264657e-09, 1.75590376e-09, 1.60985924e-09, 1.44708759e-09, 1.27021334e-09, 1.15142402e-09, 1.09313008e-09, 1.05459598e-09, 1.04368318e-09, 1.02964449e-09, 1.01722895e-09, 1.01009558e-09, 9.76803257e-10, 9.23016959e-10, 8.10544268e-10, 7.01467658e-10, 5.93230110e-10, 4.77637056e-10, 3.79337041e-10, 3.02119599e-10, 2.42595592e-10, 1.97425080e-10, 1.63789197e-10, 1.38315451e-10, 1.18023742e-10, 1.01776144e-10, 8.87013711e-11, 7.82212966e-11, 6.97812177e-11, 6.34930502e-11, 5.81222303e-11, 5.34836001e-11])
- LiH(pressure)float644.5e-38 4.5e-38 ... 1.204e-09
- units :
- v/v
array([4.50000000e-38, 4.50000000e-38, 4.50000000e-38, 4.50000000e-38, 4.50000000e-38, 4.50000000e-38, 4.50000000e-38, 4.50000000e-38, 4.50000000e-38, 4.50000000e-38, 4.50000000e-38, 4.50000000e-38, 4.50000000e-38, 4.50000000e-38, 4.50000000e-38, 4.50000000e-38, 4.50000000e-38, 4.50000000e-38, 4.50000000e-38, 4.50000000e-38, 4.50000000e-38, 4.50000000e-38, 4.50000000e-38, 4.50000000e-38, 4.50000000e-38, 4.50000000e-38, 4.50000000e-38, 4.50000000e-38, 4.50000000e-38, 4.50000000e-38, 1.31903135e-37, 2.39357440e-36, 4.27146215e-35, 7.79423121e-34, 6.82283510e-33, 3.50743544e-32, 1.77692182e-31, 9.09454280e-31, 5.06374048e-30, 3.08367924e-29, 2.10105726e-28, 1.66065602e-27, 1.40939105e-26, 1.20708064e-25, 1.09126103e-24, 1.06360707e-23, 1.10039680e-22, 1.19727977e-21, 1.32912900e-20, 1.47862810e-19, 1.67623676e-18, 1.82183552e-17, 1.98137157e-16, 1.57910028e-15, 1.03484432e-14, 4.33263520e-14, 1.53357583e-13, 4.40466995e-13, 1.13616954e-12, 2.17772802e-12, 4.23231984e-12, 7.22216426e-12, 1.15578306e-11, 1.77125099e-11, 2.59136915e-11, 3.83244040e-11, 5.51982267e-11, 8.05583479e-11, 1.14909123e-10, 1.57781096e-10, 2.14012611e-10, 2.78112682e-10, 3.61963100e-10, 4.47836004e-10, 5.27159573e-10, 5.87647904e-10, 6.42756630e-10, 6.92685700e-10, 7.37777064e-10, 7.79741338e-10, 8.21124959e-10, 8.59072031e-10, 8.92761388e-10, 9.28775616e-10, 9.67070164e-10, 1.00759060e-09, 1.05071429e-09, 1.09665500e-09, 1.13106104e-09, 1.16682351e-09, 1.20400141e-09])
- LiCl(pressure)float644.5e-38 4.5e-38 ... 4.816e-13
- units :
- v/v
array([4.50000000e-38, 4.50000000e-38, 4.50000000e-38, 4.50000000e-38, 4.50000000e-38, 4.50000000e-38, 4.50000000e-38, 4.50000000e-38, 4.50000000e-38, 4.50000000e-38, 4.50000000e-38, 4.50000000e-38, 4.50000000e-38, 4.50000000e-38, 4.50000000e-38, 4.50000000e-38, 4.50000000e-38, 4.50000000e-38, 4.50000000e-38, 4.50000000e-38, 4.50000000e-38, 4.50000000e-38, 4.50000000e-38, 4.50000000e-38, 4.50000000e-38, 4.50000000e-38, 4.50000000e-38, 4.50000000e-38, 4.50000000e-38, 4.50000000e-38, 5.53435350e-36, 2.94705911e-30, 2.01384112e-24, 2.14261539e-18, 8.94124095e-16, 1.76341876e-15, 3.48314203e-15, 6.77265033e-15, 1.35148074e-14, 2.84146020e-14, 5.99252151e-14, 1.34841154e-13, 2.99442537e-13, 6.69088931e-13, 1.44628706e-12, 3.25016781e-12, 7.54646258e-12, 1.69305103e-11, 3.83903990e-11, 8.63094937e-11, 1.93589899e-10, 4.15568727e-10, 8.84400005e-10, 1.41489352e-09, 1.89158618e-09, 1.84324098e-09, 1.65369857e-09, 1.33107233e-09, 1.04310800e-09, 1.20584639e-09, 1.33131313e-09, 1.54300994e-09, 1.82785302e-09, 1.99268508e-09, 2.13107885e-09, 2.13563209e-09, 2.13175174e-09, 2.00459826e-09, 1.85177138e-09, 1.59169746e-09, 1.34349858e-09, 1.05239315e-09, 7.96232421e-10, 5.14323882e-10, 3.04121950e-10, 1.74660886e-10, 1.01454768e-10, 5.98839895e-11, 3.60396284e-11, 2.21654730e-11, 1.39987603e-11, 9.04132519e-12, 5.95563691e-12, 4.03638183e-12, 2.80764113e-12, 1.99904108e-12, 1.45725759e-12, 1.08546963e-12, 8.13886031e-13, 6.20980743e-13, 4.81628634e-13])
- LiF(pressure)float644.5e-38 4.5e-38 ... 2.427e-14
- units :
- v/v
array([4.50000000e-38, 4.50000000e-38, 4.50000000e-38, 4.50000000e-38, 4.50000000e-38, 4.50000000e-38, 4.50000000e-38, 4.50000000e-38, 4.50000000e-38, 4.50000000e-38, 4.50000000e-38, 4.50000000e-38, 4.50000000e-38, 4.50000000e-38, 4.50000000e-38, 4.50000000e-38, 4.50000000e-38, 4.50000000e-38, 4.50000000e-38, 4.50000000e-38, 4.50000000e-38, 4.50000000e-38, 4.50000000e-38, 4.50000000e-38, 4.50000000e-38, 4.50000000e-38, 4.50000000e-38, 4.50000000e-38, 4.50000000e-38, 4.50000000e-38, 2.50755543e-36, 1.50233965e-31, 1.08309471e-26, 1.10283212e-21, 1.98915853e-19, 4.70457586e-19, 1.10344426e-18, 2.60249754e-18, 6.43875669e-18, 1.67651761e-17, 4.65137159e-17, 1.40441441e-16, 4.41354372e-16, 1.39402006e-15, 4.53614239e-15, 1.54063604e-14, 5.41327185e-14, 1.95457233e-13, 7.13824356e-13, 2.60926303e-12, 9.63641629e-12, 3.43017847e-11, 1.22227390e-10, 3.19654056e-10, 6.98680305e-10, 9.59249916e-10, 1.12474402e-09, 1.08510271e-09, 9.43329166e-10, 6.52204650e-10, 4.59851758e-10, 2.91590861e-10, 1.86092292e-10, 1.22607426e-10, 8.44928609e-11, 6.25016836e-11, 4.75690078e-11, 3.80736952e-11, 3.11522167e-11, 2.53713868e-11, 2.07746097e-11, 1.63038675e-11, 1.25495914e-11, 8.55623785e-12, 5.43657535e-12, 3.38340843e-12, 2.13210617e-12, 1.36414624e-12, 8.88331847e-13, 5.89748875e-13, 4.00738242e-13, 2.77557093e-13, 1.95453798e-13, 1.41130118e-13, 1.04251965e-13, 7.86026175e-14, 6.04942035e-14, 4.74424417e-14, 3.73806412e-14, 2.99061927e-14, 2.42720499e-14])
- pressurePandasIndex
PandasIndex(Index([ 9.999999999999999e-05, 0.00011869515722309572, 0.00014088540348215412, 0.00016722415116753576, 0.00019848696914329385, 0.00023559442009199016, 0.00027963916733702855, 0.0003319181493280418, 0.00039397076919690886, 0.0004676242239113106, 0.0005550473077848112, 0.0006588142746377424, 0.000781980639089466, 0.0009281731490214104, 0.0011016965785335226, 0.001307660486011831, 0.0015521296698160403, 0.0018423027518944656, 0.002186724147886555, 0.0025955356653693494, 0.003080775138791673, 0.0036567308946788214, 0.004340362484664547, 0.005151800075224848, 0.0061149371991076965, 0.007258134322574446, 0.008615053945643212, 0.010225651825635732, 0.01213735351152469, 0.014406450833227162, 0.017099759466766982, 0.020296586383850262, 0.024091065119232508, 0.028594927619991398, 0.03394079429637921, 0.04028607915285485, 0.04781762498950186, 0.05675720516163953, 0.06736805390204312, 0.07996261749716994, 0.09491175455796852, 0.11265565629577945, 0.13371680836098576, 0.15871537591777782, 0.1883874649828338, 0.22360679774997894, 0.26541044015086745, 0.3150293392235825, 0.37392456949031083, 0.4438303556523082, 0.5268051384453322, 0.6252921873370347, 0.7421915448634276, 0.8809454210721684, 1.0456395525912738, 1.241123510935086, 1.4731535026372076, 1.7485618660927769, 2.075458256101916, 2.463468440179892, 2.92401773821287, 3.4706674516029694, 4.1195141883709585, 4.88966384271464, 5.803794185791013, 6.888822633729537, 8.17669885592547, 9.705345562699813, 11.519775174691278, 13.673415255346974, 16.229681735100865, 19.263846252286, 22.865252596366325, 27.13994751871501, 32.21380337760442, 38.23622456658652, 45.38454686548587, 53.869259256977955, 63.9402019699871, 75.89392325704105, 90.08241153272058, 106.92345999911892, 126.91296895432794, 150.63954803683833, 178.8018483824859, 212.2291350553931, 251.90570552721528, 298.99987322947635, 354.8983696265842, 421.247177810478, 499.99999999999994], dtype='float64', name='pressure'))
- wavelengthPandasIndex
PandasIndex(Index([ 227.53128555176335, 138.79250520471896, 97.75171065493646, 73.69196757553428, 53.87931034482759, 42.211903756859435, 35.75259206292456, 29.700029700029702, 24.83854942871336, 21.39495079161318, ... 0.46478552471961815, 0.4447755551354898, 0.42581005039461944, 0.4083232613595531, 0.3870968990635158, 0.3620692620394818, 0.33703737407441114, 0.312000312000312, 0.2869566028355617, 0.26786796520127265], dtype='float64', name='wavelength', length=196))
- pressure_layerPandasIndex
PandasIndex(Index([0.00010894730709067374, 0.00012931517744160413, 0.00015349085317763729, 0.0001821862095022677, 0.00021624620780751522, 0.00025667377634611263, 0.0003046593423844756, 0.0003616158854381028, 0.0004292205437644458, 0.0005094639992550354, 0.0006047090949108359, 0.000717760410946776, 0.0008519468482584137, 0.0010112196509975335, 0.0012002687546223626, 0.0014246608853987063, 0.001691003477819942, 0.002007139236646397, 0.0023823770726238854, 0.0028277662119979073, 0.0033564215512324946, 0.0039839098373052806, 0.0047287080450158815, 0.005612747448652777, 0.006662059408713707, 0.007907541889468775, 0.00938586927818712, 0.011140572296498449, 0.013223319802881479, 0.015695440230142934, 0.01862972745802518, 0.022112584296537223, 0.02624656669686443, 0.031153403606507913, 0.03697758139109016, 0.04389059836945267, 0.052096014740779344, 0.061835446603535196, 0.07339568056566953, 0.08711711844238258, 0.10340380070341651, 0.12273530381957679, 0.14568086183689094, 0.1729161280012587, 0.2052430699951834, 0.24361358462029145, 0.28915752728187444, 0.34321598162963685, 0.40738074901008875, 0.4835412205341497, 0.5739400119514853, 0.6812389995520702, 0.8085977015833742, 0.9597663131967247, 1.1391961344231616, 1.3521706428330007, 1.6049610704351762, 1.9050110659225121, 2.2611558798140976, 2.6838825266046187, 3.1856385846365467, 3.7811987265939497, 4.488099773448383, 5.327157082423961, 6.323077474504406, 7.505186749701155, 8.908293212444732, 10.57371263440564, 12.55048483572611, 14.89681770802591, 17.681801199779322, 20.987441733953286, 24.911076963221486, 29.56824196746212, 35.09607129138454, 41.65733699843869, 49.44524164525162, 58.689107310171, 69.66112819463883, 82.68438563400882, 98.14236152723765, 116.49023031721376, 138.26826202456317, 164.1177309996972, 194.7997988410679, 231.21792750468, 274.4444825796634, 325.752310088043, 386.65221661686934, 458.9374564199777], dtype='float64', name='pressure_layer'))
- climate_params :
- {'cvs_locs': array([ 0, 73, 89, 0, 83, 89]), 'converged': 1}
- planet_params :
- {"effective_temp": 999.9689549563479, "gravity": {"value": 100000.0, "unit": "cm / s2"}, "p_reference": {"value": 1.0, "unit": "bar"}}
Benchmark with Sonora Bobcat¶
[11]:
pressure_bobcat,temp_bobcat = np.loadtxt(jdi.os.path.join(
sonora_profile_db,f"t{teff}g{grav}nc_m0.0.dat"),
usecols=[1,2],unpack=True, skiprows = 1)
plt.figure(figsize=(10,10))
plt.ylabel("Pressure [Bars]", fontsize=25)
plt.xlabel('Temperature [K]', fontsize=25)
plt.ylim(500,1e-4)
plt.xlim(200,3000)
plt.semilogy(out['temperature'],out['pressure'],color="r",linewidth=3,label="Our Run")
plt.semilogy(temp_bobcat,pressure_bobcat,color="k",linestyle="--",linewidth=3,label="Sonora Bobcat")
plt.minorticks_on()
plt.tick_params(axis='both',which='major',length =30, width=2,direction='in',labelsize=23)
plt.tick_params(axis='both',which='minor',length =10, width=2,direction='in',labelsize=23)
plt.legend(fontsize=15)
plt.title(r"T$_{\rm eff}$= 1000 K, log(g)=5.0",fontsize=25)
[11]:
Text(0.5, 1.0, 'T$_{\\rm eff}$= 1000 K, log(g)=5.0')
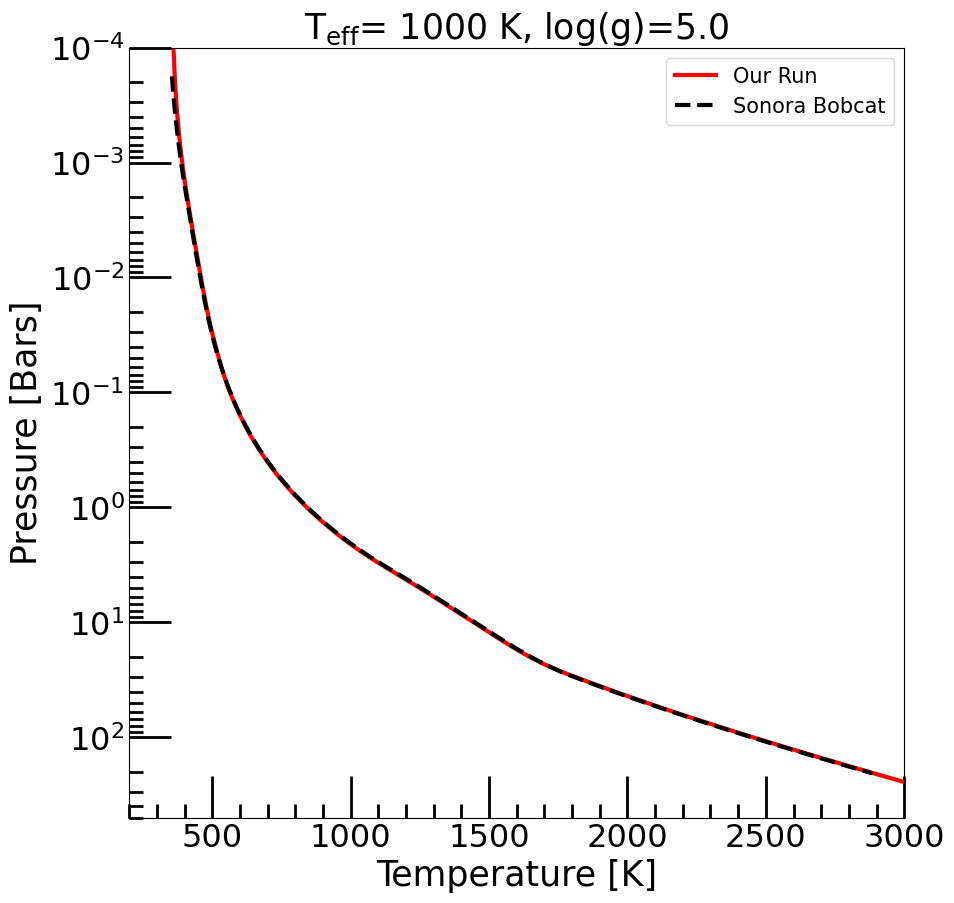
Climate Plots and Animations¶
Animate Convergence¶
We can also try to see how our initial guess of an isothermal atmosphere was changed by the code to reach the converged solution
[12]:
ani = jpi.animate_convergence(out, cl_run, opacity_ck,
molecules=['H2O','CH4','CO','NH3'])
[13]:
ani
[13]: