Custom Particle Distribution and Cloud Pressure Height¶
Following the custom haze layer tutorial, this tutorial you will learn:
How to generate your own Mie coefficients for a given radius grid
How to compute the optics of an aerosol for your custom radius grid with an arbitrary fsed, and pressure height
How to inject this aerosol as a custom layer into an atmospheric model for PICASO
You need to have downloaded PICASO, Virga. This is particularly useful for retrievals with PICASO.
First, let’s import all the necessary packages:
[1]:
#standards
import numpy as np
import pandas as pd
import warnings
warnings.filterwarnings('ignore')
#main programs
#radiative transfer and atmosphere code
from picaso import justdoit as pdi
#cloud code
from virga import justdoit as vdi
#plotting tools
from picaso import justplotit as ppi
from virga import justplotit as vpi
ppi.output_notebook()
import matplotlib.pyplot as plt
Okay, now let’s grab those Mieff coefficients we just calculated.
[2]:
mieff_dir = '/data/virga'
qext, qscat, cos_qscat, nwave, radius, wave_in = vdi.get_mie('SiO2',directory=mieff_dir)
Pick the Cloud Distribution Function¶
Gaussian particle distribution¶
[3]:
sigma = 1 #width of the distribution
mu = -7 # mean particle size
logradius = np.log10(radius)
dist = (1/(sigma * np.sqrt(2 * np.pi)) *
np.exp( - (logradius - mu)**2 / (2 * sigma**2)))
plt.plot(logradius+4, dist)
[3]:
[<matplotlib.lines.Line2D at 0x7fab3c8a2ed0>]
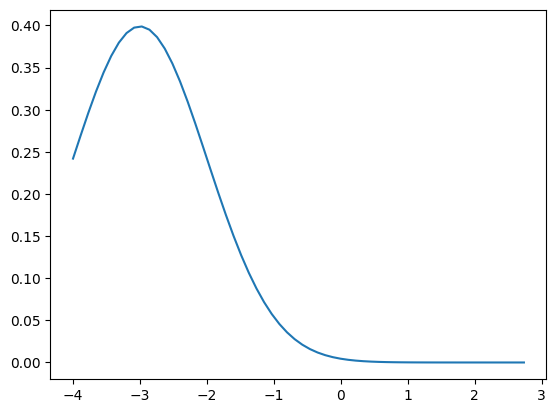
Pick an approximate particle density (if using a fitting code this will be a free parameter)
[4]:
ndz = 5e6 #that's particles/cm^2 but over the entire region of atmosphere where we're sticking aerosol
opd,w0,g0,wavenumber_grid=vdi.calc_optics_user_r_dist(wave_in, ndz ,radius, pdi.u.cm,dist,
qext, qscat, cos_qscat)
Pick \(f_{sed}\) and cloud base pressure¶
[5]:
nlayer = 30
pressure = np.logspace(-6,2,nlayer)
z = np.linspace(100,0,nlayer)#just toy model here
scale_h = 10 #toy model (could grab from picaso calc)
base_pressure = 1e-1 # let's start by setting the haze layer base at 0.1 bars
fsed=1 #this is more just an exponential scaling to control the cloud drop off, and is not connected to particle size
opd_h = pressure*0+10
opd_h[base_pressure<pressure]=0
opd_h[base_pressure>=pressure]=opd_h[base_pressure>=pressure]*np.exp(
-fsed*z[base_pressure>=pressure]/scale_h)
opd_h = opd_h/np.max(opd_h)
plt.loglog(opd_h, pressure)
plt.ylim(1e2,1e-6)
plt.xlim(1e-2,10)
[5]:
(0.01, 10)
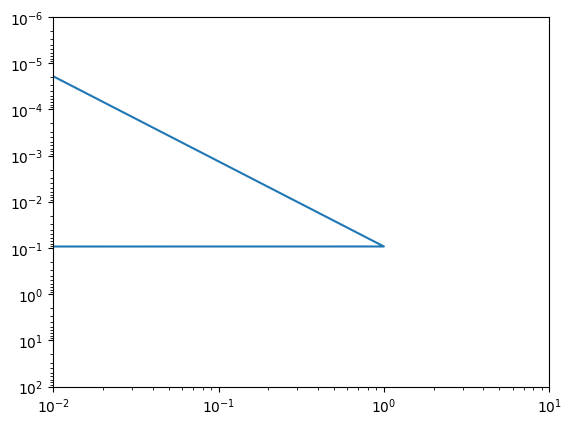
[6]:
#here's where we shove all the variables into a dataframe PICASO can read
df_cld = vdi.picaso_format_slab(base_pressure,opd, w0, g0, wavenumber_grid, pressure,
p_decay=opd_h)
Let’s checkout our optical parameters
[7]:
nwno = len(wavenumber_grid)
ppi.show(ppi.plot_cld_input(nwno, nlayer,df=df_cld,pressure=pressure, wavelength=1e4/wavenumber_grid))
Now run PICASO
¶
[8]:
opa = pdi.opannection(wave_range=[0.3,14])
case1 = pdi.inputs()
case1.phase_angle(0)
#here we are going to have to specify gravity through R and M since we need it in the Flux calc
case1.gravity(mass=1, mass_unit=pdi.u.Unit('M_jup'),
radius=1.2, radius_unit=pdi.u.Unit('R_jup'))
#here we are going to have to specify R as well
case1.star(opa, 4000,0.0122,4.437,radius=0.7, radius_unit = pdi.u.Unit('R_sun') )
#atmo -- make sure your pressure grid matches the one you computed your haze on!
case1.atmosphere( df = pdi.pd.DataFrame({'pressure':np.logspace(-6,2,31),
'temperature':np.logspace(-9,3,31)*0+600, #just an isothermal one for simplicity
"H2":np.logspace(-9,3,31)*0+0.837,
"He":np.logspace(-9,3,31)*0+0.163,
"H2O":np.logspace(-9,3,31)*0+1e-4}))
And compute and plot our clear transmission spectrum, without our haze:
[9]:
df= case1.spectrum(opa, full_output=True,calculation='transmission')
wno, rprs2 = df['wavenumber'] , df['transit_depth']
wno, rprs2 = pdi.mean_regrid(wno, rprs2, R=300)
full_output = df['full_output']
ppi.show(ppi.spectrum(wno,rprs2*1e6,plot_width=500))
Now, let’s add in that hazy information. We need to make sure the pressure grids match (by reducing the layers by one because of PICASO’s idiosyncrasies).
[10]:
case1.clouds(df=df_cld.astype(float))
hazy= case1.spectrum(opa, full_output=True,calculation='transmission')
hazyx,hazyy =hazy['wavenumber'] , hazy['transit_depth']
hazyx,hazyy = pdi.mean_regrid(hazyx,hazyy, R=300)
Let’s compare our hazy spectrum to our clear spectrum!
[11]:
ppi.show(ppi.spectrum([wno,hazyx],[(rprs2*1e6),(hazyy*1e6)],
legend=['clear','hazy'],plot_width=900,plot_height=300))
Very cloud! Verification we can see the SiO2 feature at 9um
[12]:
ppi.heatmap_taus(hazy)
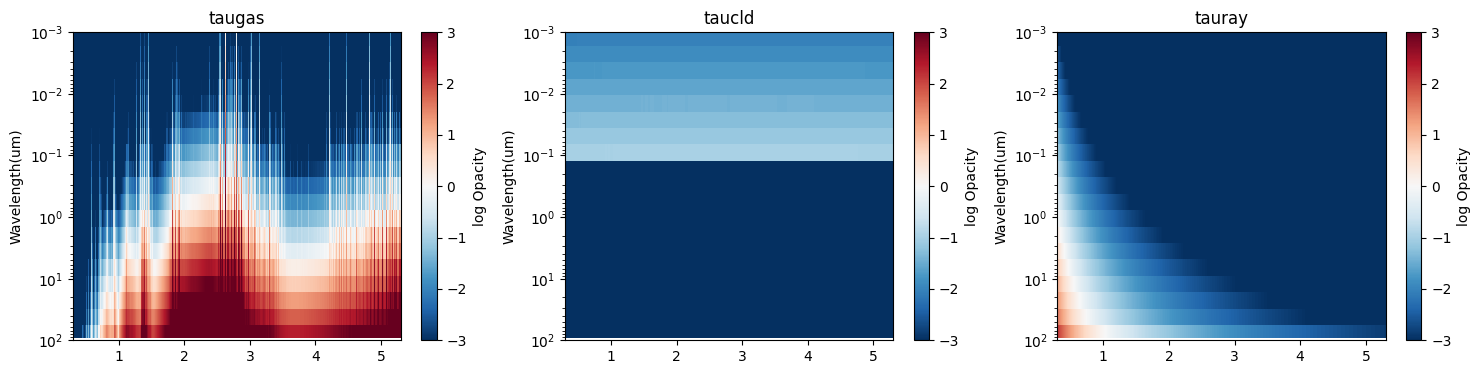